The topic of artificial intelligence, which originated in the 60s, is now experiencing just a crazy boom. Computers beat chess players and fans of Go, sometimes they are more likely to be diagnosed with a doctor, neural networks (this time not related to the minds of three technical support engineers) are seriously trying to solve complex applied problems, and somewhere there on the horizon is looming universal artificial intelligence, which when - Something will replace his applied relative.

Information security also does not remain outside the borders of hype around AI (or its evolution - here everyone decides for himself). Increasingly, we hear about the necessary approaches, solutions being worked out, and even (sometimes timidly and uncertainly, and sometimes loudly and, unfortunately, not very believably) about the first practical successes in this area. Of course, we will not undertake to speak for all information security, but we will try to figure out what are the real possibilities of using AI in the SOC (Security Operations Center) direction that is relevant to us. Who is interested in the topic or just want to sneak in the comments - welcome to cat.
Typing AI for IS tasks, or not all AIs are equally useful
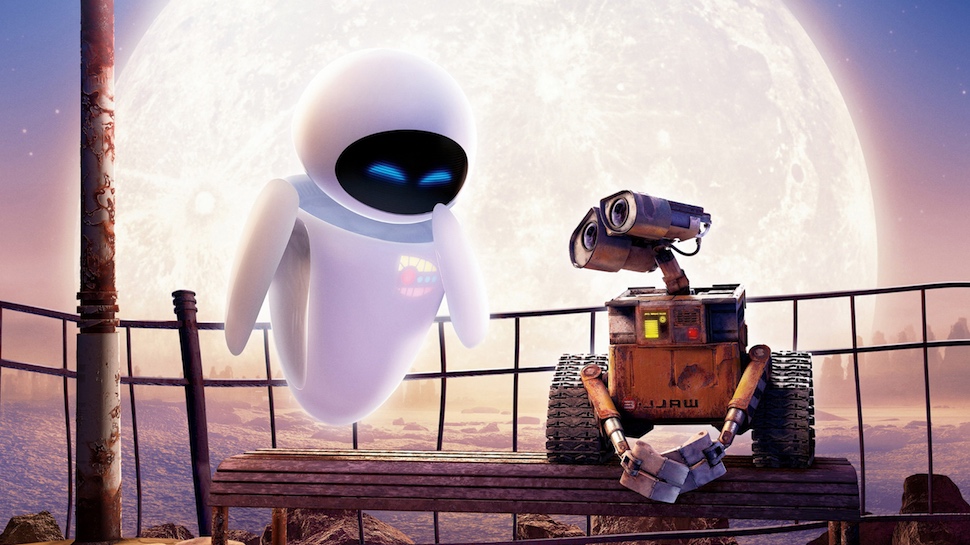
There are many approaches to the classification of artificial intelligence - from the point of view of types of systems, evolutionary waves of development of a direction, types of training, etc. In this post we will consider the classification of types of AI from the point of view of the engineering approach. In this classification, AI is divided into 4 types.
1. Logical approach (computer expert system) - AI is formed primarily as a system of proof of complex facts. The system interprets any emerging goal as a task that must be solved by logical methods. According to sources, the IBM Watson system, notorious for all Russian chess fans, uses similar approaches in its work.
The essence of this approach is that the system for the most part has two main interfaces: for acquiring information (where training is carried out by an expert in the subject area) and for solving a problem (where the knowledge and techniques obtained are used to solve logical and practical problems).
This approach is most often taken into account when speaking about the prospects for the use of AI in information security, so we will put a checkmark on it for more detailed consideration in the future.
2. Structural approach - when one of the main engineering tasks of AI is emulation of the human brain with its ability to structure and analyze information. In fact, the data streams fed into the system and the feedback provided to it (which helps a lot of ordinary people, including SOC analysts), she learns and improves internal decision-making algorithms.
Due to the possibility of detailed feedback, these approaches are often used in relation to arrays of conditionally structured data. This is image processing, personalization of data, tagging of audio / video content or other information. In most known implementations, the system, not being purely expert and not requiring a mode of acquiring knowledge, nevertheless requires substantial operator work to form a stable and meaningful feedback flow. There is a resemblance to the work of the human brain: for AI to “grow”, it must be taught what is good and what is bad, what is hot, what is cold, where is mom, and where is a stranger.
3. The evolutionary approach - the cultivation of AI in the process of sharing knowledge between simpler programs and the formation of a new, more complex code structure. The task of evolution is primarily the creation of a “perfect look” and adaptation to a new aggressive environment, survival, in order to avoid the sad fate of dinosaurs.
In my opinion, the chances of such an approach leading us to artificial intelligence, capable of solving information security problems or participating in the activities of SOC, are small. Our cyber environment, of course, is quite aggressive, attacks occur every day and massively, but the option of creating conditions for the IS environment to support and stimulate the evolutionary approach seems unlikely. People with an alternative opinion on the issue are very welcome to comment.
4. Simulation approach - the creation of a simulator of actions in the studied area through long-term observations of the simulated subject. To simplify, the task is to read all the input parameters and output data (analysis results, actions, etc.) so that after some time the machine can produce exactly the same results as the object under study, and potentially broadcast the same thoughts if the object was a person.
Despite the attractiveness of attaching Big Brother to the SOC analyst, the IB approach also seems to be of little use. First of all, due to the difficulty of collecting and separating new knowledge in the field of information security from all others (a person is weak and is happy to be distracted by external contexts even in the work process), and due to the imperfection of observation tools (shunts for reading information have not yet been specially developed and glory to god).
If you look integrally at all the described approaches, especially in the context of their application for SOC analytics tasks, a common feature is noticeable: the baby AI for proper development must be fed - with methods, correct answers and the most structured data that will explain to him how in the future he should Build and make your own decisions, or teach him how to use external information interfaces. Moreover, in our case, these interfaces should also be structured and automated: if the SOC analyst can receive information about the threat or asset by phone, then this number will not work with the AI.
In the general case, part of the information security processes (fraud detection, protection of web applications, analysis of user rights and credentials) really supports the principle of large numbers and a “logical” structure. In the case of incident detection, everything is much more entertaining.
AIIs this, or the capabilities of artificial intelligence in the context of SOC processes

Now let's try to “land” the logical and structural approaches to artificial intelligence on key SOC processes. Since in both cases imitation of human logical thinking is implied, it’s worth starting to ask a question: what would I, an SOC analyst, do in order to solve this problem or get an answer to it from somewhere - automated? Let's go through the key processes of SOC:
1. The process of inventorying or collecting information about assets. A sufficiently large task, including for AI, which should receive a context about objects of observation and with its help be trained.
In theory, this is a fertile field for AI. When a new system appears, you can reliably “compare” it with its neighbors (by analyzing network traffic, software structure and communication with other IPs) and from this make an assumption about its purpose, class, and key stored information. And if you add the creation context there (“the system was written by Vasya, and Vasya in our company is an IT document management specialist, and the last ten systems he created were document management” or “at the same time 4 more systems were created that clearly indicate the purpose” etc.), then conducting an inventory and accounting of assets seems feasible for AI task.
Emerging nuances or external problemsA. In practice, we observe a considerable level of entropy among customers, even within the framework of a separate business system. Here and features of the work of a particular engineer, and a slightly modified interaction configuration for this system, and additional software. And for the processes of monitoring and managing incidents, it is important for us to understand whether the system is productive or test, whether the combat data is uploaded to it or not, and a dozen other minor issues that are usually easy to clarify by phone and quite difficult to isolate from information flows.
B. To approach the problem, at some stage it is necessary to create a conditionally sterile environment in which we still know who is who and what tasks are being solved. The processes of even the basic creation of an asset model for most customers ... well, in general, we will not talk about sad things, you yourself know everything.
Nevertheless, we note the promise of using AI in this task as “someday” and move on.
2. The vulnerability management process. Of course, we are not talking about basic instrumental scanning and identifying vulnerabilities and configuration defects (here even ML in Python is not needed, not like AI on Powerpoint - everything works on basic algorithms). The task is to put the identified vulnerabilities on the actual asset map, prioritize them depending on the criticality and value of the assets under threat, form a plan ... And here's the stop. To really figure out which of the assets is worth is a task that even a live security guard often cannot figure out. The process of risk analysis and asset valuation usually dies at the stage of assessing the value of information or aligning this assessment with the business. In Russia, no more than a dozen companies took this road.
But, perhaps, in the facilitated mode (when the cost of a resource or its criticality is estimated by a relative 10- or 100-point scale), the problem can definitely be solved. Moreover, automation issues return us first of all to the previous item - inventory. After that, the problem is solved by classical statistical analysis, without complex AI tricks.
3. Threat analysis. When we finally inventoried all the assets, understood all the configuration errors and possible vulnerabilities, it would be nice to put the well-known attack vectors and attacker's techniques on this picture. This will allow us to assess the likelihood that the attacker will be able to achieve the goal. It’s ideal to add statistics on testing employees for the ability to determine phishing and the capabilities of the IS or SOC service to detect incidents (the volume of the controlled part of the infrastructure, the number and types of monitored cyber attack scenarios, etc.).
Does the task look solvable? Provided that we managed in the previous two stages, there are two key nuances.
1. Techniques and methods for attacking an attacker also require an input machine interpretation. And it’s not about IoCs that are easily decomposed and applied, but, first of all, about TTP (Tactics, Techniques and Procedures) attackers, which entail a much more complex chain of conditions (“under what kind of input am I vulnerable?”). Even a basic analysis of the well-known techniques of the Miter matrix confirms that the event tree will be very branched, and for each decision to make a decision about the relevance of the threat, each fork requires algorithmization.
2. In this case, the artificial neural brain is completely opposed by the natural - the attacker. And the likelihood of non-standard, not described or not directly falling into TTP actions, there are extremely many.
4. Detection / detection of new threats / anomalies, etc. When people discuss the use of AI in SOCs, they usually mean these processes. Indeed, unlimited computing power, the lack of a broken focus of attention, Data Lake - what is not the basis for AI to detect new anomalies and threats, have not been fixed before?
The key problem is that for this you need to at least cluster activities by functional / business structures and information assets (return to point 1), otherwise the entire huge data stream in our Data Lake will not have the required context for detecting anomalies. The use of AI in this area is limited to a clearly defined range of applied tasks; in the general case, it will produce too many false positives.
5. Incident analysis is the “unicorn” of all automation lovers regarding SOC issues: all data is automatically collected, false alarms are filtered, informed decisions are made
, and the door to Narnia lurks in every wardrobe .
Unfortunately, this approach is incompatible with the level of entropy mess that we see in the information flows of organizations. The volume of detected anomalies can change daily - not because of the growing volume of cyberattacks, but because of updating and changing the principles of application software, changing user functionality, the mood of the CIO, the moon phase, etc. In order to at least somehow work with incidents received from Data Lake (as well as from UBA, NTA, etc.), SOC analyst would need to not only go on for a long time and persistently google the probable causes of such a strange behavior of the system, but also have a Full View of information systems: see every process that is launched and updated, every adjustment of the registry or network stream flags, understand all the actions performed in the system. Even if you forget what a huge stream of events this will provoke, and how many orders of magnitude the cost of a license for any product used in the work of SOC will increase, there are still enormous operational costs for maintaining such an infrastructure. In one of the Russian companies we knew, we managed to “comb” all network flows, enable port security, configure NAC — in short, do everything in Feng Shui. This allowed a very high quality analysis and investigation of all network attacks, but at the same time increased the number of network administrators supporting this state by about 60%. Whether an elegant IB solution is worth such additional costs - each company decides and evaluates for itself.
Therefore, a telephone receiver, communication with administrators and users, hypotheses that require verification at stands, etc., remain the necessary link in the analysis of incidents. And these AI functions are poorly delegated.
In general, for the time being we say that we strictly do not believe in the use of AI in incident analysis, but we really hope that in the near future we will be able to give AI at least an asset inventory and vulnerability management.
6. Response and response to incidents. Oddly enough, in this part, the use of AI seems to be a fairly viable model. Indeed, after a qualitative analysis, classification and filtering of false positives, as a rule, it is already clear what to do. Yes, and in the work of many SOCs, the basic playbooks for responding and blocking can be performed not even by IBs, but by IT specialists. This is a good field for the possible development of AI or simpler approaches to automation.
But, as always, there are nuances ...
A. Once again, I emphasize that for the successful work of AI at this stage, it is necessary that the previous one go through a human analyst, and do this as fully and efficiently as possible. This is also not always an easy task.
B. On the part of IT and business, you will encounter a sharp rejection of the automation of even basic playbooks for responding (blocking IP addresses and accounts, isolating a workstation), since all this is fraught with downtime and disruption of business processes. And, while this paradigm has not been successfully tested by practice and time - at least in semi-manual mode on the go-ahead from the analyst, it is probably premature to talk about transferring functions to a machine.
Now let's look at the situation as a whole. Some processes are not alienated in favor of AI, some require elaboration and maintenance of the full infrastructure context. It seems that the time for the widespread adoption of these technologies has not come yet - the only exception is the task of improving the quality of incident detection by identifying anomalies. However, there is reason to believe that the listed SOC tasks are, in principle, amenable to automation, which means that in the long run, AI can well find its place there.
Skynet is not ready to win
In the final, I would like to highlight a few very important, in our opinion, points that allow one to answer the common question: “Can the AI replace me with the first line / command of Threat hunting / SOC?”
Firstly, even in very large streamlined and automated industries, where most of the functionality is given to machines, the operator is always present. This can be observed in any of the sectors of our economy. The tasks of the operator in this sense are deterministically simple - by their human factor eliminate the “machine factor” and stabilize the situation with their own hands in the event of a failure / accident / violation of the correctness of the process. If we automate or cybernetize SOC tasks, then automatically there is a need to attract a strong specialized specialist who is able to quickly assess impact from machine error and the effectiveness of the actions taken. Therefore, automation and the development of AI, even in the future, is unlikely to lead to the rejection of a round-the-clock duty shift.
Secondly, as we saw, any AI in one way or another requires replenishment of knowledge and feedback. And in the case of SOC - not only about changing attack vectors or the external information context (which in theory can be part of training / expert packages, etc.), but, first of all, the information context of your incidents, your organization and business processes. This means that AI will not be able to replace full-time expert analysts. At least in the near future.
Thus, in our opinion, any approaches to the integration of AI in SOC at the current stage can be considered only as elements of automation of work with the context and the solution of some analytic subtasks. Such a complex process as providing information security is not yet ready for the complete transfer to robots.