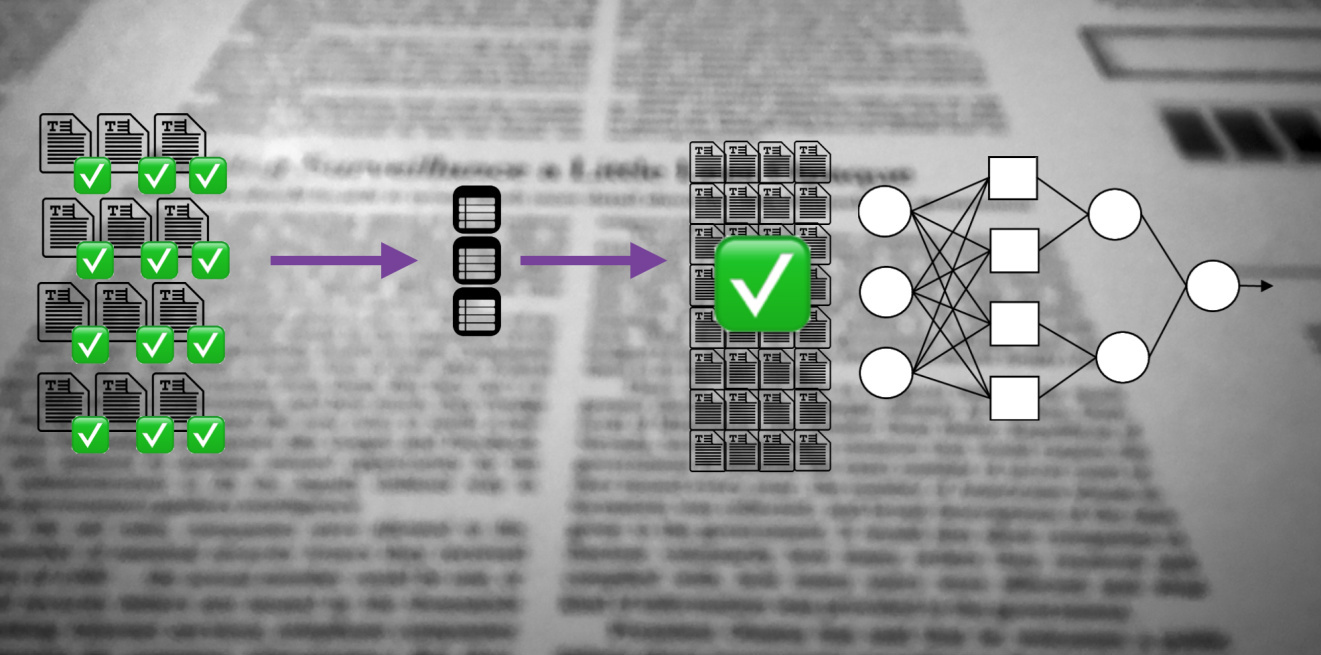
The editorial staff of the Oxford Dictionary chose the word “post-truth” (post-truth) as the honorary “
word of 2016 ”. This term describes circumstances in which objective facts are less important for the formation of public opinion than appeal to emotions and personal convictions.
A particularly high frequency of using this word was observed in English-language publications after the presidential elections in the United States in connection with the spread of a large amount of fake news, after which the truth itself did not matter much.
According
to some analysts, the spread of false, defamatory news was one of the reasons for the defeat of Hilary Clinton in the elections. One of the main sources of false news
has become an algorithmic Facebook feed.
Against this background, news coming from developers in the field of artificial intelligence remained almost unnoticed. We have already got used to the fact that neural networks draw pictures, create a photo of a person according to his verbal description, generate music. They are doing more and more, and each time they get better. But the most interesting thing is that the machines have learned how to create fakes.
Fake influence
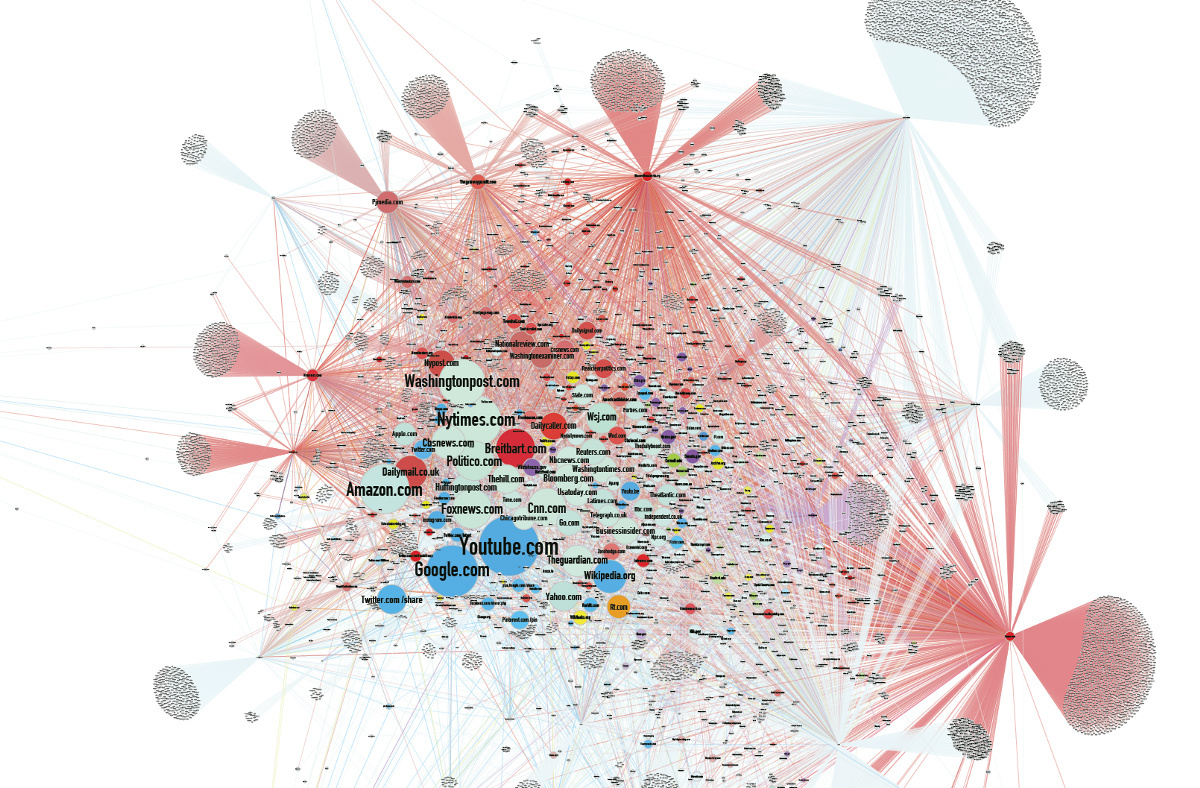
During the pre-election race in the United States, the number of fake news reposts on social networks exceeded the number of reposts of true messages, because the fakes met more expectations or were more exciting. After the election, Facebook hired independent fact-checkers who began tagging unverified messages to alert users.
The combination of increased political polarization of the society and a tendency to read mainly the headings, gives a cumulative effect. Fake news is also often disseminated through fake news sites, information from which often gets into the mainstream media that are chasing user traffic. And nothing attracts traffic like a catchy headline.
But in fact, the influence of fakes is not limited to the political sphere alone. There are many examples that demonstrate the impact of false news on society.
An Australian video production company has been producing
fake viral videos for two years, which have gained hundreds of millions of views.
The
thoughtful fake about Stalin, whose face allegedly appeared in the Moscow metro, was believed even on April 1st.
Millionaire Ilya Medkov in 1992 began to pay major news agencies in Russia and the CIS (including RIA Novosti, Interfax, ITAR-TASS). ITAR-TASS launched a
false report in the media about the accident at the Leningrad NPP in January 1993. As a result, the shares of the leading Scandinavian companies fell in price, and until a denial appeared in the media, Medkov’s agents bought the most profitable shares of Swedish, Finnish and Norwegian companies.
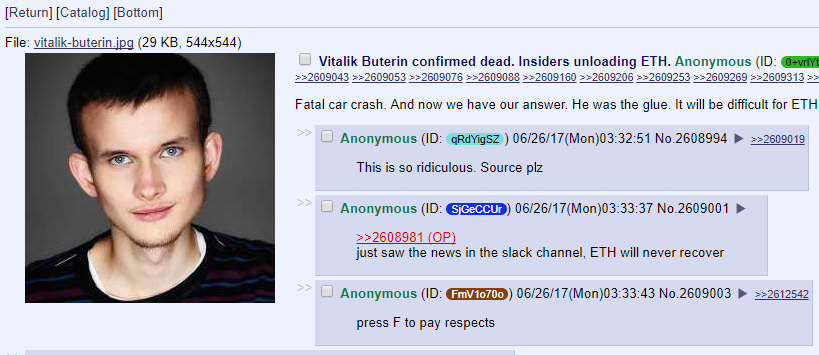
On June 26, 2017, Ethereum’s second-largest cryptocurrency capitalization rate went down sharply - the exchange reacted to rumors about the tragic death of Vitaly Buterin, the founder of Ether, appeared on the network. And the first “urgent news” appeared on the anonymous image board 4chan - and this source, frankly, is not the most reliable.
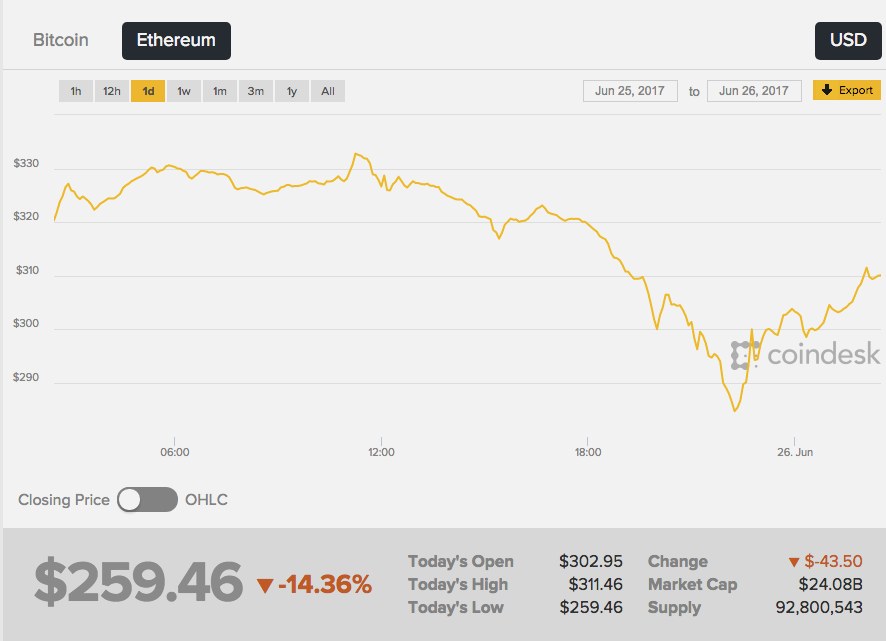
Wikipedia wrote that "Vitalik
was a Russian programmer," and then the yellow press picked up the news. As a result, the rate of broadcast
fell by 13%, from about 289 to 252 dollars. The course began to grow immediately after the refutation of the news from Vitaly himself.
There is no evidence that someone deliberately created a fake for making a profit on the difference in the rates of cryptocurrency. It is indisputable only that invented news without a single confirmed fact has a powerful effect on people.
Imitation of reality
French singer Francoise Ardi in the video will repeat the speech of Kelliann Conway, adviser to US President Donald Trump, who gained fame after speaking of "alternative facts." The interesting thing here is that in fact, Ardi is 73 years old, and in the video she looks like a twenty year old.
The Alternative Face video v1.1 was
created by German action artist Mario Klingeman. He took audio interviews from Conway and Ardi’s old music videos. And then he applied the generative neural network (GAN), which made unique video content from a variety of frames of different clips of the singer, and on top of it laid out an audio track with comments from Conway.
In this case, the fake is easy to recognize, but you can go further - to make changes to the audio file. People will believe the image and sound recording more eagerly than just text. But how to fake the very sound of a human voice?
GAN systems are able to study the statistical characteristics of an audio recording, and then reproduce them in a different context with millisecond accuracy. It is enough to enter the text that the neural network should reproduce, and you will get a believable performance.
Canadian startup Lyrebird has published its algorithms that can mimic the voice of any person based on a one-minute sound file. To demonstrate the capabilities of the company
laid out a conversation between Obama, Trump and Clinton - all the characters, of course, were fakes.
DeepMind, the Baidu Institute of Deep Learning and the Montreal Algorithm Institute (MILA) are already working on highly realistic text-to-speech algorithms.
The result is not yet perfect, you can quickly distinguish the recreated voice from the original one, but the similarity is felt. In addition, in the voice network changes emotions, adds anger or sadness depending on the situation.
Image generation
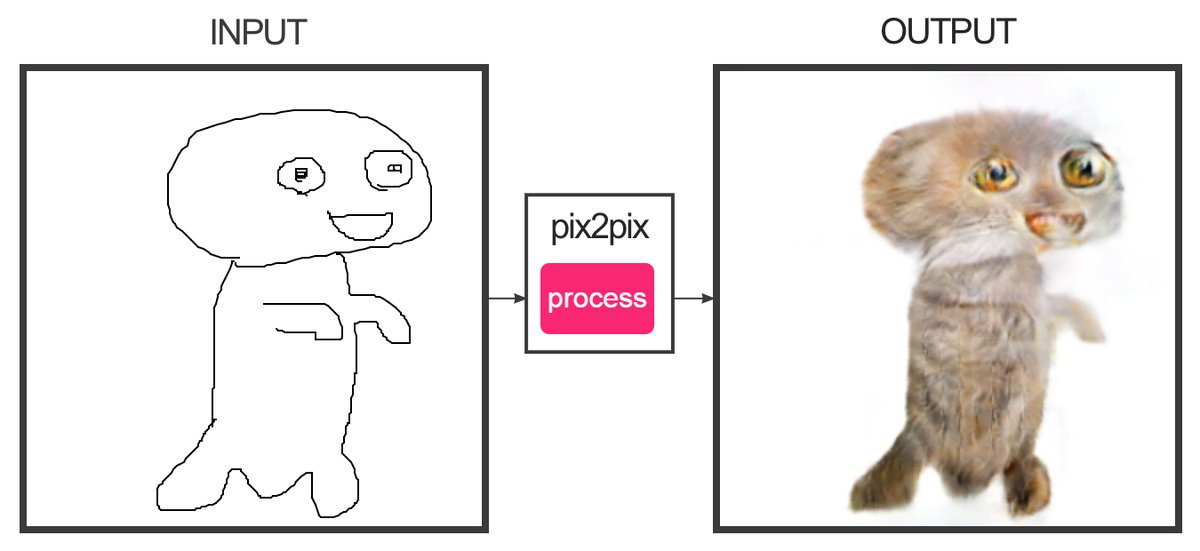
Developer Christopher Hesse created a
service that, with the help of machine learning, can “draw in” sketches consisting of several lines to color photographs. Yes, this is the same site that draws seals. Seals are so-so - it is difficult to confuse them with real ones.
Programmer Alex Jolicier-Martino was able to
make cats that are exactly like real ones. For this, he used DCGAN - Deep Convolutional Generative Adversarial Networks (deep generative convolutional adversarial networks). DCGANs are capable of creating unique photorealistic images using a combination of two deep neural networks that compete with each other.

The first network (generating) receives the values of variables as input, and the output gives the values of functions from these variables, which must “convince” the second network (distinguishing or discriminating) that the results of the first network are indistinguishable from the standard. As samples in the work of Joliker-Martino, a database of ten thousand cat portraits was used.
Using the DCGAN methods allows you to create fake images that can not be objectively recognized as fake ones without another neural network.
Content combinations
The University of Washington has
developed an algorithm that allows you to overlay audio on a video of a person speaking with accurate lip synchronization. The algorithm was trained on 17 hours of Barack Obama video addresses. The neural network was taught to imitate Obama's lip movements in such a way as to correct their movements, imitating the pronunciation of the right words. For now, you can only generate videos with the words that the person actually spoke.
And now look at the work of the algorithm, which
allows you to change the mimicry of another person on the fly to your own mimicry. On the video with Trump (there is still a demonstration on Bush, Putin and Obama) they impose a man’s antics in the studio, and it turns out a grimacing Trump. The algorithm is used in the program Face2Face. The created technology is similar in its way of working to the
Smile Vector bot, which adds a smile to people in the photos.
Thus, it is already possible to create a realistic video in which a famous person speaks out made-up facts. Speech can be cut from previous speeches so as to make up any message. But soon even such tricks will be superfluous - any text will put the network into the mouth of the fake character perfectly accurate.
Effects
From a practical point of view, all these technologies allow us to do a lot of good. For example, you can improve the quality of video conferencing by synthesizing the missing frames if they fall out of the video stream. And even synthesize whole missing words, providing excellent communication in places with any signal level.
It will be possible to completely “digitize” the actor and add his realistic copy to films and games.
But what about the news?
It is likely that in the coming years, generating fakes will reach a new level. There are new ways to fight. For example, if you correlate a photo with known conditions on the ground (wind speed, slope of shadows, level of illumination, etc.), then this data will help to reveal the fake.
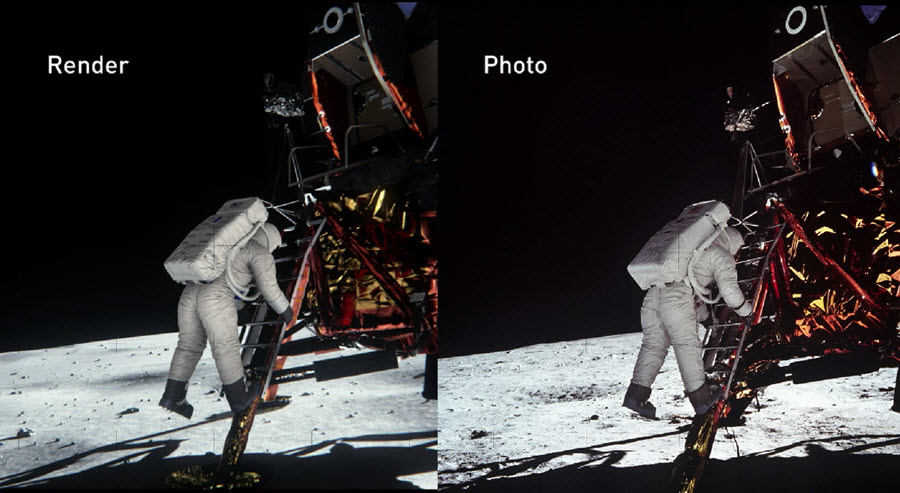
In 2014, NVIDIA engineers reconstructed the moon landing scene as precisely as they could, based on the documentary evidence that has been preserved from that time. In this case, all physical and optical properties of objects were taken into account in order to find out how light is reflected from various materials and behaves in real-time conditions. As a result, they were able to
reliably confirm the authenticity of NASA photos.
NVIDIA confirmed that with proper preparation, it is possible to prove the authenticity (or refute) of even the most complex photo work created a decade ago.
Last year, the United States Defense Advanced Research Projects Agency (DARPA) launched a four-year project to create an open-source
Media Forensic system that can detect photos that were somehow processed or distorted.
Neural networks will be able not only to change the original content, but also to identify the most high-quality fakes. Who will eventually win in this technology race - time will tell.