AntipovSN and MihhaCF
UPD part two here
Part one, in which the Count has not yet become Athos, has not met Milady and everything is fine with him
Introduction from the authors:
Good day! Today we are starting a series of articles devoted to scoring and the use of graph theory in it (T.G.). I hope we have enough fuse, strength and patience, because the topic is quite voluminous and, in our opinion, interesting.
Despite the comic title, we will try to touch upon not comic topics that already affect the lives of many of us, and in the near future they can affect everyone, without exception.
All comic allegories, inserts, etc. are designed to slightly relieve the narrative and not allow it to fall into a tedious lecture. We apologize to everyone who doesn’t get into our humor
Now to the point.
The purpose of this article: in no more than 30 minutes, introduce the reader to the research problem, determine the level of consideration of the problem, describe the basic concept of the study and introduce basic terms.
Terms and Definitions:
- Scoring is a system of point assessment of an object based on numerical statistical methods.
- A graph is a way of modeling the relationships of objects. Imagine that you are playing poker with friends and want to simulate who owes whom now. For example, "D'Artagnan owes Athos 10 louis"

A complete graph might look like this:
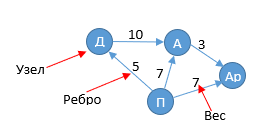
Aramis was always cunning ... on his mind, even Athos owed him. Porthos, until he met Madame Koknar, could not afford to buy a dressing and managed to owe a beggar to D'artanyan, although, frankly, they mutilated something all the way together ...
Graphs consist of nodes and edges. A node can be directly connected to several other nodes. These nodes are called neighbors.
- A weighted graph is a graph with a weight assigned to each edge. A graph without weights is called unweighted.
- A directed or directed graph is a graph whose edges are assigned a direction
- A directed acyclic graph is a case of a directed graph in which there are no directed cycles, that is, paths starting and ending at the same vertex.
- Data Mining is a collective name used to denote a set of methods for detecting previously unknown, nontrivial, practically useful and accessible interpretations of knowledge in data necessary for making decisions in various fields of human activity.
- The breadth-first search algorithm (BFS, Breadth-First Search) answers two questions: does the path from node A to node B exist and what is the shortest path from node A to node B. Bypass is performed by levels: first level nodes are checked, their child nodes are added to the queue, and so on until the end
- Depth-first search algorithm - Depth-first search strategy - Depth-first search strategy is to go deeper into the graph as far as possible. The search algorithm is described recursively: we sort through all the edges coming from the vertex in question. If the edge leads to a vertex that was not previously considered, then we run the algorithm from this unexamined vertex, and after that we return and continue to sort through the edges. The return occurs if there are no edges in the vertex under consideration that lead to the unexamined vertex. If, after the completion of the algorithm, not all vertices were considered, then it is necessary to run the algorithm from one of the unexamined vertices
- Dijkstra's Algorithm - Finds the shortest paths from one of the vertices of the graph to all the others. The algorithm only works for acyclic graphs with weighted edges, without negative weight.
Well, sort of, with the most basic concepts figured out, you can get closer to the point.
Scoring can be used to evaluate almost anything, which can be expressed in statistical indicators. This is an assessment of the creditworthiness of an individual / legal entity (scoring of the applicant), and an assessment of the likelihood of fraud (scoring from fraud), and an assessment of the insured (insurance scoring), an assessment of the supplier / customer (scoring of the counterparty), assessment of consumer behavior (behavioral scoring), social assessment ("Chinese" scoring), etc.
Graph theory, in turn, is also a universal tool that can be used in any field of activity in which it is necessary to process large, multi-level volumes of data.
These two tools are created for each other, like D'artanyan and Constance ( you just need to monitor Constance normally and not let any Miladya go ).
We will not write anything about the importance and topicality of scoring, for it is enough to take a closer look around and it will immediately become clear that we have been explicitly or not explicitly scoring for a long time, then it will only be more fun.
In the series of articles, we will try to clearly demonstrate how scoring works using graph theory in the banking sector. That is, we will determine the creditworthiness of legal entities (maybe we’ll even hook physicists) based on the data they provide and the relationships they have with other organizations - the so-called “borrower scoring” .
As follows from the official definition, the scoring of the borrower is designed to eliminate the subjectivity of the decision of the credit inspector, reduce the level of internal fraud and increase the speed of decision-making on the loan. Let's see if this is so, expand the candy, so to speak, and see what it is made of.
The banking sector was not chosen by chance - banks have extensive sources of information and are scoring using automation, more and more actively.
A little closer to the point. Remember how D'artagnan fought with Mr. de Jussac? A step there, a step here, then they ran around the tree and only then started stabbing each other. We won’t pull like that, but it also makes no sense to stab right away - it will not be clear.
So! In a combat system, a scoring ball will be calculated based on two groups of indicators:
- Indicators obtained directly from the borrower and the state. organs:
- tax reporting;
- passport details of the owners, gene. directors, ch. accountant;
- Statements of Unified State Register of Legal Entities, EGRIP;
- title documents;
- debt data;
- court data;
- and so forth
- Indicators obtained using graph analysis and data mining:
- interaction with state. bodies - in a row / subcontract / supply;
- interaction with companies from the top 100;
- the presence in the environment of the borrower of bankrupt companies, debtors, companies with a low scoring score;
- participation in charity organizations
- and so forth
Based on the listed indicators, a model will be built: the vertices of the graph will be all the organizations with which the borrower interacted in one way or another, the edges of the graph will have weight. The weight of the connection will be set in the range from 1 to 5, characterizing the degree of influence of the nodes on each other.
For example:
- The borrower, who, in this case, is the supplier, is bound by contracts with the Customer for 1 million rubles. The annual turnover of the borrower is 5 million. The annual turnover of the Customer is 100 million rubles. It is clearly seen that the Supplier depends on the Customer more than the Customer from the supplier. Thus, for the Supplier, the connection will be 5 (for example), and for the Customer 1.
It is clear that the example is purely speculative and in real life we will do a more detailed analysis. This is a matter of the following articles, and now it makes no sense to go so deep.
The degree of interaction and the interactions themselves will be determined, inter alia, using graph search algorithms.
In our test system, we will use the same topic with the musketeers and their connections. The model will be as close as possible to the combat and sufficiently demonstrate our idea. What will we ultimately come to, what will the model look like? Take your time to say: “Canalia!” Or “I do not need academies. Any Gascon from childhood is an academician! ” Everything will not be as primitive as it seems.
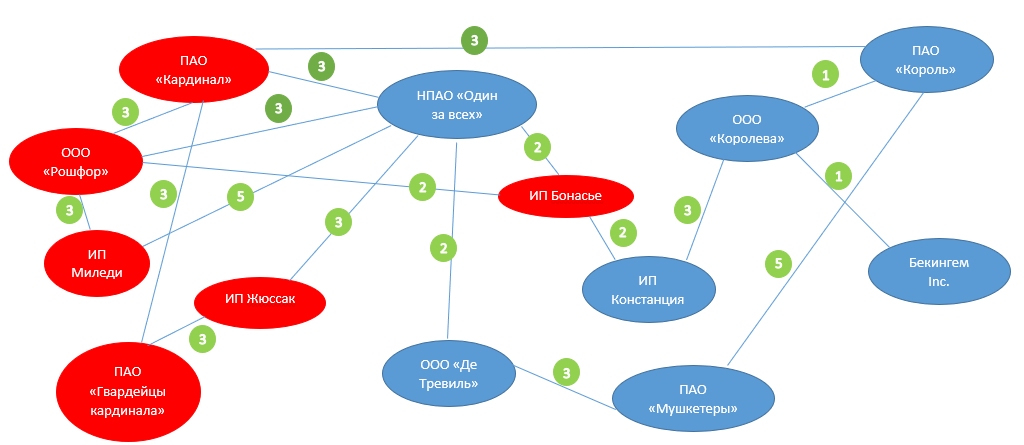
Short description: our musketeers decided to create a non-public joint-stock company (NPAO), which will supply jewelry and provide security services, they need a loan to start the activity. The credit institution is PJSC Korol, which commissioned the evaluation of NPO One for All
Features of the presented graph:
- The graph is non-oriented (bidirectional) and weighted.
- Each rib has a weight - the degree of interaction. In the figure, we did not complicate and make our connection value in each direction from node to node. We limited ourselves to a single aggregated communication assessment. But in the calculation algorithm this will be taken into account.
- Red marked organizations that oppose ours and in every way interfere with it. In real life, it will be competitors, bankrupt companies, malicious defaulters, companies against which litigation is ongoing, etc.
- Probably, you can already guess that you will need to evaluate the relationships by levels and directions, that is, you will need to take into account not only the level of communication, but also the direction. It will be necessary to take into account the mutual influence of the nodes and much more.
We have a lot of work ahead. Well, as part of this article, we are done. The stated objectives of the article, as it seems to us, have been achieved. We hope we managed to interest you, and you read to the end.