About 5 milliseconds go from request to response if the data is stored on the hard drive. SSD responds 30 times faster - in 150 microseconds. RAM requires 300,000 times less time - only 15 nanoseconds. *
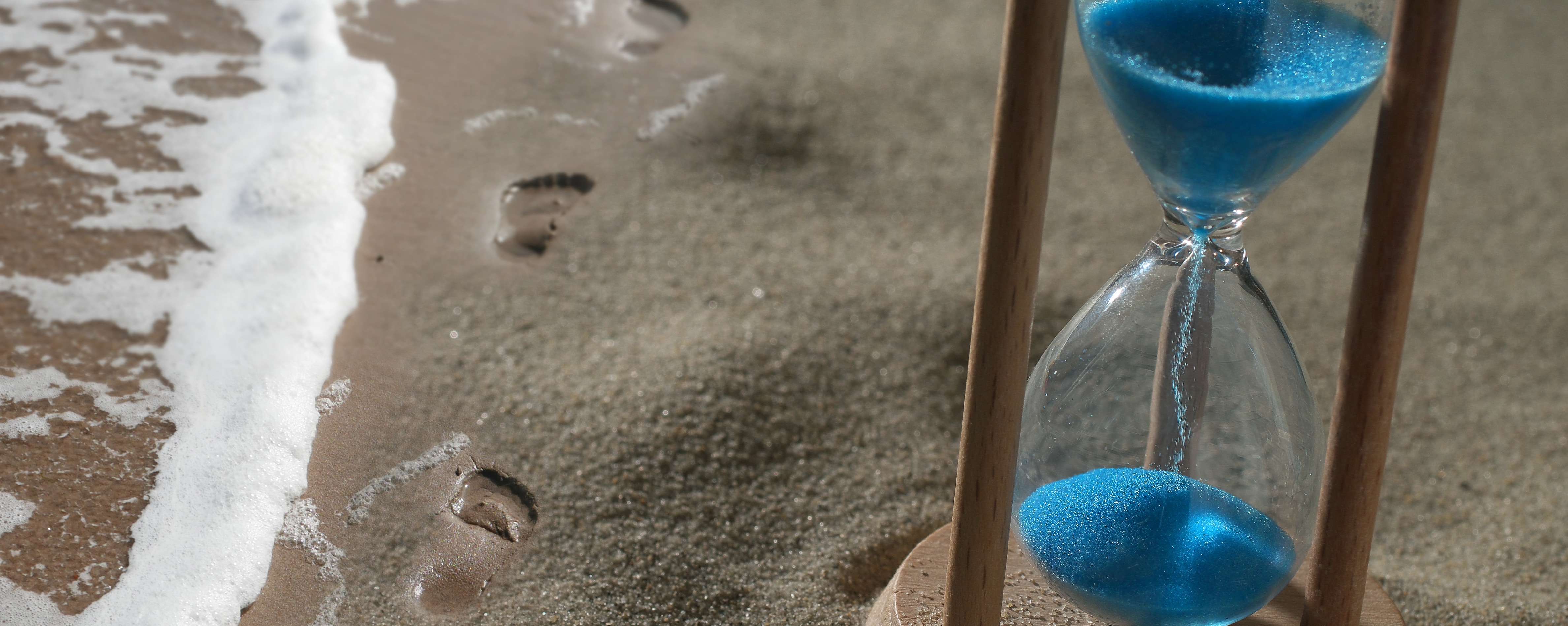
You can talk for a long time about how business intelligence helps finance or logistics. There are many ways to apply information, new ones appear all the time. But the principle of operation of different analytical solutions is the same and it consists in combining data from different sources and looking at them together - that is, in its entirety.
To use information from several sources, you need to connect to them and extract data. But the data was created in different ways, with different frequency and stored in different formats. Therefore, before visualizing the data or transferring it to other systems for further processing, they will have to be combined using some mathematical operations - transform.
The in-memory technology consists in the fact that all data from different sources is loaded at once into the RAM. After this, the transformation can be performed "on the fly", without querying the disk. For example, click to select a dimension and immediately get a graph that will display the values of the indicators in the desired section. Due to the fact that all the data is already in RAM, the analytical application does not need to make requests to the hard drive to obtain new information.
This introduction should help me talk about how and why the technologies underlying modern analytical solutions have changed.
It was expensive at first
“Memory is the new drive,” said Microsoft researcher Jim Gray in the early 2000s. In 2003, he published an article entitled “The Economics of Distributed Computing” **, where he compared the cost of different stages of computer data processing. Jim Gray showed that the calculations should be in the same place as the data - so as not to move it again. He advised moving the calculations as close as possible to data sources. That is, filter the data as early as possible and save as a result.
Over the next few years, in-memory DBMS appeared on the market from several industry leaders, including Oracle, IBM, and SAP, as well as several open source projects - for example, Redis and MemcacheDB.
The first task that the DBMS in-memory solved was not business analytics or even business applications, but e-commerce opportunities that open up in connection with instant information extraction. For example, an in-memory DBMS could allow an online store in real time to offer customers products based on their preferences, or to display ads.
The market for enterprise data analysis solutions has evolved along a different trajectory. Most enterprises are inextricably linked with systems that use transactional DBMSs, which are based on principles developed back in the 80s of the last century. Their task is to constantly save small portions of data going to the stream to disk and immediately confirm their integrity (OLTP work scenario). Among the systems using such DBMS are ERP-solutions, automated banking systems, billing, POS-terminals.
But analytical tasks require a completely different database. Here you need to quickly retrieve previously saved information. Moreover, in large pieces - for every analytical report, absolutely all the data that should be reflected in it will be needed. Even if the report itself consists of one digit.
Moreover, it would be good to upload data as rarely as possible, because their volume can be large, and loading a large data set using analytical queries will run into several obstacles.
Firstly, the hard drive that stores information is a slow drive. Secondly, the structure of data storage in a traditional DBMS will not allow it to quickly perform an analytical query. The data was stored line by line - as they were received, so physically nearby are the values that belong to one row. And in response to an analytical query, the database needs to return the values of one column, but from different rows. Therefore, such requests are slow and create a large load on the storage system. That is, the location of the information on the disk is organized in an inappropriate way.
Thus, traditional DBMSs, in which all the initial information for analysis was initially stored, were poorly suited to play the role of a data source to which the analytical system is connected directly. Therefore, in the last century, for analytical tasks, the standard practice was to use an intermediate data model in which all values are already calculated at some point in time. This data model was called the “analytic cube,” or OLAP cube. To create an OLAP cube, the so-called ETL processes (extract, transform, load) were developed - database queries in the source systems and the rules according to which data transformations should be carried out. Obviously, if there is no information in the OLAP cube, then it cannot appear in the report.
The problem with this approach was the high cost of the solution. First, a data warehouse was required, where the pre-calculated indicators would be placed. Secondly, if we needed a certain indicator in a different context, then in order to get it, all the processes of data transformation on the way from the source system to the OLAP cube had to be re-created by rewriting the analytical queries. Then recalculate the entire OLAP cube, which took several hours.
Suppose an OLAP cube contains sales information for different countries. But the CFO suddenly wanted to see sales by city, and then group them by average bill. To receive such a report, he had to contact the IT department to rebuild the OLAP cube. Or he could force things and attract a connoisseur of MS Excel, who would create such a report manually. To do this, he had to unload data from the source systems into tables using analytical queries and do a number of laborious and undeclared manipulations with them.
In the first case, the CFO had to wait. In the second, he received numbers that are difficult to trust.
In addition, the solution was very expensive. It was necessary to spend money on creating a repository, which must be administered. It was necessary to hire DBMS specialists to do ETL - rebuild OLAP cubes for each of the tasks. In parallel, special analytics usually appeared in the company, which created reports on demand (so-called ad-hoc reports). In fact, they invented different ways to get the desired report using MS Excel and overcame the difficulties associated with the fact that this program is designed for other tasks.
As a result, the reporting path was expensive even for large companies. Managers from small and medium-sized businesses had to be content with the opportunities that are available in MS Excel.
The solution was found elsewhere.
In 1994, the then-Swedish company QlikTech from the small town of Lund released the QuikView program, which was later renamed QlikView. The app was designed to optimize production. It made it possible to know the use of which parts and materials are interconnected and which are not. That is, the program was required to visualize the logical relationships between parts, materials, assemblies and products. To do this, she loaded into the RAM memory data sets from various sources, compared them and instantly showed the connection.
For example, there are several tables with actors, their roles in films, directors, genres, release dates, fees - with anything. All of them are loaded into RAM. Now you can click on any parameter to select it and immediately see all the others that are associated with it. We click on Brad Pitt - we get box office of all the films in which he starred. Choose comedies - get the amount of box office comedies with Brad Pitt. All this happens instantly, in real time.
Although in those years in the market of corporate information systems analytical tasks were solved using intermediate data models - OLAP cubes, the QlikTech approach turned out to be much more convenient. It allowed to abandon the intermediate stage in the form of calculating an OLAP cube and, as a result, save a lot.
The analytical application was directly connected to the sources and periodically loaded all the data necessary for the report into the RAM. The need to change ETL processes each time in order to get the values of indicators in new sections has disappeared - now they are calculated in real time at the time of the request. There is no longer a need to create and administer a data warehouse. The cost of ownership of the analytical solution has plummeted.
With the proliferation of 64-bit servers that made it possible to work with large amounts of RAM, in-memory technology began to rapidly change business intelligence. This is well illustrated by reports by Magic Quadrant research company Gartner. In 2016, six BI platform developers left the quadrant of leaders at once, including industry veterans such as IBM, Oracle and SAP. There are only three players left who have relied on in-memory technology and abandoned OLAP cubes. These are Microsoft, Qlik and Tableau.
Player Position in Gartner's Magic Quadrant for Analytics and Business Intelligence Platforms ***
We can say that Qlik has become a pioneer and leader in market transformation. By 2016, QlikView data analysis platform was used by customers around the world, and annual sales exceeded $ 600M.
From reports to data-driven management
With the spread of analytical solutions based on in-memory technology, a huge number of companies opened up previously inaccessible ways to use corporate data. There was an opportunity not to be limited to management reports, which are standard for each of the industries. A variety of processes began to "measure" - to introduce metrics and use them to describe processes. It has become much easier to use objective information to make more informed decisions. The number of business users working with data has risen sharply.
A huge influence on the interest in the use of data was made by changes in consumer behavior and marketing, which became digital - that is, based on metrics. Many new people have been attracted to Data Science by expectations of how the world will change Big Data.
As a result of all these processes, the “democratization” of corporate data quickly occurred. Previously, data belonged to IT services. Marketing, sales, business intelligence and executives contacted the IT department for reports. Now employees worked with the data on their own. It turned out that direct employee access to data can increase productivity and give a competitive advantage.
However, the first generation of in-memory technology-based analytical solutions gave business users very limited opportunities to use data. They could only work with ready-made panels and dashboards. In-memory technology allowed them to "fall" deep into any indicator and see what it is made of. But it was always about those indicators that are determined in advance. The study was limited to visualizations already on the dashboard. This method of using the data was called “directional analytics” and he did not assume that the business user would independently connect new sources and create indicators and visualizations himself.
The next step in the democratization of data was self-service. The idea of self-service was that business users explore the data, creating visualizations and introducing new indicators on their own.
It is worth noting that by the time in-memory technology began to change business analytics, there were no serious technological obstacles before giving users access to all the data. Perhaps the most conservative customers had a question about the appropriateness of such a function. But the world has already turned in the direction of the desire to "count everything." Now managers who do not have a mathematical education and programming skills, also needed a tool that would allow them to speak the data language.
Direct access to data for business analysts has opened up many new opportunities. They could put forward and test any hypotheses, apply Data Science methods, identify such dependencies, the existence of which is difficult to predict in advance. Now you can combine internal corporate data with external data obtained from third-party sources.
In September 2014, Qlik released the second generation of its platform, called Qlik Sense. Qlik Sense used the same architecture and the same technology. The difference was in the new approach to creating visualizations. Now standard visualizations could be created on the fly simply by dragging and dropping fields with the desired dimensions onto the sheet. This simplified data mining due to a very sharp reduction in the research cycle. Testing a hypothesis began to take only a couple of seconds.
Perhaps the rapid growth in sales of self-service analytic platforms was largely due to the ease of demonstration. If earlier the customer had to make a purchase decision, considering the presentation slides, now he could install the program on his computer, connect to sources and in a couple of hours go all the way from creating a dashboard to opening it in his data.
There is data. Now what?
In-memory technology has had a big impact on how businesses use information today. Combining and exploring data has become easier, and it was a strong business push towards digital transformation. However, it is foolish to say that the digital transformation has become commonplace and now any company can easily implement it.
From the point of view of technology, everything is simple as long as the volume of the studied data is limited to several Excel tables. If it comes to combining billions of records, then most likely the task will continue to be difficult from a technical point of view, and its solution will require expertise in the field of BI and engineering findings. Especially if you still need to manage the quality of the data, which is a common task for most medium and large companies.
From a business point of view, everything is simple as long as you need reporting or dashboards with industry-standard indicators. If we are talking about an analytical system, to which new sources are constantly added, new metrics are introduced, and specialists from different fields are involved in all this, then there is no simplicity either.
However, these are not the difficulties that customers overcame several years ago. The maturity level of analytical platforms today is such that even if there is a lot of initial data, then you no longer need to wait for the calculation of indicators, and you can trust the obtained numbers. At the heart of the transformation is in-memory computing.
The next technology that will change the market for analytical solutions is likely to be cloud platforms. Already, the infrastructure of cloud service providers (CSP), along with a set of services on it, is turning into a data management platform.
Sources:
* IDC, "Market Guide for In-Memory Computing Technologies",
www.academia.edu/20067779/Market_Guide_for_In-Memory_Computing_Technologies** Jim Gray "Distributed Computing Economics",
www.microsoft.com/en-us/research/wp-content/uploads/2016/02/tr-2003-24.doc*** You can see how the situation of BI platform developers in Gartner Magic Quadrant reports has changed from 2010 to 2019 on the interactive visualization:
qap.bitmetric.nl/extensions/magicquadrant/index.html