рдирдорд╕реНрдХрд╛рд░ред рдореИрдВ c # рдореЗрдВ
рдорд╢реАрди рд▓рд░реНрдирд┐рдВрдЧ рдХреЗ рддрд░реАрдХреЛрдВ рдХреЛ рд▓рд╛рдЧреВ рдХрд░рдиреЗ рдХреЗ
рд╡рд┐рд╖рдп рдХреЛ рдЬрд╛рд░реА рд░рдЦрдирд╛ рдЪрд╛рд╣рддрд╛ рд╣реВрдВ, рдФрд░ рдЗрд╕ рд▓реЗрдЦ рдореЗрдВ рдореИрдВ рд╕реАрдзреЗ рд╡рд┐рддрд░рдг
рддрдВрддреНрд░рд┐рдХрд╛ рдиреЗрдЯрд╡рд░реНрдХ рдХреЗ рдкреНрд░рд╢рд┐рдХреНрд╖рдг рдХреЗ рд▓рд┐рдП
рддреНрд░реБрдЯрд┐ рд╡рд╛рдкрд╕ рдкреНрд░рд╕рд╛рд░ рдПрд▓реНрдЧреЛрд░рд┐рджрдо рдХреЗ рдмрд╛рд░реЗ
рдореЗрдВ рдмрд╛рдд рдХрд░реВрдВрдЧрд╛, рдФрд░ рд╕реА # рдореЗрдВ рдЗрд╕рдХреЗ рдХрд╛рд░реНрдпрд╛рдиреНрд╡рдпрди рдХреЛ рднреА рджреЗ рджреВрдВрдЧрд╛ред рдЗрд╕ рдХрд╛рд░реНрдпрд╛рдиреНрд╡рдпрди рдХреА рдЦрд╝рд╛рд╕рд┐рдпрдд рдпрд╣ рд╣реИ рдХрд┐ рдПрд▓реНрдЧреЛрд░рд┐рдереНрдо рдХрд╛ рдХрд╛рд░реНрдпрд╛рдиреНрд╡рдпрди рдЙрджреНрджреЗрд╢реНрдп рдлрд╝рдВрдХреНрд╢рди (рдПрдХ рдЬрд┐рд╕реЗ рддрдВрддреНрд░рд┐рдХрд╛ рдиреЗрдЯрд╡рд░реНрдХ рдХреЛ рдХрдо рдХрд░рдиреЗ рдХреА рдХреЛрд╢рд┐рд╢ рдХрд░ рд░рд╣рд╛ рд╣реИ) рдФрд░ рдиреНрдпреВрд░реЙрдиреНрд╕ рдХреЗ рд╕рдХреНрд░рд┐рдпрдг рдлрд╝рдВрдХреНрд╢рди рдХреЗ рдХрд╛рд░реНрдпрд╛рдиреНрд╡рдпрди рд╕реЗ рдЕрдореВрд░реНрдд рд╣реИред рдкрд░рд┐рдгрд╛рдо рдПрдХ рдкреНрд░рдХрд╛рд░ рдХрд╛ рдХрдВрд╕реНрдЯреНрд░рдХреНрдЯрд░ рд╣реИ, рдЬрд┐рд╕рдХреЗ рд╕рд╛рде рдЖрдк рдиреЗрдЯрд╡рд░реНрдХ рдХреЗ рд╡рд┐рднрд┐рдиреНрди рдорд╛рдкрджрдВрдбреЛрдВ рдФрд░ рд▓рд░реНрдирд┐рдВрдЧ рдПрд▓реНрдЧреЛрд░рд┐рджрдо рдХреЗ рд╕рд╛рде рдЦреЗрд▓ рд╕рдХрддреЗ рд╣реИрдВ, рдкрд░рд┐рдгрд╛рдо рджреЗрдЦ рдФрд░ рддреБрд▓рдирд╛ рдХрд░ рд╕рдХрддреЗ рд╣реИрдВред рдпрд╣ рдорд╛рдирд╛ рдЬрд╛рддрд╛ рд╣реИ рдХрд┐ рдЖрдк рдкрд╣рд▓реЗ рд╕реЗ рд╣реА рдкрд░рд┐рдЪрд┐рдд рд╣реИрдВ рдХрд┐ рдПрдХ рдХреГрддреНрд░рд┐рдо рддрдВрддреНрд░рд┐рдХрд╛ рдиреЗрдЯрд╡рд░реНрдХ рдХреНрдпрд╛ рд╣реИ (рдпрджрд┐ рдирд╣реАрдВ, рддреЛ рдореИрдВ рджреГрдврд╝рддрд╛ рд╕реЗ рдЕрдиреБрд╢рдВрд╕рд╛ рдХрд░рддрд╛ рд╣реВрдВ рдХрд┐ рдЖрдк рдкрд╣рд▓реЗ
рд╡рд┐рдХрд┐рдкреАрдбрд┐рдпрд╛ рдпрд╛
рдЗрдирдореЗрдВ рд╕реЗ рдПрдХ рд▓реЗрдЦ рдХрд╛ рдЕрдзреНрдпрдпрди
рдХрд░реЗрдВ )ред рд░реБрдЪрд┐ рд░рдЦрддреЗ рд╣реИрдВ? рд╣рдо рдмрд┐рд▓реНрд▓реА рдХреЗ рдиреАрдЪреЗ рдЪрдврд╝рддреЗ рд╣реИрдВред
рдкрджрдирд╛рдо
рд╢реБрд░реВ рдХрд░рдиреЗ рдХреЗ рд▓рд┐рдП, рд╣рдо рдЙрд╕ рдЕрдВрдХрди рдкрд░ рд╡рд┐рдЪрд╛рд░ рдХрд░реЗрдВрдЧреЗ рдЬрд┐рд╕рдХрд╛ рдЙрдкрдпреЛрдЧ рдореИрдВ рд▓реЗрдЦ рдореЗрдВ рдХрд░реВрдВрдЧрд╛, рдФрд░ рдПрдХ рдХреЗ рд▓рд┐рдП рд╣рдо рдмреБрдирд┐рдпрд╛рджреА рдЕрд╡рдзрд╛рд░рдгрд╛рдУрдВ рдХреЛ рдпрд╛рдж рдХрд░реЗрдВрдЧреЗ, рдореИрдВ рдиреНрдпреВрд░реЙрдиреНрд╕ рдФрд░ рдкрд░рддреЛрдВ рдХреЗ рд╕рд╛рде рдЪрд┐рддреНрд░ рдирд╣реАрдВ рджреВрдВрдЧрд╛, рдпрд╣ рд╕рдм
рд╡рд┐рдХрд┐рдкреАрдбрд┐рдпрд╛ рдФрд░ рдпрд╣рд╛рдБ рд╣рдм рдкрд░ рд╣реИ рддреЛ, рддреБрд░рдВрдд рдпреБрджреНрдз рдореЗрдВ, рдиреНрдпреВрд░реЙрди рдХрд╛ рдкреНрд░реЗрд░рд┐рдд рд╕реНрдерд╛рдиреАрдп рдХреНрд╖реЗрддреНрд░ (рдпрд╛ рд╕рд┐рд░реНрдл рдПрдХ рдпреЛрдЬрдХ) рдРрд╕рд╛ рджрд┐рдЦрддрд╛ рд╣реИ:
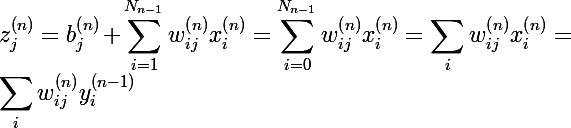
рдкрд░рдд n рдХреЗ jth рдиреНрдпреВрд░реЙрди рдХреЗ рднрд╛рд░ рдХреЗ рд╡реЗрдХреНрдЯрд░ рдФрд░ рдЗрдирдкреБрдЯ рдорд╛рдиреЛрдВ рдХреЗ рд╡реЗрдХреНрдЯрд░ рдХреЗ рд░реИрдЦрд┐рдХ рд╕рдВрдпреЛрдЬрди рдХрд╛ рдорд╛рди рд╣реИ- рдмреА - рдПрдХ рдиреНрдпреВрд░реЙрди рдХреА рдкрд╛рд░реА рдпрд╛ рд╡рд┐рд╕реНрдерд╛рдкрди; рдпрджрд┐ рд╣рдо рд╕рд╣рдордд рд╣реИрдВ рдХрд┐ рдЗрдирдкреБрдЯ рд╡реЗрдХреНрдЯрд░ рдХреЗ рд╢реВрдиреНрдп рдорд╛рди рдореЗрдВ рд╣рдореЗрд╢рд╛ рдПрдХ рд╣реЛрддрд╛ рд╣реИ
, рдлрд┐рд░ рд╢рд┐рдлреНрдЯ рдХреЛ рд╢реВрдиреНрдп рд╕реВрдЪрдХрд╛рдВрдХ рдХреЗ рд╕рд╛рде рд╡рдЬрди рдХреЗ рд░реВрдк рдореЗрдВ рдирд╛рдорд┐рдд рдХрд┐рдпрд╛ рдЬрд╛ рд╕рдХрддрд╛ рд╣реИ, рдФрд░ рд╕реВрддреНрд░ рдХреЛ рд╕рд░рд▓ рдмрдирд╛рдпрд╛ рдЬрд╛ рд╕рдХрддрд╛ рд╣реИ
- рдкрд░рдд рдиреНрдпреВрд░реЙрдиреНрд╕ рдХреА рд╕рдВрдЦреНрдпрд╛ n
рдиреНрдпреВрд░реЙрди рд╕рдХреНрд░рд┐рдпрдг рдлрд╝рдВрдХреНрд╢рди, рдпрд╛ рдпреЛрдЬрдХ рдорд╛рди рдХрд╛ рд╕реНрдерд╛рдирд╛рдВрддрд░рдг рдлрд╝рдВрдХреНрд╢рди:
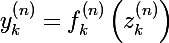
- рдиреЗрдЯрд╡рд░реНрдХ рдХреЗ рдкреНрд░рддреНрдпреЗрдХ рдиреНрдпреВрд░реЙрди рдХрд╛ рдЕрдкрдирд╛ рд╕рдХреНрд░рд┐рдпрдг рдХрд╛рд░реНрдп рд╣реЛ рд╕рдХрддрд╛ рд╣реИ
- рдкрд╣рд▓реЗ рдХреЛ рдЫреЛрдбрд╝рдХрд░ рд╕рднреА рдкрд░рддреЛрдВ рдХреЗ рд▓рд┐рдП, рдЗрдирдкреБрдЯ рд╡реЗрдХреНрдЯрд░ рдкрд┐рдЫрд▓реА рдкрд░рдд рдХрд╛ рдЖрдЙрдЯрдкреБрдЯ рд╡реЗрдХреНрдЯрд░ рд╣реЛрдЧрд╛, рдЗрд╕рд▓рд┐рдП

рдЪрд▓рд┐рдП рдиреНрдпреВрд░реЙрди рд╕реЗ рд╣реА рдиреЗрдЯрд╡рд░реНрдХ рдХреА рдУрд░ рдмрдврд╝рддреЗ рд╣реИрдВред рдПрдХ рддрдВрддреНрд░рд┐рдХрд╛ рдиреЗрдЯрд╡рд░реНрдХ рдПрдХ рдореЙрдбрд▓ рд╣реИ, рдЗрд╕рдореЗрдВ рдкреИрд░рд╛рдореАрдЯрд░ рд╣реИрдВ, рдФрд░ рд▓рд░реНрдирд┐рдВрдЧ рдПрд▓реНрдЧреЛрд░рд┐рджрдо рдХрд╛ рдХрд╛рд░реНрдп рддреНрд░реБрдЯрд┐ рдиреЗрдЯрд╡рд░реНрдХ рдХреЗ рдореВрд▓реНрдп рдХреЛ рдХрдо рдХрд░рдиреЗ рдХреЗ рд▓рд┐рдП рдРрд╕реЗ рдиреЗрдЯрд╡рд░реНрдХ рдорд╛рдкрджрдВрдбреЛрдВ рдХрд╛ рдЪрдпрди рдХрд░рдирд╛ рд╣реИред рддреНрд░реБрдЯрд┐ рдлрд╝рдВрдХреНрд╢рди рдХреЛ
рдИ рджреНрд╡рд╛рд░рд╛ рдЪрд┐рд╣реНрдирд┐рдд рдХрд┐рдпрд╛ рдЬрд╛рдПрдЧрд╛
ред рдореЙрдбрд▓ рдХреЗ рдкреИрд░рд╛рдореАрдЯрд░ рдиреНрдпреВрд░реЙрдиреНрд╕ рдХреЗ рд╡рдЬрди рд╣реИрдВ:

- рд▓реЗрдпрд░ n рдХреЗ jth рдиреНрдпреВрд░реЙрди рдХрд╛ рд╡рдЬрди, рдЬреЛ рд▓реЗрдпрд░ рдХреЗ i-th рдиреНрдпреВрд░реЙрди (n - 1) рдореЗрдВ рдЙрддреНрдкрдиреНрди рд╣реЛрддрд╛ рд╣реИред
рдпрд╣ рдЧреНрд░реАрдХ

рд╣рдо рд╕реАрдЦрдиреЗ рдХреЗ рдПрд▓реНрдЧреЛрд░рд┐рдереНрдо рдХреЗ рд╣рд╛рдЗрдкрд░рдкреИрд░рдореАрдЯрд░ рдХреЛ рдирд┐рд░реВрдкрд┐рдд рдХрд░рддреЗ рд╣реИрдВ - рд╕реАрдЦрдиреЗ рдХреА рдЧрддрд┐ред
рд╡рдЬрди рдореЗрдВ рдкрд░рд┐рд╡рд░реНрддрди
рдбреЗрд▓реНрдЯрд╛ рджреНрд╡рд╛рд░рд╛ рджрд░реНрд╢рд╛рдпрд╛ рдЧрдпрд╛
рд╣реИ :

- рдЧреНрд░реЗрдбрд┐рдПрдВрдЯ рдХреА рджрд┐рд╢рд╛ рд╣рдореЗрдВ рдлрд╝рдВрдХреНрд╢рди рдХреЗ рдорд╛рди рдХреЛ рдмрдврд╝рд╛рдиреЗ рдХреА рджрд┐рд╢рд╛ рдореЗрдВ рджрд┐рдЦрд╛рддреА рд╣реИ, рд▓реЗрдХрд┐рди рдЗрд╕реЗ рдХрдо рдХрд░рдиреЗ рдХреЗ рд▓рд┐рдП, рд╣рдореЗрдВ рд╡рд┐рдкрд░реАрдд рджрд┐рд╢рд╛ рдореЗрдВ рдЬрд╛рдиреЗ рдХреА рдЖрд╡рд╢реНрдпрдХрддрд╛ рд╣реИ
рдЗрд╕ рдкреНрд░рдХрд╛рд░, рдирдпрд╛ рдиреНрдпреВрд░реЙрди рд╡рдЬрди рдирд┐рдореНрдирд╛рдиреБрд╕рд╛рд░ рд╣реИ:

рдпрд╣ рдЙрд▓реНрд▓реЗрдЦрдиреАрдп рд╣реИ рдХрд┐ рд╡рдЬрди рдореЗрдВ рдмрджрд▓рд╛рд╡ рдХреЗ рд▓рд┐рдП рдирд┐рдпрдорд┐рддреАрдХрд░рдг рдХреЛ рдЕрднреА рднреА (рдпрд╛ рдмрд▓реНрдХрд┐ рдЖрд╡рд╢реНрдпрдХ) рдЬреЛрдбрд╝рд╛ рдЬрд╛ рд╕рдХрддрд╛ рд╣реИред рдирд┐рдпрдорд┐рддрд┐рдХрд░рдг рдлрд╝рдВрдХреНрд╢рди
рдЖрд░ рдореЙрдбрд▓ рдорд╛рдкрджрдВрдбреЛрдВ рдХрд╛ рдПрдХ рдХрд╛рд░реНрдп рд╣реИ, рд╣рдорд╛рд░реЗ рдорд╛рдорд▓реЗ рдореЗрдВ, рдпреЗ рдиреНрдпреВрд░реЙрдиреНрд╕ рдХреЗ рд╡рдЬрди рд╣реИрдВред рдЗрд╕ рдкреНрд░рдХрд╛рд░, рдирдпрд╛ рддреНрд░реБрдЯрд┐ рдлрд╝рдВрдХреНрд╢рди
рдИ + рдЖрд░ рдХреА рддрд░рд╣ рджрд┐рдЦрддрд╛ рд╣реИ, рдФрд░ рд╡рдЬрди рдмрджрд▓рдиреЗ рдХрд╛ рд╕реВрддреНрд░ рдирд┐рдореНрди рдореЗрдВ рдкрд░рд┐рд╡рд░реНрддрд┐рдд рд╣реЛрддрд╛ рд╣реИ:
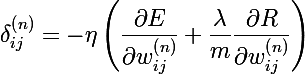
- рд▓реИрдореНрдмреНрдбрд╛ - рд╕реАрдЦрдиреЗ рдХрд╛ рд╣рд╛рдЗрдкрд░рдкреИрд░рд╛рдореАрдЯрд░, рдирд┐рдпрдорд┐рддреАрдХрд░рдг рдЧреБрдгрд╛рдВрдХ (рд╕реАрдЦрдиреЗ рдХреА рдЧрддрд┐ рдХреЗ рд╕рдорд╛рди)
- рдореА - рдкреНрд░рд╢рд┐рдХреНрд╖рдг рдирдореВрдиреЗ рдХрд╛ рдЖрдХрд╛рд░
рд╕рд╛рдорд╛рдиреНрдп рддреМрд░ рдкрд░, рдирд┐рдпрдорд┐рддреАрдХрд░рдг рдХреЗ рдХрд╛рд░реНрдпрд╛рдиреНрд╡рдпрди рдХреЛ рд╕реАрдЦрдиреЗ рдХреЗ рдПрд▓реНрдЧреЛрд░рд┐рдереНрдо рд╕реЗ рдЕрд▓рдЧ рдХрд┐рдпрд╛ рдЬрд╛ рд╕рдХрддрд╛ рд╣реИ, рд▓реЗрдХрд┐рди рдореИрдВ рдЗрд╕реЗ рдЕрднреА рддрдХ рдирд╣реАрдВ рдХрд░реВрдВрдЧрд╛, рдХреНрдпреЛрдВрдХрд┐ рд╕реАрдЦрдиреЗ рдХреЗ рдПрд▓реНрдЧреЛрд░рд┐рдереНрдо рдХрд╛ рд╡рд░реНрддрдорд╛рди рдХрд╛рд░реНрдпрд╛рдиреНрд╡рдпрди рд╡реИрд╕реЗ рднреА рд╕рдмрд╕реЗ рддреЗрдЬрд╝ рдирд╣реАрдВ рд╣реИ, рдХреНрдпреЛрдВрдХрд┐ рдЕрдиреНрдпрдерд╛, рдкреНрд░рддреНрдпреЗрдХ рд╕реАрдЦрдиреЗ рдХреЗ рдпреБрдЧ рдореЗрдВ (рд╕рднреА рдкреНрд░рд╢рд┐рдХреНрд╖рдг рдЙрджрд╛рд╣рд░рдгреЛрдВ рдХреЛ рдЪрд▓рд╛рдиреЗ), рдЖрдкрдХреЛ рдПрдХ рдЪрдХреНрд░ рдореЗрдВ рдЧрд┐рдирдирд╛ рд╣реЛрдЧрд╛ред рд╕рдВрдЪрд┐рдд рддреНрд░реБрдЯрд┐, рдФрд░ рджреВрд╕рд░реЗ рдореЗрдВ - рдирд┐рдпрдорд┐рддреАрдХрд░рдгред рдПрдХ рдФрд░ рдХрд╛рд░рдг рдпрд╣ рд╣реИ рдХрд┐ рдХрдИ рдкреНрд░рдХрд╛рд░ рдХреЗ рдирд┐рдпрдорд┐рддреАрдХрд░рдг рдирд╣реАрдВ рд╣реИрдВ (рдЙрджрд╛рд╣рд░рдг рдХреЗ рд▓рд┐рдП, рдореИрдВ рдХреЗрд╡рд▓ рдПрд▓ 1 рдФрд░ рдПрд▓ 2 рдЬрд╛рдирддрд╛ рд╣реВрдВ) рдЬреЛ рдХрд┐ рддрдВрддреНрд░рд┐рдХрд╛ рдиреЗрдЯрд╡рд░реНрдХ рдХреЛ рдкреНрд░рд╢рд┐рдХреНрд╖рд┐рдд рдХрд░рдиреЗ рдореЗрдВ рдЙрдкрдпреЛрдЧ рдХрд┐рдпрд╛ рдЬрд╛рддрд╛ рд╣реИред рдЗрд╕ рдХрд╛рд░реНрдпрд╛рдиреНрд╡рдпрди рдореЗрдВ, рдореИрдВ рдПрд▓ 2 рдорд╛рдирдХ рдХрд╛ рдЙрдкрдпреЛрдЧ рдХрд░реВрдВрдЧрд╛, рдФрд░ рдпрд╣ рд╕реАрдЦрдиреЗ рдХреЗ рдПрд▓реНрдЧреЛрд░рд┐рдереНрдо рдХрд╛ рдПрдХ рдЕрднрд┐рдиреНрди рдЕрдВрдЧ рд╣реЛрдЧрд╛ред

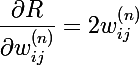
рддреНрд░реБрдЯрд┐ рдкреНрд░рдЪрд╛рд░ рдПрд▓реНрдЧреЛрд░рд┐рдердо
рд╕рдмрд╕реЗ рдкрд╣рд▓реЗ, рдкреНрд░рд╢рд┐рдХреНрд╖рдг рдореЛрдб рдкрд░ рдзреНрдпрд╛рди рджреЗрдВред рд╡рдЬрд╝рди рдмрджрд▓рдиреЗ рдХреЗ рдХрдИ рддрд░реАрдХреЗ рд╣реИрдВ:
- рдпрд╛ рдкреНрд░рддреНрдпреЗрдХ рдкреНрд░рд╢рд┐рдХреНрд╖рдг рдЙрджрд╛рд╣рд░рдг рдХреЗ рдмрд╛рдж (рд╡рд╛рд╕реНрддрд╡рд┐рдХ рд╕рдордп рдкреНрд░рд╢рд┐рдХреНрд╖рдг, рдСрдирд▓рд╛рдЗрди рдкреНрд░рд╢рд┐рдХреНрд╖рдг, рдмреИрдЪрд╕рд╛рдЗрдЬрд╝ = 1)
- рдпрд╛ рддреЛ рдкреВрд░реЗ рдкреНрд░рд╢рд┐рдХреНрд╖рдг рдирдореВрдиреЗ рдХреЗ рд▓рд┐рдП рдкрд░рд┐рд╡рд░реНрддрди рдЬрдорд╛ рдХрд░реЗрдВ, рдФрд░ рдлрд┐рд░ рд╕рднреА рднрд╛рд░ (рдкреВрд░реНрдг-рдмреИрдЪ, рдмреИрдЪрд╕рд╛рдЗрдЬрд╝ = рдкреНрд░рд╢рд┐рдХреНрд╖рдгрд╕реЗрдЯред рдЧрддрд┐) рдХреЛ рдмрджрд▓реЗрдВ
- рдпрд╛ рдкреНрд░рд╢рд┐рдХреНрд╖рдг рдХреЗ рдЙрджрд╛рд╣рд░рдгреЛрдВ рдХреА рдПрдХ рдирд┐рд╢реНрдЪрд┐рдд рд╕рдВрдЦреНрдпрд╛ рдХреЛ рдЪрд▓рд╛рдиреЗ рдХреЗ рдмрд╛рдж (рдорд┐рдиреА-рдмреИрдЪ, рдмреИрдЪрд╕рд╛рдЗрдЬрд╝ = any_number <trainingSet.Length)
рдСрдирд▓рд╛рдЗрди рд╕реАрдЦрдиреЗ рдХреЗ рд╕рд╛рде рд╕реНрдерд┐рддрд┐ рдкрд░ рд╡рд┐рдЪрд╛рд░ рдХрд░реЗрдВ, рдпрд╣ рдЖрд╕рд╛рди рд╣реЛрдЧрд╛ред рддреЛ, рдЧрддрд┐ рдиреЗрдЯрд╡рд░реНрдХ рдЗрдирдкреБрдЯ рдХреЗ рд▓рд┐рдП рдЖрдпрд╛ рдерд╛

рдиреЗрдЯрд╡рд░реНрдХ рдиреЗ рдЬрд╡рд╛рдм рджрд┐рдпрд╛

рд╣рд╛рд▓рд╛рдВрдХрд┐
рдПрдХреНрд╕ рдХреЗ рд▓рд┐рдП рд╕рд╣реА рдкреНрд░рддрд┐рдХреНрд░рд┐рдпрд╛ рд╣реИ

ред
рддреНрд░реБрдЯрд┐ рдлрд╝рдВрдХреНрд╢рди
E рдХреЗ рдЖрдВрд╢рд┐рдХ рд╡реНрдпреБрддреНрдкрдиреНрди рдкрд░ рд╡рд┐рдЪрд╛рд░ рдХрд░реЗрдВ:

- рд▓реЗрдХрд┐рди рдЪреВрдВрдХрд┐ рддреНрд░реБрдЯрд┐ рдлрд╝рдВрдХреНрд╢рди рдХреЗрд╡рд▓ рдпреЛрдЬрдХ рдХреЗ рдорд╛рдзреНрдпрдо рд╕реЗ рд╡реНрдпрдХреНрдд рдХрд┐рдпрд╛ рдЬрд╛ рд╕рдХрддрд╛ рд╣реИ, рдФрд░ рдпреЛрдЬрдХ рд╡рдЬрди рдкрд░ рдирд┐рд░реНрднрд░ рдХрд░рддрд╛ рд╣реИ, рд╣рдо рдЗрд╕ рдкреНрд░рд╡рд┐рд╖реНрдЯрд┐ рдХреЛ рдЗрд╕ рдкреНрд░рдХрд╛рд░ рдлрд┐рд░ рд╕реЗ рд▓рд┐рдЦ рд╕рдХрддреЗ рд╣реИрдВ


- рдЗрд╕ рдкреНрд░рдХрд╛рд░, рд╡рдЬрди рджреНрд╡рд╛рд░рд╛ рдпреЛрдЬрдХ рдХреЗ рдЖрдВрд╢рд┐рдХ рд╡реНрдпреБрддреНрдкрдиреНрди рдХреА рдЧрдгрдирд╛ рдХреА рдЬрд╛рддреА рд╣реИ, рдлрд┐рд░ рд╣рдо рд╡рдЬрди рджреНрд╡рд╛рд░рд╛ рдЙрджреНрджреЗрд╢реНрдп рдлрд╝рдВрдХреНрд╢рди рдХреЗ рдЖрдВрд╢рд┐рдХ рд╡реНрдпреБрддреНрдкрдиреНрди рдХреА рдЕрднрд┐рд╡реНрдпрдХреНрддрд┐ рдХреЛ рдлрд┐рд░ рд╕реЗ рд▓рд┐рдЦ рд╕рдХрддреЗ рд╣реИрдВ; рдЕрдВрдд рдореЗрдВ рд╣рдо рдкреНрд░рд╛рдкреНрдд рдХрд░рддреЗ рд╣реИрдВ

рдЖрдЧреЗ рдХреА рдЪрд░реНрдЪрд╛ рдХреЛ рджреЛ рд╢рд╛рдЦрд╛рдУрдВ рдореЗрдВ рд╡рд┐рднрд╛рдЬрд┐рдд рдХрд┐рдпрд╛ рдЧрдпрд╛ рд╣реИ: рдЕрдВрддрд┐рдо рдкрд░рдд рдХреЗ рд▓рд┐рдП рдФрд░ рд╢реЗрд╖ рдкрд░рддреЛрдВ рдХреЗ рд▓рд┐рдПред
рдЖрдЙрдЯрдкреБрдЯ рд▓реЗрдпрд░
рдЖрдЙрдЯрдкреБрдЯ рд▓реЗрдпрд░ рдХреЗ рд▓рд┐рдП, рд╕рдм рдХреБрдЫ рд╕рд░рд▓ рд╣реИ, рддреНрд░реБрдЯрд┐ рд╕реБрдзрд╛рд░ рдХреЗ рд▓рд┐рдП, рд╣рдореЗрдВ рдХреЗрд╡рд▓ рдПрдХ рд╡реЗрдЯ рдХреЗ рдЕрдиреБрд╕рд╛рд░ рдСрдмреНрдЬреЗрдХреНрдЯрд┐рд╡ рдлрд╝рдВрдХреНрд╢рди рдХреЗ рд╡реНрдпреБрддреНрдкрдиреНрди рдХреА рдЧрдгрдирд╛ рдХрд░рдиреЗ рдФрд░ рдбреЗрд▓реНрдЯрд╛ рдорд╛рди рдХреА рдЧрдгрдирд╛ рдХрд░рдиреЗ рдХреА рдЖрд╡рд╢реНрдпрдХрддрд╛ рд╣реИред рд╣рдо рдЗрд╕ рдмрд╛рдд рдХреЛ рдзреНрдпрд╛рди рдореЗрдВ рд░рдЦрддреЗ рд╣реИрдВ рдХрд┐ рдЙрджреНрджреЗрд╢реНрдп рдлрд╝рдВрдХреНрд╢рди рдкреВрд░реА рддрд░рд╣ рд╕реЗ рдХреЗрд╡рд▓ рдиреНрдпреВрд░реЙрди рдХреЗ рдЖрдЙрдЯрдкреБрдЯ рдореВрд▓реНрдп, рдпрд╛ рд╕рдХреНрд░рд┐рдпрдг рдлрд╝рдВрдХреНрд╢рди рдХреЗ рдореВрд▓реНрдп рдкрд░ рдирд┐рд░реНрднрд░ рдХрд░рддрд╛ рд╣реИ, рдФрд░ рд╕рдХреНрд░рд┐рдпрдг рдлрд╝рдВрдХреНрд╢рди рдХреЗрд╡рд▓ рдпреЛрдЬрдХ рдкрд░ рдирд┐рд░реНрднрд░ рдХрд░рддрд╛ рд╣реИ

рдпрд╣рд╛рдВ рдпрд╣ рджреЗрдЦрд╛ рдЬрд╛ рд╕рдХрддрд╛ рд╣реИ рдХрд┐ рдЖрдЙрдЯрдкреБрдЯ рд▓реЗрдпрд░ рдХреА рддреНрд░реБрдЯрд┐ рдХреА рдЧрдгрдирд╛ рдХрд░рдиреЗ рдХреЗ рд▓рд┐рдП, рд╣рдорд╛рд░реЗ рд▓рдХреНрд╖реНрдп рдлрд╝рдВрдХреНрд╢рди рдпрд╛ рдиреНрдпреВрд░реЙрди рдХрд╛ рд╕рдХреНрд░рд┐рдпрдг рдлрд╝рдВрдХреНрд╢рди рдХреНрдпрд╛ рд╣реИ, рдЗрд╕рдХреА рдкрд░рд╡рд╛рд╣ рдХрд┐рдП рдмрд┐рдирд╛, рдмрд┐рдВрджреБрдУрдВ рдкрд░ рдЖрдВрд╢рд┐рдХ рдбреЗрд░рд┐рд╡реЗрдЯрд┐рд╡ рдХреЗ рдореВрд▓реНрдп рдХреА рдЧрдгрдирд╛ рдХрд░рдирд╛ рдЖрд╡рд╢реНрдпрдХ рд╣реИред
рдХреЛрдИ рдЫрд┐рдкреА рд╣реБрдИ рдкрд░рдд
рд▓реЗрдХрд┐рди рдЕрдЧрд░ рдкрд░рдд рдЖрдЙрдЯрдкреБрдЯ рдирд╣реАрдВ рд╣реИ, рддреЛ рд╣рдореЗрдВ рдмрд╛рдж рдХреА рд╕рднреА рдкрд░рддреЛрдВ рдХреЗ рддреНрд░реБрдЯрд┐ рдорд╛рдиреЛрдВ рдХреЛ рдЬрдорд╛ рдХрд░рдиреЗ рдХреА рдЖрд╡рд╢реНрдпрдХрддрд╛ рд╣реИред

- рдЖрдЧреЗ рд╣рдо рдЗрд╕ рдмрд╛рдд рдХрд╛ рдзреНрдпрд╛рди рд░рдЦрддреЗ рд╣реИрдВ рдХрд┐ рдЕрдЧрд▓реА рдкрд░рдд рдХрд╛ рдпреЛрдЬрдХ рдХреЗрд╡рд▓ рд╡рд░реНрддрдорд╛рди рдкрд░рдд рдХреЗ рдЖрдЙрдЯрдкреБрдЯ рдкрд░ рдирд┐рд░реНрднрд░ рдХрд░рддрд╛ рд╣реИ, рдФрд░ рд╡рд░реНрддрдорд╛рди рдХреЗ рдЖрдЙрдЯрдкреБрдЯ рдХреЗрд╡рд▓ рд╡рд░реНрддрдорд╛рди рдкрд░рдд рдХреЗ рдпреЛрдЬрдХ рдкрд░ рдирд┐рд░реНрднрд░ рдХрд░рддреЗ рд╣реИрдВ

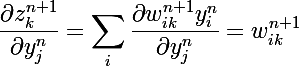
- рдкрд┐рдЫрд▓реА рдкрд░рдд рдХреЗ рдЖрдЙрдЯрдкреБрдЯ рдкрд░ рдпреЛрдЬрдХ рдХреЗ рд╡реНрдпреБрддреНрдкрдиреНрди рд╣рдореЗрдВ рдиреНрдпреВрд░реЙрдиреНрд╕ рдХреЗ рдмреАрдЪ рд╕рд┐рд░реНрдл рд╡рдЬрди рджреЗрдВрдЧреЗ, рдЗрд╕реЗ рджреЗрдЦрддреЗ рд╣реБрдП рд╣рдо рдореБрдЦреНрдп рдирд┐рд╖реНрдХрд░реНрд╖ рдХреЛ рдлрд┐рд░ рд╕реЗ рд▓рд┐рдЦрддреЗ рд╣реИрдВред

рдкреБрдирд╢реНрдЪ: рдореИрдВрдиреЗ рджреЗрдЦрд╛ рд╣реИ рдХрд┐ рдореИрдВ рдпрд╣ рджрд░реНрд╢рд╛рдиреЗ рдХреЗ рд▓рд┐рдП рдХреЛрд╖реНрдардХ рдореЗрдВ рдХреЛрд╖реНрдардХ рд▓рдЧрд╛рдирд╛ рднреВрд▓ рдЧрдпрд╛ рдХрд┐ рдпрд╣ рдХреЛрдИ рдбрд┐рдЧреНрд░реА рдирд╣реАрдВ рд╣реИ, рдмрд▓реНрдХрд┐ рдПрдХ рдкрд░рдд рд╕реВрдЪрдХрд╛рдВрдХ рд╣реИ; рдЗрд╕реЗ рдзреНрдпрд╛рди рдореЗрдВ рд░рдЦреЗрдВ рдЬрдмрдХрд┐ рдХрд╣реАрдВ рднреА рдХреЛрдИ рдбрд┐рдЧреНрд░реА рдирд╣реАрдВ рд╣реИредрд╣рдорд╛рд░реЗ рдкрд╛рд╕ рдХреНрдпрд╛ рд╣реИ:
- рдПрдХ рдирд┐рд╢реНрдЪрд┐рдд рд╕рдХреНрд░рд┐рдпрдг рдлрд╝рдВрдХреНрд╢рди рдХреЗ рд╡реНрдпреБрддреНрдкрдиреНрди рдХреА рдЧрдгрдирд╛ рд╕рд┐рд░реНрдл рд╡рд╣реА рд╣реИ рдЬреЛ рдЖрдкрдХреЛ рдЪрд╛рд╣рд┐рдП
- рдЕрдЧрд▓реА рдкрд░рдд рдХреЗ рдпреЛрдЬрдХ рдХреЗ рдореВрд▓реНрдп рджреНрд╡рд╛рд░рд╛ рдЙрджреНрджреЗрд╢реНрдп рдлрд╝рдВрдХреНрд╢рди рдХреЗ рдЖрдВрд╢рд┐рдХ рд╡реНрдпреБрддреНрдкрдиреНрди рдХреА рдЧрдгрдирд╛; рдпрд╣рд╛рдВ рд╕рдм рдХреБрдЫ рднреА рд╕рд░рд▓ рд╣реИ, рдХреНрдпреЛрдВрдХрд┐ рд╣рдо рдЕрдВрддрд┐рдо рдкрд░рдд рдкрд░ рдирд╣реАрдВ рд╣реИрдВ, рдФрд░ рд╣рдо рдкрд┐рдЫрд▓реЗ рд╕реЗ рдкрд╣рд▓реЗ рддрдХ рд╡рдЬрди рдореЗрдВ рдмрджрд▓рд╛рд╡ рдХреА рдЧрдгрдирд╛ рдХрд░ рд░рд╣реЗ рд╣реИрдВ, рдЗрд╕рд▓рд┐рдП рдЗрд╕ рдореВрд▓реНрдп рдХреА рдЧрдгрдирд╛ рдкрд┐рдЫрд▓реЗ рдЪрд░рдг рдореЗрдВ рдкрд╣рд▓реЗ рд╣реА рдХреА рдЬрд╛ рдЪреБрдХреА рд╣реИ
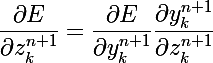
- рдпрджрд┐ рдЕрдЧрд▓реА рдкрд░рдд рдЕрдВрддрд┐рдо рд╣реИ, рддреЛ рд╣рдо рд╡рд░реНрддрдорд╛рди рдкрд░рдд рдХреЗ рд▓рд┐рдП рдЗрд╕ рдорд╛рди рдХреА рдЧрдгрдирд╛ рдХрд░реЗрдВрдЧреЗ, рдФрд░ рдЗрд╕ рддрд░рд╣ рдкреВрд░реЗ рдиреЗрдЯрд╡рд░реНрдХ рдХреЗ рд╕рдорд╛рдзрд╛рди рдХрд╛ рд╡рд┐рд╕реНрддрд╛рд░ рдХрд░реЗрдВрдЧреЗ
рдХрд╛рд░реНрдпрд╛рдиреНрд╡рдпрди
рддреНрд░реБрдЯрд┐ рд╕рдорд╛рд░реЛрд╣
рд╣рдордиреЗ рдлрд╝рд╛рд░реНрдореБрд▓реЛрдВ рдХреЛ рд╕рдорд╛рдкреНрдд рдХрд░ рджрд┐рдпрд╛ рд╣реИ, рдЖрдЗрдП рдХрд╛рд░реНрдпрд╛рдиреНрд╡рдпрди рдХреЗ рд▓рд┐рдП рдЖрдЧреЗ рдмрдврд╝реЗрдВ, рдФрд░ рдПрдХ рддреНрд░реБрдЯрд┐ рдлрд╝рдВрдХреНрд╢рди рдХреА рдЕрд╡рдзрд╛рд░рдгрд╛ рдХреЗ рд╕рд╛рде рд╢реБрд░реВ рдХрд░реЗрдВред рдореИрдВрдиреЗ рдЗрд╕реЗ рдПрдХ рдореАрдЯреНрд░рд┐рдХ рдХреЗ рд░реВрдк рдореЗрдВ рдкреНрд░рд╕реНрддреБрдд рдХрд┐рдпрд╛ рд╣реИ (рд╡рд╛рд╕реНрддрд╡ рдореЗрдВ, рдпрд╣ рдорд╛рдорд▓рд╛ рд╣реИ)ред
CalculatePartialDerivaitveByV2Index рд╡рд┐рдзрд┐ v2 рд╕реЗ рд╕реВрдЪрдХрд╛рдВрдХ рдЪрд░ рджреНрд╡рд╛рд░рд╛ рдЗрдирдкреБрдЯ рд╡реИрдХреНрдЯрд░ рдХреЗ рд▓рд┐рдП рдПрдХ рдлрд╝рдВрдХреНрд╢рди рдХреЗ рдЖрдВрд╢рд┐рдХ рд╡реНрдпреБрддреНрдкрдиреНрди рдХреЗ рдореВрд▓реНрдп рдХреА рдЧрдгрдирд╛ рдХрд░рддрд╛ рд╣реИред
public interface IMetrics<T> { double Calculate(T[] v1, T[] v2);
рдЗрд╕ рдкреНрд░рдХрд╛рд░, рд╣рдо рдиреЗрдЯрд╡рд░реНрдХ рдХреЗ рд╡рд╛рд╕реНрддрд╡рд┐рдХ рдЖрдЙрдЯрдкреБрдЯ рд╕реЗ рдЕрдВрддрд┐рдо рдкрд░рдд рдХреЗ рд▓рд┐рдП рддреНрд░реБрдЯрд┐ рдлрд╝рдВрдХреНрд╢рди рдХреЗ рдЖрдВрд╢рд┐рдХ рд╡реНрдпреБрддреНрдкрдиреНрди рдХреЗ рдореВрд▓реНрдп рдХреА рдЧрдгрдирд╛ рдХрд░ рд╕рдХрддреЗ рд╣реИрдВ

ред
рдЙрджрд╛рд╣рд░рдг рдХреЗ рд▓рд┐рдП, рдЖрдЗрдП рдХреБрдЫ рдХрд╛рд░реНрдпрд╛рдиреНрд╡рдпрди рд▓рд┐рдЦрддреЗ рд╣реИрдВред
рдпреВрдХреНрд▓рд┐рдбрд┐рдпрди рджреВрд░реА рдХреЗ рдЖрдзреЗ рд╡рд░реНрдЧ рдХреЛ рдХрдо рдХрд░рдирд╛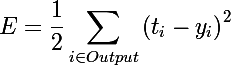
рдФрд░ рд╡реНрдпреБрддреНрдкрдиреНрди рдЗрд╕ рддрд░рд╣ рджрд┐рдЦреЗрдЧрд╛:

internal class HalfSquaredEuclidianDistance : IMetrics<T> { public override double Calculate(double[] v1, double[] v2) { double d = 0; for (int i = 0; i < v1.Length; i++) { d += (v1[i] - v2[i]) * (v1[i] - v2[i]); } return 0.5 * d; } public override double CalculatePartialDerivaitveByV2Index(double[] v1, double[] v2, int v2Index) { return v2[v2Index] - v1[v2Index]; } }
рд▓реЙрдЧ рд▓рд╛рдЗрдХреИрд▓рд┐рдЯреА рдХреЛ рдиреНрдпреВрдирддрдо рдХрд░рдирд╛

internal class Loglikelihood : IMetrics<double> { public override double Calculate(double[] v1, double[] v2) { double d = 0; for (int i = 0; i < v1.Length; i++) { d += v1[i]*Math.Log(v2[i]) + (1 - v1[i])*Math.Log(1 - v2[i]); } return -d; } public override double CalculatePartialDerivaitveByV2Index(double[] v1, double[] v2, int v2Index) { return -(v1[v2Index]/v2[v2Index] - (1 - v1[v2Index])/(1 - v2[v2Index])); } }
рдпрд╣рд╛рдВ рдореБрдЦреНрдп рдмрд╛рдд рдпрд╣ рдирд╣реАрдВ рднреВрд▓рдирд╛ рд╣реИ рдХрд┐ рд▓реЙрдЧрд░рд┐рджрдорд┐рдХ рд╕рдВрднрд╛рд╡рдирд╛ рдХреА рд╢реБрд░реБрдЖрдд рдореЗрдВ рдПрдХ рдЛрдг рдЪрд┐рд╣реНрди рдХреЗ рд╕рд╛рде рдЧрдгрдирд╛ рдХреА рдЬрд╛рддреА рд╣реИ, рдФрд░ рд╡реНрдпреБрддреНрдкрдиреНрди рднреА рдПрдХ рдЛрдг рдХреЗ рд╕рд╛рде рд╣реЛрдЧрд╛ред рдореИрдВ рдЪреЗрдХ рдкрд░ рдпрд╛ рд╢реВрдиреНрдп рд╕реЗ рд╡рд┐рднрд╛рдЬрди рдХреЗ рдорд╛рдорд▓реЛрдВ рд╕реЗ рдмрдЪрдиреЗ рдпрд╛ рд╢реВрдиреНрдп рдХреЗ рд▓рдШреБрдЧрдгрдХ рдкрд░ рдзреНрдпрд╛рди рдХреЗрдВрджреНрд░рд┐рдд рдирд╣реАрдВ рдХрд░рддрд╛ рд╣реВрдВред
рдиреНрдпреВрд░реЙрди рд╕рдХреНрд░рд┐рдпрдг рд╕рдорд╛рд░реЛрд╣
рдЗрд╕реА рддрд░рд╣ рд╕реЗ, рд╣рдо рдиреНрдпреВрд░реЙрди рд╕рдХреНрд░рд┐рдпрдг рдлрд╝рдВрдХреНрд╢рди рдХрд╛ рд╡рд░реНрдгрди рдХрд░рддреЗ рд╣реИрдВред
public interface IFunction { double Compute(double x); double ComputeFirstDerivative(double x); }
рдФрд░ рдЙрджрд╛рд╣рд░рдг рд╣реИред
рдЕрд╡рдЧреНрд░рд╣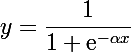

internal class SigmoidFunction : IFunction { private double _alpha = 1; internal SigmoidFunction(double alpha) { _alpha = alpha; } public double Compute(double x) { double r = (1 / (1 + Math.Exp(-1 * _alpha * x)));
рдЕрддрд┐рд╢рдпреЛрдХреНрддрд┐рдкреВрд░реНрдг рд╕реНрдкрд░реНрд╢рдЬреНрдпрд╛

internal class HyperbolicTangensFunction : IFunction { private double _alpha = 1; internal HyperbolicTangensFunction(double alpha) { _alpha = alpha; } public double Compute(double x) { return (Math.Tanh(_alpha * x)); } public double ComputeFirstDerivative(double x) { double t = Math.Tanh(_alpha*x); return _alpha*(1 - t*t); } }
рдиреНрдпреВрд░реЙрди, рдкрд░рдд рдФрд░ рдиреЗрдЯрд╡рд░реНрдХ
рдЗрд╕ рдЦрдВрдб рдореЗрдВ, рд╣рдо рдиреЗрдЯрд╡рд░реНрдХ рдХреЗ рдореБрдЦреНрдп рддрддреНрд╡реЛрдВ рдХреА рдкреНрд░рд╕реНрддреБрддрд┐ рдкрд░ рд╡рд┐рдЪрд╛рд░ рдХрд░реЗрдВрдЧреЗ, рдореИрдВ рдЙрдирдХрд╛ рдХрд╛рд░реНрдпрд╛рдиреНрд╡рдпрди рдирд╣реАрдВ рджреВрдВрдЧрд╛, рдХреНрдпреЛрдВрдХрд┐ рд╡рд╣ рд╕реНрдкрд╖реНрдЯ рд╣реИред рдПрд▓реНрдЧреЛрд░рд┐рдереНрдо рдкреВрд░реА рддрд░рд╣ рд╕реЗ рдЬреБрдбрд╝реЗ "рд╕реНрддрд░рд┐рдд" рдиреЗрдЯрд╡рд░реНрдХ рдХреЗ рд▓рд┐рдП рджрд┐рдпрд╛ рдЬрд╛рдПрдЧрд╛, рдЗрд╕рд▓рд┐рдП рдиреЗрдЯрд╡рд░реНрдХ рдХреЗ рдХрд╛рд░реНрдпрд╛рдиреНрд╡рдпрди рдХреЛ рддрджрдиреБрд╕рд╛рд░ рдХрд░рдиреЗ рдХреА рдЖрд╡рд╢реНрдпрдХрддрд╛ рд╣реЛрдЧреАред
рддреЛ, рдиреНрдпреВрд░реЙрди рдирд┐рдореНрдирд╛рдиреБрд╕рд╛рд░ рд╣реИред
public interface INeuron {
рдХреНрдпреЛрдВрдХрд┐ рд╣рдо рдкреВрд░реА рддрд░рд╣ рд╕реЗ рдЬреБрдбрд╝реЗ "рд╕реНрддрд░рд┐рдд" рдиреЗрдЯрд╡рд░реНрдХ рдкрд░ рд╡рд┐рдЪрд╛рд░ рдХрд░ рд░рд╣реЗ рд╣реИрдВ, рдлрд┐рд░ рдЪрд┐рд▓реНрдб рдФрд░ рдкреЗрд░реЗрдВрдЯреНрд╕ рдХреЛ рд▓рд╛рдЧреВ рдирд╣реАрдВ рдХрд┐рдпрд╛ рдЬрд╛ рд╕рдХрддрд╛ рд╣реИ, рд▓реЗрдХрд┐рди рдпрджрд┐ рдЖрдк рдПрдХ рд╕рд╛рдорд╛рдиреНрдп рдПрд▓реНрдЧреЛрд░рд┐рдереНрдо рдмрдирд╛рддреЗ рд╣реИрдВ, рддреЛ рдЖрдкрдХреЛ рдХрд░рдирд╛ рд╣реЛрдЧрд╛ред рдЙрди рдХреНрд╖реЗрддреНрд░реЛрдВ рдкрд░ рд╡рд┐рдЪрд╛рд░ рдХрд░реЗрдВ рдЬреЛ рд▓рд░реНрдирд┐рдВрдЧ рдПрд▓реНрдЧреЛрд░рд┐рджрдо рдХреЗ рд▓рд┐рдП рд╡рд┐рд╢реЗрд╖ рд░реВрдк рд╕реЗ рдорд╣рддреНрд╡рдкреВрд░реНрдг рд╣реИрдВ:
- рд▓рд╛рд╕реНрдЯрдиреЗрдЯ - рдиреНрдпреВрд░реЙрди рдпреЛрдЬрдХ, рдЕрдВрддрд┐рдо рдЧрдгрдирд╛ рдореВрд▓реНрдп рдпрд╣рд╛рдВ рд╕рдВрдЧреНрд░рд╣реАрдд рд╣реИ
- LastState - рдиреНрдпреВрд░реЙрди рдЖрдЙрдЯрдкреБрдЯ, рдЕрдВрддрд┐рдо рдЧрдгрдирд╛ рдореВрд▓реНрдп рдпрд╣рд╛рдВ рд╕рдВрдЧреНрд░рд╣реАрдд рд╣реИ
- dEdz рдиреНрдпреВрд░реЙрди рдХрд╛ рд╡рд╣реА dE / dz рд╣реИ рдЬреЛ рдКрдкрд░ рдЙрд▓реНрд▓рд┐рдЦрд┐рдд рд╣реИ, рдФрд░ рдпрд╣ рдЧрдгрдирд╛ рдХреА рдЬрд╛рддреА рд╣реИ рдХрд┐ рд╡рд░реНрддрдорд╛рди рдиреНрдпреВрд░реЙрди рдХрд┐рд╕ рдкрд░рдд рдкрд░ рдирд┐рд░реНрднрд░ рдХрд░рддрд╛ рд╣реИ; рдиреНрдпреВрд░реЙрди рдХреЗ рдпреЛрдЬрдХ рдХреЗ рд╕рдВрдмрдВрдз рдореЗрдВ рддреНрд░реБрдЯрд┐ рдлрд╝рдВрдХреНрд╢рди рдХрд╛ рдЖрдВрд╢рд┐рдХ рд╡реНрдпреБрддреНрдкрдиреНрди
рдиреЗрдЯрд╡рд░реНрдХ рдкрд░рдд рд╕рд░рд▓ рджрд┐рдЦрддреА рд╣реИ:
public interface ILayer {
рдФрд░ рдиреЗрдЯрд╡рд░реНрдХ рджреГрд╢реНрдп:
public interface INeuralNetwork {
рд▓реЗрдХрд┐рди рд╣рдо рдПрдХ рдмрд╣реБрдкрд░рдд рддрдВрддреНрд░рд┐рдХрд╛ рдиреЗрдЯрд╡рд░реНрдХ рдкрд░ рд╡рд┐рдЪрд╛рд░ рдХрд░ рд░рд╣реЗ рд╣реИрдВ, рдЗрд╕рд▓рд┐рдП рдПрдХ рд╡рд┐рд╢реЗрд╖ рджреГрд╢реНрдп рдХрд╛ рдЙрдкрдпреЛрдЧ рдХрд┐рдпрд╛ рдЬрд╛рдПрдЧрд╛:
public interface IMultilayerNeuralNetwork : INeuralNetwork {
рд▓рд░реНрдирд┐рдВрдЧ рдПрд▓реНрдЧреЛрд░рд┐рджрдо
рд▓рд░реНрдирд┐рдВрдЧ рдПрд▓реНрдЧреЛрд░рд┐рджрдо рдХреЛ
рд░рдгрдиреАрддрд┐ рдкреИрдЯрд░реНрди рдХреЗ рдорд╛рдзреНрдпрдо рд╕реЗ рдХрд╛рд░реНрдпрд╛рдиреНрд╡рд┐рдд рдХрд┐рдпрд╛ рдЬрд╛рдПрдЧрд╛:
public interface ILearningStrategy<T> {
рдмреЗрд╣рддрд░ рд╕рдордЭ рдХреЗ рд▓рд┐рдП, рдореИрдВ рдЗрд╕ рдХрд╛рд░реНрдпрд╛рдиреНрд╡рдпрди рдХреЗ рд╕рдВрджрд░реНрдн рдореЗрдВ рдХрд┐рд╕реА рднреА рддрдВрддреНрд░рд┐рдХрд╛ рдиреЗрдЯрд╡рд░реНрдХ рдХрд╛ рдПрдХ рд╡рд┐рд╢рд┐рд╖реНрдЯ рдЯреНрд░реЗрди рдлрд╝рдВрдХреНрд╢рди рджреВрдВрдЧрд╛:
public void Train(IList<DataItem<double>> data) { _learningStrategy.Train(this, data); }
рдЗрдирдкреБрдЯ рдкреНрд░рд╛рд░реВрдк
рдореИрдВ рдирд┐рдореНрдирд▓рд┐рдЦрд┐рдд рдЗрдирдкреБрдЯ рдкреНрд░рд╛рд░реВрдк рдХрд╛ рдЙрдкрдпреЛрдЧ рдХрд░рддрд╛ рд╣реВрдВ:
public class DataItem<T> { private T[] _input = null; private T[] _output = null; public DataItem() { } public DataItem(T[] input, T[] output) { _input = input; _output = output; } public T[] Input { get { return _input; } set { _input = value; } } public T[] Output { get { return _output; } set { _output = value; } } }
рдЬреИрд╕рд╛ рдХрд┐ рдкрд┐рдЫрд▓реЗ рднрд╛рдЧреЛрдВ рдореЗрдВ рдХреЛрдб рд╕реЗ рджреЗрдЦрд╛ рдЧрдпрд╛ рд╣реИ, рддрдВрддреНрд░рд┐рдХрд╛ рдиреЗрдЯрд╡рд░реНрдХ рдХреЗ рд╕рд╛рде рдХрд╛рдо рдХрд░рддрд╛ рд╣реИ
DataItem.
, ( ), :
public class LearningAlgorithmConfig { public double LearningRate { get; set; } /// <summary> /// Size of the butch. -1 means fullbutch size. /// </summary> public int BatchSize { get; set; } public double RegularizationFactor { get; set; } public int MaxEpoches { get; set; } /// <summary> /// If cumulative error for all training examples is less then MinError, then algorithm stops /// </summary> public double MinError { get; set; } /// <summary> /// If cumulative error change for all training examples is less then MinErrorChange, then algorithm stops /// </summary> public double MinErrorChange { get; set; } /// <summary> /// Function to minimize /// </summary> public IMetrics<double> ErrorFunction { get; set; } }
DataItem.
, ( ), :
public class LearningAlgorithmConfig { public double LearningRate { get; set; } /// <summary> /// Size of the butch. -1 means fullbutch size. /// </summary> public int BatchSize { get; set; } public double RegularizationFactor { get; set; } public int MaxEpoches { get; set; } /// <summary> /// If cumulative error for all training examples is less then MinError, then algorithm stops /// </summary> public double MinError { get; set; } /// <summary> /// If cumulative error change for all training examples is less then MinErrorChange, then algorithm stops /// </summary> public double MinErrorChange { get; set; } /// <summary> /// Function to minimize /// </summary> public IMetrics<double> ErrorFunction { get; set; } }
DataItem.
, ( ), :
public class LearningAlgorithmConfig { public double LearningRate { get; set; } /// <summary> /// Size of the butch. -1 means fullbutch size. /// </summary> public int BatchSize { get; set; } public double RegularizationFactor { get; set; } public int MaxEpoches { get; set; } /// <summary> /// If cumulative error for all training examples is less then MinError, then algorithm stops /// </summary> public double MinError { get; set; } /// <summary> /// If cumulative error change for all training examples is less then MinErrorChange, then algorithm stops /// </summary> public double MinErrorChange { get; set; } /// <summary> /// Function to minimize /// </summary> public IMetrics<double> ErrorFunction { get; set; } }
рдПрд▓реНрдЧреЛрд░рд┐рдереНрдо
рдФрд░ рдЕрдВрдд рдореЗрдВ, рдкреВрд░реЗ рд╕рдВрджрд░реНрдн рдХреЛ рджрд┐рдЦрд╛рддреЗ рд╣реБрдП, рд╣рдо рддрдВрддреНрд░рд┐рдХрд╛ рдиреЗрдЯрд╡рд░реНрдХ рд▓рд░реНрдирд┐рдВрдЧ рдПрд▓реНрдЧреЛрд░рд┐рджрдо рдХреЗ рд╡рд╛рд╕реНрддрд╡рд┐рдХ рдХрд╛рд░реНрдпрд╛рдиреНрд╡рдпрди рдХреЗ рд▓рд┐рдП рдЖрдЧреЗ рдмрдврд╝ рд╕рдХрддреЗ рд╣реИрдВ
internal class BackpropagationFCNLearningAlgorithm : ILearningStrategy, public void Train(IMultilayerNeuralNetwork network, IList<DataItem> data).
( ) :
if (_config.BatchSize < 1 || _config.BatchSize > data.Count) { _config.BatchSize = data.Count; } double currentError = Single.MaxValue; double lastError = 0; int epochNumber = 0; Logger.Instance.Log("Start learning...");
, , :
do { //... } while (epochNumber < _config.MaxEpoches && currentError > _config.MinError && Math.Abs(currentError - lastError) > _config.MinErrorChange);
, , . , batch , .
lastError = currentError; DateTime dtStart = DateTime.Now; //preparation for epoche int[] trainingIndices = new int[data.Count]; for (int i = 0; i < data.Count; i++) { trainingIndices[i] = i; } if (_config.BatchSize > 0) { trainingIndices = Shuffle(trainingIndices); }
, , , :
//process data set int currentIndex = 0; do { #region initialize accumulated error for batch, for weights and biases double[][][] nablaWeights = new double[network.Layers.Length][][]; double[][] nablaBiases = new double[network.Layers.Length][]; for (int i = 0; i < network.Layers.Length; i++) { nablaBiases[i] = new double[network.Layers[i].Neurons.Length]; nablaWeights[i] = new double[network.Layers[i].Neurons.Length][]; for (int j = 0; j < network.Layers[i].Neurons.Length; j++) { nablaBiases[i][j] = 0; nablaWeights[i][j] = new double[network.Layers[i].Neurons[j].Weights.Length]; for (int k = 0; k < network.Layers[i].Neurons[j].Weights.Length; k++) { nablaWeights[i][j][k] = 0; } } } #endregion //process one batch for (int inBatchIndex = currentIndex; inBatchIndex < currentIndex + _config.BatchSize && inBatchIndex < data.Count; inBatchIndex++) { //forward pass double[] realOutput = network.ComputeOutput(data[trainingIndices[inBatchIndex]].Input); //backward pass, error propagation //last layer //....................................... //hidden layers //....................................... } //update weights and bias for (int layerIndex = 0; layerIndex < network.Layers.Length; layerIndex++) { for (int neuronIndex = 0; neuronIndex < network.Layers[layerIndex].Neurons.Length; neuronIndex++) { network.Layers[layerIndex].Neurons[neuronIndex].Bias -= nablaBiases[layerIndex][neuronIndex]; for (int weightIndex = 0; weightIndex < network.Layers[layerIndex].Neurons[neuronIndex].Weights.Length; weightIndex++) { network.Layers[layerIndex].Neurons[neuronIndex].Weights[weightIndex] -= nablaWeights[layerIndex][neuronIndex][weightIndex]; } } } currentIndex += _config.BatchSize; } while (currentIndex < data.Count);
:
"", ( , ) dE/dz
//last layer for (int j = 0; j < network.Layers[network.Layers.Length - 1].Neurons.Length; j++) { network.Layers[network.Layers.Length - 1].Neurons[j].dEdz = _config.ErrorFunction.CalculatePartialDerivaitveByV2Index(data[inBatchIndex].Output, realOutput, j) * network.Layers[network.Layers.Length - 1].Neurons[j].ActivationFunction. ComputeFirstDerivative(network.Layers[network.Layers.Length - 1].Neurons[j].LastNET); nablaBiases[network.Layers.Length - 1][j] += _config.LearningRate * network.Layers[network.Layers.Length - 1].Neurons[j].dEdz; for (int i = 0; i < network.Layers[network.Layers.Length - 1].Neurons[j].Weights.Length; i++) { nablaWeights[network.Layers.Length - 1][j][i] += _config.LearningRate*(network.Layers[network.Layers.Length - 1].Neurons[j].dEdz* (network.Layers.Length > 1 ? network.Layers[network.Layers.Length - 1 - 1].Neurons[i].LastState : data[inBatchIndex].Input[i]) + _config.RegularizationFactor * network.Layers[network.Layers.Length - 1].Neurons[j].Weights[i] / data.Count); } }
:
"", ( , ) dE/dz, ,
//hidden layers for (int hiddenLayerIndex = network.Layers.Length - 2; hiddenLayerIndex >= 0; hiddenLayerIndex--) { for (int j = 0; j < network.Layers[hiddenLayerIndex].Neurons.Length; j++) { network.Layers[hiddenLayerIndex].Neurons[j].dEdz = 0; for (int k = 0; k < network.Layers[hiddenLayerIndex + 1].Neurons.Length; k++) { network.Layers[hiddenLayerIndex].Neurons[j].dEdz += network.Layers[hiddenLayerIndex + 1].Neurons[k].Weights[j]* network.Layers[hiddenLayerIndex + 1].Neurons[k].dEdz; } network.Layers[hiddenLayerIndex].Neurons[j].dEdz *= network.Layers[hiddenLayerIndex].Neurons[j].ActivationFunction. ComputeFirstDerivative( network.Layers[hiddenLayerIndex].Neurons[j].LastNET ); nablaBiases[hiddenLayerIndex][j] += _config.LearningRate* network.Layers[hiddenLayerIndex].Neurons[j].dEdz; for (int i = 0; i < network.Layers[hiddenLayerIndex].Neurons[j].Weights.Length; i++) { nablaWeights[hiddenLayerIndex][j][i] += _config.LearningRate * ( network.Layers[hiddenLayerIndex].Neurons[j].dEdz * (hiddenLayerIndex > 0 ? network.Layers[hiddenLayerIndex - 1].Neurons[i].LastState : data[inBatchIndex].Input[i]) + _config.RegularizationFactor * network.Layers[hiddenLayerIndex].Neurons[j].Weights[i] / data.Count ); } } }
, ( ), :
//recalculating error on all data //real error currentError = 0; for (int i = 0; i < data.Count; i++) { double[] realOutput = network.ComputeOutput(data[i].Input); currentError += _config.ErrorFunction.Calculate(data[i].Output, realOutput); } currentError *= 1d/data.Count; //regularization term if (Math.Abs(_config.RegularizationFactor - 0d) > Double.Epsilon) { double reg = 0; for (int layerIndex = 0; layerIndex < network.Layers.Length; layerIndex++) { for (int neuronIndex = 0; neuronIndex < network.Layers[layerIndex].Neurons.Length; neuronIndex++) { for (int weightIndex = 0; weightIndex < network.Layers[layerIndex].Neurons[neuronIndex].Weights.Length; weightIndex++) { reg += network.Layers[layerIndex].Neurons[neuronIndex].Weights[weightIndex] * network.Layers[layerIndex].Neurons[neuronIndex].Weights[weightIndex]; } } } currentError += _config.RegularizationFactor * reg / (2 * data.Count); } epochNumber++; Logger.Instance.Log("Eposh #" + epochNumber.ToString() + " finished; current error is " + currentError.ToString() + "; it takes: " + (DateTime.Now - dtStart).Duration().ToString());
, :
} while (epochNumber < _config.MaxEpoches && currentError > _config.MinError && Math.Abs(currentError - lastError) > _config.MinErrorChange);
. , , , . . , , , , , .
:
:
.
, , , , . -)
internal class BackpropagationFCNLearningAlgorithm : ILearningStrategy, public void Train(IMultilayerNeuralNetwork network, IList<DataItem> data).
( ) :
if (_config.BatchSize < 1 || _config.BatchSize > data.Count) { _config.BatchSize = data.Count; } double currentError = Single.MaxValue; double lastError = 0; int epochNumber = 0; Logger.Instance.Log("Start learning...");
, , :
do { //... } while (epochNumber < _config.MaxEpoches && currentError > _config.MinError && Math.Abs(currentError - lastError) > _config.MinErrorChange);
, , . , batch , .
lastError = currentError; DateTime dtStart = DateTime.Now; //preparation for epoche int[] trainingIndices = new int[data.Count]; for (int i = 0; i < data.Count; i++) { trainingIndices[i] = i; } if (_config.BatchSize > 0) { trainingIndices = Shuffle(trainingIndices); }
, , , :
//process data set int currentIndex = 0; do { #region initialize accumulated error for batch, for weights and biases double[][][] nablaWeights = new double[network.Layers.Length][][]; double[][] nablaBiases = new double[network.Layers.Length][]; for (int i = 0; i < network.Layers.Length; i++) { nablaBiases[i] = new double[network.Layers[i].Neurons.Length]; nablaWeights[i] = new double[network.Layers[i].Neurons.Length][]; for (int j = 0; j < network.Layers[i].Neurons.Length; j++) { nablaBiases[i][j] = 0; nablaWeights[i][j] = new double[network.Layers[i].Neurons[j].Weights.Length]; for (int k = 0; k < network.Layers[i].Neurons[j].Weights.Length; k++) { nablaWeights[i][j][k] = 0; } } } #endregion //process one batch for (int inBatchIndex = currentIndex; inBatchIndex < currentIndex + _config.BatchSize && inBatchIndex < data.Count; inBatchIndex++) { //forward pass double[] realOutput = network.ComputeOutput(data[trainingIndices[inBatchIndex]].Input); //backward pass, error propagation //last layer //....................................... //hidden layers //....................................... } //update weights and bias for (int layerIndex = 0; layerIndex < network.Layers.Length; layerIndex++) { for (int neuronIndex = 0; neuronIndex < network.Layers[layerIndex].Neurons.Length; neuronIndex++) { network.Layers[layerIndex].Neurons[neuronIndex].Bias -= nablaBiases[layerIndex][neuronIndex]; for (int weightIndex = 0; weightIndex < network.Layers[layerIndex].Neurons[neuronIndex].Weights.Length; weightIndex++) { network.Layers[layerIndex].Neurons[neuronIndex].Weights[weightIndex] -= nablaWeights[layerIndex][neuronIndex][weightIndex]; } } } currentIndex += _config.BatchSize; } while (currentIndex < data.Count);
:
"", ( , ) dE/dz
//last layer for (int j = 0; j < network.Layers[network.Layers.Length - 1].Neurons.Length; j++) { network.Layers[network.Layers.Length - 1].Neurons[j].dEdz = _config.ErrorFunction.CalculatePartialDerivaitveByV2Index(data[inBatchIndex].Output, realOutput, j) * network.Layers[network.Layers.Length - 1].Neurons[j].ActivationFunction. ComputeFirstDerivative(network.Layers[network.Layers.Length - 1].Neurons[j].LastNET); nablaBiases[network.Layers.Length - 1][j] += _config.LearningRate * network.Layers[network.Layers.Length - 1].Neurons[j].dEdz; for (int i = 0; i < network.Layers[network.Layers.Length - 1].Neurons[j].Weights.Length; i++) { nablaWeights[network.Layers.Length - 1][j][i] += _config.LearningRate*(network.Layers[network.Layers.Length - 1].Neurons[j].dEdz* (network.Layers.Length > 1 ? network.Layers[network.Layers.Length - 1 - 1].Neurons[i].LastState : data[inBatchIndex].Input[i]) + _config.RegularizationFactor * network.Layers[network.Layers.Length - 1].Neurons[j].Weights[i] / data.Count); } }
:
"", ( , ) dE/dz, ,
//hidden layers for (int hiddenLayerIndex = network.Layers.Length - 2; hiddenLayerIndex >= 0; hiddenLayerIndex--) { for (int j = 0; j < network.Layers[hiddenLayerIndex].Neurons.Length; j++) { network.Layers[hiddenLayerIndex].Neurons[j].dEdz = 0; for (int k = 0; k < network.Layers[hiddenLayerIndex + 1].Neurons.Length; k++) { network.Layers[hiddenLayerIndex].Neurons[j].dEdz += network.Layers[hiddenLayerIndex + 1].Neurons[k].Weights[j]* network.Layers[hiddenLayerIndex + 1].Neurons[k].dEdz; } network.Layers[hiddenLayerIndex].Neurons[j].dEdz *= network.Layers[hiddenLayerIndex].Neurons[j].ActivationFunction. ComputeFirstDerivative( network.Layers[hiddenLayerIndex].Neurons[j].LastNET ); nablaBiases[hiddenLayerIndex][j] += _config.LearningRate* network.Layers[hiddenLayerIndex].Neurons[j].dEdz; for (int i = 0; i < network.Layers[hiddenLayerIndex].Neurons[j].Weights.Length; i++) { nablaWeights[hiddenLayerIndex][j][i] += _config.LearningRate * ( network.Layers[hiddenLayerIndex].Neurons[j].dEdz * (hiddenLayerIndex > 0 ? network.Layers[hiddenLayerIndex - 1].Neurons[i].LastState : data[inBatchIndex].Input[i]) + _config.RegularizationFactor * network.Layers[hiddenLayerIndex].Neurons[j].Weights[i] / data.Count ); } } }
, ( ), :
//recalculating error on all data //real error currentError = 0; for (int i = 0; i < data.Count; i++) { double[] realOutput = network.ComputeOutput(data[i].Input); currentError += _config.ErrorFunction.Calculate(data[i].Output, realOutput); } currentError *= 1d/data.Count; //regularization term if (Math.Abs(_config.RegularizationFactor - 0d) > Double.Epsilon) { double reg = 0; for (int layerIndex = 0; layerIndex < network.Layers.Length; layerIndex++) { for (int neuronIndex = 0; neuronIndex < network.Layers[layerIndex].Neurons.Length; neuronIndex++) { for (int weightIndex = 0; weightIndex < network.Layers[layerIndex].Neurons[neuronIndex].Weights.Length; weightIndex++) { reg += network.Layers[layerIndex].Neurons[neuronIndex].Weights[weightIndex] * network.Layers[layerIndex].Neurons[neuronIndex].Weights[weightIndex]; } } } currentError += _config.RegularizationFactor * reg / (2 * data.Count); } epochNumber++; Logger.Instance.Log("Eposh #" + epochNumber.ToString() + " finished; current error is " + currentError.ToString() + "; it takes: " + (DateTime.Now - dtStart).Duration().ToString());
, :
} while (epochNumber < _config.MaxEpoches && currentError > _config.MinError && Math.Abs(currentError - lastError) > _config.MinErrorChange);
. , , , . . , , , , , .
:

:
.
, , , , . -)
internal class BackpropagationFCNLearningAlgorithm : ILearningStrategy, public void Train(IMultilayerNeuralNetwork network, IList<DataItem> data).
( ) :
if (_config.BatchSize < 1 || _config.BatchSize > data.Count) { _config.BatchSize = data.Count; } double currentError = Single.MaxValue; double lastError = 0; int epochNumber = 0; Logger.Instance.Log("Start learning...");
, , :
do { //... } while (epochNumber < _config.MaxEpoches && currentError > _config.MinError && Math.Abs(currentError - lastError) > _config.MinErrorChange);
, , . , batch , .
lastError = currentError; DateTime dtStart = DateTime.Now; //preparation for epoche int[] trainingIndices = new int[data.Count]; for (int i = 0; i < data.Count; i++) { trainingIndices[i] = i; } if (_config.BatchSize > 0) { trainingIndices = Shuffle(trainingIndices); }
, , , :
//process data set int currentIndex = 0; do { #region initialize accumulated error for batch, for weights and biases double[][][] nablaWeights = new double[network.Layers.Length][][]; double[][] nablaBiases = new double[network.Layers.Length][]; for (int i = 0; i < network.Layers.Length; i++) { nablaBiases[i] = new double[network.Layers[i].Neurons.Length]; nablaWeights[i] = new double[network.Layers[i].Neurons.Length][]; for (int j = 0; j < network.Layers[i].Neurons.Length; j++) { nablaBiases[i][j] = 0; nablaWeights[i][j] = new double[network.Layers[i].Neurons[j].Weights.Length]; for (int k = 0; k < network.Layers[i].Neurons[j].Weights.Length; k++) { nablaWeights[i][j][k] = 0; } } } #endregion //process one batch for (int inBatchIndex = currentIndex; inBatchIndex < currentIndex + _config.BatchSize && inBatchIndex < data.Count; inBatchIndex++) { //forward pass double[] realOutput = network.ComputeOutput(data[trainingIndices[inBatchIndex]].Input); //backward pass, error propagation //last layer //....................................... //hidden layers //....................................... } //update weights and bias for (int layerIndex = 0; layerIndex < network.Layers.Length; layerIndex++) { for (int neuronIndex = 0; neuronIndex < network.Layers[layerIndex].Neurons.Length; neuronIndex++) { network.Layers[layerIndex].Neurons[neuronIndex].Bias -= nablaBiases[layerIndex][neuronIndex]; for (int weightIndex = 0; weightIndex < network.Layers[layerIndex].Neurons[neuronIndex].Weights.Length; weightIndex++) { network.Layers[layerIndex].Neurons[neuronIndex].Weights[weightIndex] -= nablaWeights[layerIndex][neuronIndex][weightIndex]; } } } currentIndex += _config.BatchSize; } while (currentIndex < data.Count);
:
"", ( , ) dE/dz
//last layer for (int j = 0; j < network.Layers[network.Layers.Length - 1].Neurons.Length; j++) { network.Layers[network.Layers.Length - 1].Neurons[j].dEdz = _config.ErrorFunction.CalculatePartialDerivaitveByV2Index(data[inBatchIndex].Output, realOutput, j) * network.Layers[network.Layers.Length - 1].Neurons[j].ActivationFunction. ComputeFirstDerivative(network.Layers[network.Layers.Length - 1].Neurons[j].LastNET); nablaBiases[network.Layers.Length - 1][j] += _config.LearningRate * network.Layers[network.Layers.Length - 1].Neurons[j].dEdz; for (int i = 0; i < network.Layers[network.Layers.Length - 1].Neurons[j].Weights.Length; i++) { nablaWeights[network.Layers.Length - 1][j][i] += _config.LearningRate*(network.Layers[network.Layers.Length - 1].Neurons[j].dEdz* (network.Layers.Length > 1 ? network.Layers[network.Layers.Length - 1 - 1].Neurons[i].LastState : data[inBatchIndex].Input[i]) + _config.RegularizationFactor * network.Layers[network.Layers.Length - 1].Neurons[j].Weights[i] / data.Count); } }
:
"", ( , ) dE/dz, ,
//hidden layers for (int hiddenLayerIndex = network.Layers.Length - 2; hiddenLayerIndex >= 0; hiddenLayerIndex--) { for (int j = 0; j < network.Layers[hiddenLayerIndex].Neurons.Length; j++) { network.Layers[hiddenLayerIndex].Neurons[j].dEdz = 0; for (int k = 0; k < network.Layers[hiddenLayerIndex + 1].Neurons.Length; k++) { network.Layers[hiddenLayerIndex].Neurons[j].dEdz += network.Layers[hiddenLayerIndex + 1].Neurons[k].Weights[j]* network.Layers[hiddenLayerIndex + 1].Neurons[k].dEdz; } network.Layers[hiddenLayerIndex].Neurons[j].dEdz *= network.Layers[hiddenLayerIndex].Neurons[j].ActivationFunction. ComputeFirstDerivative( network.Layers[hiddenLayerIndex].Neurons[j].LastNET ); nablaBiases[hiddenLayerIndex][j] += _config.LearningRate* network.Layers[hiddenLayerIndex].Neurons[j].dEdz; for (int i = 0; i < network.Layers[hiddenLayerIndex].Neurons[j].Weights.Length; i++) { nablaWeights[hiddenLayerIndex][j][i] += _config.LearningRate * ( network.Layers[hiddenLayerIndex].Neurons[j].dEdz * (hiddenLayerIndex > 0 ? network.Layers[hiddenLayerIndex - 1].Neurons[i].LastState : data[inBatchIndex].Input[i]) + _config.RegularizationFactor * network.Layers[hiddenLayerIndex].Neurons[j].Weights[i] / data.Count ); } } }
, ( ), :
//recalculating error on all data //real error currentError = 0; for (int i = 0; i < data.Count; i++) { double[] realOutput = network.ComputeOutput(data[i].Input); currentError += _config.ErrorFunction.Calculate(data[i].Output, realOutput); } currentError *= 1d/data.Count; //regularization term if (Math.Abs(_config.RegularizationFactor - 0d) > Double.Epsilon) { double reg = 0; for (int layerIndex = 0; layerIndex < network.Layers.Length; layerIndex++) { for (int neuronIndex = 0; neuronIndex < network.Layers[layerIndex].Neurons.Length; neuronIndex++) { for (int weightIndex = 0; weightIndex < network.Layers[layerIndex].Neurons[neuronIndex].Weights.Length; weightIndex++) { reg += network.Layers[layerIndex].Neurons[neuronIndex].Weights[weightIndex] * network.Layers[layerIndex].Neurons[neuronIndex].Weights[weightIndex]; } } } currentError += _config.RegularizationFactor * reg / (2 * data.Count); } epochNumber++; Logger.Instance.Log("Eposh #" + epochNumber.ToString() + " finished; current error is " + currentError.ToString() + "; it takes: " + (DateTime.Now - dtStart).Duration().ToString());
, :
} while (epochNumber < _config.MaxEpoches && currentError > _config.MinError && Math.Abs(currentError - lastError) > _config.MinErrorChange);
. , , , . . , , , , , .
:
:
.
, , , , . -)
internal class BackpropagationFCNLearningAlgorithm : ILearningStrategy, public void Train(IMultilayerNeuralNetwork network, IList<DataItem> data).
( ) :
if (_config.BatchSize < 1 || _config.BatchSize > data.Count) { _config.BatchSize = data.Count; } double currentError = Single.MaxValue; double lastError = 0; int epochNumber = 0; Logger.Instance.Log("Start learning...");
, , :
do { //... } while (epochNumber < _config.MaxEpoches && currentError > _config.MinError && Math.Abs(currentError - lastError) > _config.MinErrorChange);
, , . , batch , .
lastError = currentError; DateTime dtStart = DateTime.Now; //preparation for epoche int[] trainingIndices = new int[data.Count]; for (int i = 0; i < data.Count; i++) { trainingIndices[i] = i; } if (_config.BatchSize > 0) { trainingIndices = Shuffle(trainingIndices); }
, , , :
//process data set int currentIndex = 0; do { #region initialize accumulated error for batch, for weights and biases double[][][] nablaWeights = new double[network.Layers.Length][][]; double[][] nablaBiases = new double[network.Layers.Length][]; for (int i = 0; i < network.Layers.Length; i++) { nablaBiases[i] = new double[network.Layers[i].Neurons.Length]; nablaWeights[i] = new double[network.Layers[i].Neurons.Length][]; for (int j = 0; j < network.Layers[i].Neurons.Length; j++) { nablaBiases[i][j] = 0; nablaWeights[i][j] = new double[network.Layers[i].Neurons[j].Weights.Length]; for (int k = 0; k < network.Layers[i].Neurons[j].Weights.Length; k++) { nablaWeights[i][j][k] = 0; } } } #endregion //process one batch for (int inBatchIndex = currentIndex; inBatchIndex < currentIndex + _config.BatchSize && inBatchIndex < data.Count; inBatchIndex++) { //forward pass double[] realOutput = network.ComputeOutput(data[trainingIndices[inBatchIndex]].Input); //backward pass, error propagation //last layer //....................................... //hidden layers //....................................... } //update weights and bias for (int layerIndex = 0; layerIndex < network.Layers.Length; layerIndex++) { for (int neuronIndex = 0; neuronIndex < network.Layers[layerIndex].Neurons.Length; neuronIndex++) { network.Layers[layerIndex].Neurons[neuronIndex].Bias -= nablaBiases[layerIndex][neuronIndex]; for (int weightIndex = 0; weightIndex < network.Layers[layerIndex].Neurons[neuronIndex].Weights.Length; weightIndex++) { network.Layers[layerIndex].Neurons[neuronIndex].Weights[weightIndex] -= nablaWeights[layerIndex][neuronIndex][weightIndex]; } } } currentIndex += _config.BatchSize; } while (currentIndex < data.Count);
:
"", ( , ) dE/dz
//last layer for (int j = 0; j < network.Layers[network.Layers.Length - 1].Neurons.Length; j++) { network.Layers[network.Layers.Length - 1].Neurons[j].dEdz = _config.ErrorFunction.CalculatePartialDerivaitveByV2Index(data[inBatchIndex].Output, realOutput, j) * network.Layers[network.Layers.Length - 1].Neurons[j].ActivationFunction. ComputeFirstDerivative(network.Layers[network.Layers.Length - 1].Neurons[j].LastNET); nablaBiases[network.Layers.Length - 1][j] += _config.LearningRate * network.Layers[network.Layers.Length - 1].Neurons[j].dEdz; for (int i = 0; i < network.Layers[network.Layers.Length - 1].Neurons[j].Weights.Length; i++) { nablaWeights[network.Layers.Length - 1][j][i] += _config.LearningRate*(network.Layers[network.Layers.Length - 1].Neurons[j].dEdz* (network.Layers.Length > 1 ? network.Layers[network.Layers.Length - 1 - 1].Neurons[i].LastState : data[inBatchIndex].Input[i]) + _config.RegularizationFactor * network.Layers[network.Layers.Length - 1].Neurons[j].Weights[i] / data.Count); } }
:
"", ( , ) dE/dz, ,
//hidden layers for (int hiddenLayerIndex = network.Layers.Length - 2; hiddenLayerIndex >= 0; hiddenLayerIndex--) { for (int j = 0; j < network.Layers[hiddenLayerIndex].Neurons.Length; j++) { network.Layers[hiddenLayerIndex].Neurons[j].dEdz = 0; for (int k = 0; k < network.Layers[hiddenLayerIndex + 1].Neurons.Length; k++) { network.Layers[hiddenLayerIndex].Neurons[j].dEdz += network.Layers[hiddenLayerIndex + 1].Neurons[k].Weights[j]* network.Layers[hiddenLayerIndex + 1].Neurons[k].dEdz; } network.Layers[hiddenLayerIndex].Neurons[j].dEdz *= network.Layers[hiddenLayerIndex].Neurons[j].ActivationFunction. ComputeFirstDerivative( network.Layers[hiddenLayerIndex].Neurons[j].LastNET ); nablaBiases[hiddenLayerIndex][j] += _config.LearningRate* network.Layers[hiddenLayerIndex].Neurons[j].dEdz; for (int i = 0; i < network.Layers[hiddenLayerIndex].Neurons[j].Weights.Length; i++) { nablaWeights[hiddenLayerIndex][j][i] += _config.LearningRate * ( network.Layers[hiddenLayerIndex].Neurons[j].dEdz * (hiddenLayerIndex > 0 ? network.Layers[hiddenLayerIndex - 1].Neurons[i].LastState : data[inBatchIndex].Input[i]) + _config.RegularizationFactor * network.Layers[hiddenLayerIndex].Neurons[j].Weights[i] / data.Count ); } } }
, ( ), :
//recalculating error on all data //real error currentError = 0; for (int i = 0; i < data.Count; i++) { double[] realOutput = network.ComputeOutput(data[i].Input); currentError += _config.ErrorFunction.Calculate(data[i].Output, realOutput); } currentError *= 1d/data.Count; //regularization term if (Math.Abs(_config.RegularizationFactor - 0d) > Double.Epsilon) { double reg = 0; for (int layerIndex = 0; layerIndex < network.Layers.Length; layerIndex++) { for (int neuronIndex = 0; neuronIndex < network.Layers[layerIndex].Neurons.Length; neuronIndex++) { for (int weightIndex = 0; weightIndex < network.Layers[layerIndex].Neurons[neuronIndex].Weights.Length; weightIndex++) { reg += network.Layers[layerIndex].Neurons[neuronIndex].Weights[weightIndex] * network.Layers[layerIndex].Neurons[neuronIndex].Weights[weightIndex]; } } } currentError += _config.RegularizationFactor * reg / (2 * data.Count); } epochNumber++; Logger.Instance.Log("Eposh #" + epochNumber.ToString() + " finished; current error is " + currentError.ToString() + "; it takes: " + (DateTime.Now - dtStart).Duration().ToString());
, :
} while (epochNumber < _config.MaxEpoches && currentError > _config.MinError && Math.Abs(currentError - lastError) > _config.MinErrorChange);
. , , , . . , , , , , .
:

:
.
, , , , . -)
internal class BackpropagationFCNLearningAlgorithm : ILearningStrategy, public void Train(IMultilayerNeuralNetwork network, IList<DataItem> data).
( ) :
if (_config.BatchSize < 1 || _config.BatchSize > data.Count) { _config.BatchSize = data.Count; } double currentError = Single.MaxValue; double lastError = 0; int epochNumber = 0; Logger.Instance.Log("Start learning...");
, , :
do { //... } while (epochNumber < _config.MaxEpoches && currentError > _config.MinError && Math.Abs(currentError - lastError) > _config.MinErrorChange);
, , . , batch , .
lastError = currentError; DateTime dtStart = DateTime.Now; //preparation for epoche int[] trainingIndices = new int[data.Count]; for (int i = 0; i < data.Count; i++) { trainingIndices[i] = i; } if (_config.BatchSize > 0) { trainingIndices = Shuffle(trainingIndices); }
, , , :
//process data set int currentIndex = 0; do { #region initialize accumulated error for batch, for weights and biases double[][][] nablaWeights = new double[network.Layers.Length][][]; double[][] nablaBiases = new double[network.Layers.Length][]; for (int i = 0; i < network.Layers.Length; i++) { nablaBiases[i] = new double[network.Layers[i].Neurons.Length]; nablaWeights[i] = new double[network.Layers[i].Neurons.Length][]; for (int j = 0; j < network.Layers[i].Neurons.Length; j++) { nablaBiases[i][j] = 0; nablaWeights[i][j] = new double[network.Layers[i].Neurons[j].Weights.Length]; for (int k = 0; k < network.Layers[i].Neurons[j].Weights.Length; k++) { nablaWeights[i][j][k] = 0; } } } #endregion //process one batch for (int inBatchIndex = currentIndex; inBatchIndex < currentIndex + _config.BatchSize && inBatchIndex < data.Count; inBatchIndex++) { //forward pass double[] realOutput = network.ComputeOutput(data[trainingIndices[inBatchIndex]].Input); //backward pass, error propagation //last layer //....................................... //hidden layers //....................................... } //update weights and bias for (int layerIndex = 0; layerIndex < network.Layers.Length; layerIndex++) { for (int neuronIndex = 0; neuronIndex < network.Layers[layerIndex].Neurons.Length; neuronIndex++) { network.Layers[layerIndex].Neurons[neuronIndex].Bias -= nablaBiases[layerIndex][neuronIndex]; for (int weightIndex = 0; weightIndex < network.Layers[layerIndex].Neurons[neuronIndex].Weights.Length; weightIndex++) { network.Layers[layerIndex].Neurons[neuronIndex].Weights[weightIndex] -= nablaWeights[layerIndex][neuronIndex][weightIndex]; } } } currentIndex += _config.BatchSize; } while (currentIndex < data.Count);
:
"", ( , ) dE/dz
//last layer for (int j = 0; j < network.Layers[network.Layers.Length - 1].Neurons.Length; j++) { network.Layers[network.Layers.Length - 1].Neurons[j].dEdz = _config.ErrorFunction.CalculatePartialDerivaitveByV2Index(data[inBatchIndex].Output, realOutput, j) * network.Layers[network.Layers.Length - 1].Neurons[j].ActivationFunction. ComputeFirstDerivative(network.Layers[network.Layers.Length - 1].Neurons[j].LastNET); nablaBiases[network.Layers.Length - 1][j] += _config.LearningRate * network.Layers[network.Layers.Length - 1].Neurons[j].dEdz; for (int i = 0; i < network.Layers[network.Layers.Length - 1].Neurons[j].Weights.Length; i++) { nablaWeights[network.Layers.Length - 1][j][i] += _config.LearningRate*(network.Layers[network.Layers.Length - 1].Neurons[j].dEdz* (network.Layers.Length > 1 ? network.Layers[network.Layers.Length - 1 - 1].Neurons[i].LastState : data[inBatchIndex].Input[i]) + _config.RegularizationFactor * network.Layers[network.Layers.Length - 1].Neurons[j].Weights[i] / data.Count); } }
:
"", ( , ) dE/dz, ,
//hidden layers for (int hiddenLayerIndex = network.Layers.Length - 2; hiddenLayerIndex >= 0; hiddenLayerIndex--) { for (int j = 0; j < network.Layers[hiddenLayerIndex].Neurons.Length; j++) { network.Layers[hiddenLayerIndex].Neurons[j].dEdz = 0; for (int k = 0; k < network.Layers[hiddenLayerIndex + 1].Neurons.Length; k++) { network.Layers[hiddenLayerIndex].Neurons[j].dEdz += network.Layers[hiddenLayerIndex + 1].Neurons[k].Weights[j]* network.Layers[hiddenLayerIndex + 1].Neurons[k].dEdz; } network.Layers[hiddenLayerIndex].Neurons[j].dEdz *= network.Layers[hiddenLayerIndex].Neurons[j].ActivationFunction. ComputeFirstDerivative( network.Layers[hiddenLayerIndex].Neurons[j].LastNET ); nablaBiases[hiddenLayerIndex][j] += _config.LearningRate* network.Layers[hiddenLayerIndex].Neurons[j].dEdz; for (int i = 0; i < network.Layers[hiddenLayerIndex].Neurons[j].Weights.Length; i++) { nablaWeights[hiddenLayerIndex][j][i] += _config.LearningRate * ( network.Layers[hiddenLayerIndex].Neurons[j].dEdz * (hiddenLayerIndex > 0 ? network.Layers[hiddenLayerIndex - 1].Neurons[i].LastState : data[inBatchIndex].Input[i]) + _config.RegularizationFactor * network.Layers[hiddenLayerIndex].Neurons[j].Weights[i] / data.Count ); } } }
, ( ), :
//recalculating error on all data //real error currentError = 0; for (int i = 0; i < data.Count; i++) { double[] realOutput = network.ComputeOutput(data[i].Input); currentError += _config.ErrorFunction.Calculate(data[i].Output, realOutput); } currentError *= 1d/data.Count; //regularization term if (Math.Abs(_config.RegularizationFactor - 0d) > Double.Epsilon) { double reg = 0; for (int layerIndex = 0; layerIndex < network.Layers.Length; layerIndex++) { for (int neuronIndex = 0; neuronIndex < network.Layers[layerIndex].Neurons.Length; neuronIndex++) { for (int weightIndex = 0; weightIndex < network.Layers[layerIndex].Neurons[neuronIndex].Weights.Length; weightIndex++) { reg += network.Layers[layerIndex].Neurons[neuronIndex].Weights[weightIndex] * network.Layers[layerIndex].Neurons[neuronIndex].Weights[weightIndex]; } } } currentError += _config.RegularizationFactor * reg / (2 * data.Count); } epochNumber++; Logger.Instance.Log("Eposh #" + epochNumber.ToString() + " finished; current error is " + currentError.ToString() + "; it takes: " + (DateTime.Now - dtStart).Duration().ToString());
, :
} while (epochNumber < _config.MaxEpoches && currentError > _config.MinError && Math.Abs(currentError - lastError) > _config.MinErrorChange);
. , , , . . , , , , , .
:
:
.
, , , , . -)
internal class BackpropagationFCNLearningAlgorithm : ILearningStrategy, public void Train(IMultilayerNeuralNetwork network, IList<DataItem> data).
( ) :
if (_config.BatchSize < 1 || _config.BatchSize > data.Count) { _config.BatchSize = data.Count; } double currentError = Single.MaxValue; double lastError = 0; int epochNumber = 0; Logger.Instance.Log("Start learning...");
, , :
do { //... } while (epochNumber < _config.MaxEpoches && currentError > _config.MinError && Math.Abs(currentError - lastError) > _config.MinErrorChange);
, , . , batch , .
lastError = currentError; DateTime dtStart = DateTime.Now; //preparation for epoche int[] trainingIndices = new int[data.Count]; for (int i = 0; i < data.Count; i++) { trainingIndices[i] = i; } if (_config.BatchSize > 0) { trainingIndices = Shuffle(trainingIndices); }
, , , :
//process data set int currentIndex = 0; do { #region initialize accumulated error for batch, for weights and biases double[][][] nablaWeights = new double[network.Layers.Length][][]; double[][] nablaBiases = new double[network.Layers.Length][]; for (int i = 0; i < network.Layers.Length; i++) { nablaBiases[i] = new double[network.Layers[i].Neurons.Length]; nablaWeights[i] = new double[network.Layers[i].Neurons.Length][]; for (int j = 0; j < network.Layers[i].Neurons.Length; j++) { nablaBiases[i][j] = 0; nablaWeights[i][j] = new double[network.Layers[i].Neurons[j].Weights.Length]; for (int k = 0; k < network.Layers[i].Neurons[j].Weights.Length; k++) { nablaWeights[i][j][k] = 0; } } } #endregion //process one batch for (int inBatchIndex = currentIndex; inBatchIndex < currentIndex + _config.BatchSize && inBatchIndex < data.Count; inBatchIndex++) { //forward pass double[] realOutput = network.ComputeOutput(data[trainingIndices[inBatchIndex]].Input); //backward pass, error propagation //last layer //....................................... //hidden layers //....................................... } //update weights and bias for (int layerIndex = 0; layerIndex < network.Layers.Length; layerIndex++) { for (int neuronIndex = 0; neuronIndex < network.Layers[layerIndex].Neurons.Length; neuronIndex++) { network.Layers[layerIndex].Neurons[neuronIndex].Bias -= nablaBiases[layerIndex][neuronIndex]; for (int weightIndex = 0; weightIndex < network.Layers[layerIndex].Neurons[neuronIndex].Weights.Length; weightIndex++) { network.Layers[layerIndex].Neurons[neuronIndex].Weights[weightIndex] -= nablaWeights[layerIndex][neuronIndex][weightIndex]; } } } currentIndex += _config.BatchSize; } while (currentIndex < data.Count);
:
"", ( , ) dE/dz
//last layer for (int j = 0; j < network.Layers[network.Layers.Length - 1].Neurons.Length; j++) { network.Layers[network.Layers.Length - 1].Neurons[j].dEdz = _config.ErrorFunction.CalculatePartialDerivaitveByV2Index(data[inBatchIndex].Output, realOutput, j) * network.Layers[network.Layers.Length - 1].Neurons[j].ActivationFunction. ComputeFirstDerivative(network.Layers[network.Layers.Length - 1].Neurons[j].LastNET); nablaBiases[network.Layers.Length - 1][j] += _config.LearningRate * network.Layers[network.Layers.Length - 1].Neurons[j].dEdz; for (int i = 0; i < network.Layers[network.Layers.Length - 1].Neurons[j].Weights.Length; i++) { nablaWeights[network.Layers.Length - 1][j][i] += _config.LearningRate*(network.Layers[network.Layers.Length - 1].Neurons[j].dEdz* (network.Layers.Length > 1 ? network.Layers[network.Layers.Length - 1 - 1].Neurons[i].LastState : data[inBatchIndex].Input[i]) + _config.RegularizationFactor * network.Layers[network.Layers.Length - 1].Neurons[j].Weights[i] / data.Count); } }
:
"", ( , ) dE/dz, ,
//hidden layers for (int hiddenLayerIndex = network.Layers.Length - 2; hiddenLayerIndex >= 0; hiddenLayerIndex--) { for (int j = 0; j < network.Layers[hiddenLayerIndex].Neurons.Length; j++) { network.Layers[hiddenLayerIndex].Neurons[j].dEdz = 0; for (int k = 0; k < network.Layers[hiddenLayerIndex + 1].Neurons.Length; k++) { network.Layers[hiddenLayerIndex].Neurons[j].dEdz += network.Layers[hiddenLayerIndex + 1].Neurons[k].Weights[j]* network.Layers[hiddenLayerIndex + 1].Neurons[k].dEdz; } network.Layers[hiddenLayerIndex].Neurons[j].dEdz *= network.Layers[hiddenLayerIndex].Neurons[j].ActivationFunction. ComputeFirstDerivative( network.Layers[hiddenLayerIndex].Neurons[j].LastNET ); nablaBiases[hiddenLayerIndex][j] += _config.LearningRate* network.Layers[hiddenLayerIndex].Neurons[j].dEdz; for (int i = 0; i < network.Layers[hiddenLayerIndex].Neurons[j].Weights.Length; i++) { nablaWeights[hiddenLayerIndex][j][i] += _config.LearningRate * ( network.Layers[hiddenLayerIndex].Neurons[j].dEdz * (hiddenLayerIndex > 0 ? network.Layers[hiddenLayerIndex - 1].Neurons[i].LastState : data[inBatchIndex].Input[i]) + _config.RegularizationFactor * network.Layers[hiddenLayerIndex].Neurons[j].Weights[i] / data.Count ); } } }
, ( ), :
//recalculating error on all data //real error currentError = 0; for (int i = 0; i < data.Count; i++) { double[] realOutput = network.ComputeOutput(data[i].Input); currentError += _config.ErrorFunction.Calculate(data[i].Output, realOutput); } currentError *= 1d/data.Count; //regularization term if (Math.Abs(_config.RegularizationFactor - 0d) > Double.Epsilon) { double reg = 0; for (int layerIndex = 0; layerIndex < network.Layers.Length; layerIndex++) { for (int neuronIndex = 0; neuronIndex < network.Layers[layerIndex].Neurons.Length; neuronIndex++) { for (int weightIndex = 0; weightIndex < network.Layers[layerIndex].Neurons[neuronIndex].Weights.Length; weightIndex++) { reg += network.Layers[layerIndex].Neurons[neuronIndex].Weights[weightIndex] * network.Layers[layerIndex].Neurons[neuronIndex].Weights[weightIndex]; } } } currentError += _config.RegularizationFactor * reg / (2 * data.Count); } epochNumber++; Logger.Instance.Log("Eposh #" + epochNumber.ToString() + " finished; current error is " + currentError.ToString() + "; it takes: " + (DateTime.Now - dtStart).Duration().ToString());
, :
} while (epochNumber < _config.MaxEpoches && currentError > _config.MinError && Math.Abs(currentError - lastError) > _config.MinErrorChange);
. , , , . . , , , , , .
:

:
.
, , , , . -)
internal class BackpropagationFCNLearningAlgorithm : ILearningStrategy, public void Train(IMultilayerNeuralNetwork network, IList<DataItem> data).
( ) :
if (_config.BatchSize < 1 || _config.BatchSize > data.Count) { _config.BatchSize = data.Count; } double currentError = Single.MaxValue; double lastError = 0; int epochNumber = 0; Logger.Instance.Log("Start learning...");
, , :
do { //... } while (epochNumber < _config.MaxEpoches && currentError > _config.MinError && Math.Abs(currentError - lastError) > _config.MinErrorChange);
, , . , batch , .
lastError = currentError; DateTime dtStart = DateTime.Now; //preparation for epoche int[] trainingIndices = new int[data.Count]; for (int i = 0; i < data.Count; i++) { trainingIndices[i] = i; } if (_config.BatchSize > 0) { trainingIndices = Shuffle(trainingIndices); }
, , , :
//process data set int currentIndex = 0; do { #region initialize accumulated error for batch, for weights and biases double[][][] nablaWeights = new double[network.Layers.Length][][]; double[][] nablaBiases = new double[network.Layers.Length][]; for (int i = 0; i < network.Layers.Length; i++) { nablaBiases[i] = new double[network.Layers[i].Neurons.Length]; nablaWeights[i] = new double[network.Layers[i].Neurons.Length][]; for (int j = 0; j < network.Layers[i].Neurons.Length; j++) { nablaBiases[i][j] = 0; nablaWeights[i][j] = new double[network.Layers[i].Neurons[j].Weights.Length]; for (int k = 0; k < network.Layers[i].Neurons[j].Weights.Length; k++) { nablaWeights[i][j][k] = 0; } } } #endregion //process one batch for (int inBatchIndex = currentIndex; inBatchIndex < currentIndex + _config.BatchSize && inBatchIndex < data.Count; inBatchIndex++) { //forward pass double[] realOutput = network.ComputeOutput(data[trainingIndices[inBatchIndex]].Input); //backward pass, error propagation //last layer //....................................... //hidden layers //....................................... } //update weights and bias for (int layerIndex = 0; layerIndex < network.Layers.Length; layerIndex++) { for (int neuronIndex = 0; neuronIndex < network.Layers[layerIndex].Neurons.Length; neuronIndex++) { network.Layers[layerIndex].Neurons[neuronIndex].Bias -= nablaBiases[layerIndex][neuronIndex]; for (int weightIndex = 0; weightIndex < network.Layers[layerIndex].Neurons[neuronIndex].Weights.Length; weightIndex++) { network.Layers[layerIndex].Neurons[neuronIndex].Weights[weightIndex] -= nablaWeights[layerIndex][neuronIndex][weightIndex]; } } } currentIndex += _config.BatchSize; } while (currentIndex < data.Count);
:
"", ( , ) dE/dz
//last layer for (int j = 0; j < network.Layers[network.Layers.Length - 1].Neurons.Length; j++) { network.Layers[network.Layers.Length - 1].Neurons[j].dEdz = _config.ErrorFunction.CalculatePartialDerivaitveByV2Index(data[inBatchIndex].Output, realOutput, j) * network.Layers[network.Layers.Length - 1].Neurons[j].ActivationFunction. ComputeFirstDerivative(network.Layers[network.Layers.Length - 1].Neurons[j].LastNET); nablaBiases[network.Layers.Length - 1][j] += _config.LearningRate * network.Layers[network.Layers.Length - 1].Neurons[j].dEdz; for (int i = 0; i < network.Layers[network.Layers.Length - 1].Neurons[j].Weights.Length; i++) { nablaWeights[network.Layers.Length - 1][j][i] += _config.LearningRate*(network.Layers[network.Layers.Length - 1].Neurons[j].dEdz* (network.Layers.Length > 1 ? network.Layers[network.Layers.Length - 1 - 1].Neurons[i].LastState : data[inBatchIndex].Input[i]) + _config.RegularizationFactor * network.Layers[network.Layers.Length - 1].Neurons[j].Weights[i] / data.Count); } }
:
"", ( , ) dE/dz, ,
//hidden layers for (int hiddenLayerIndex = network.Layers.Length - 2; hiddenLayerIndex >= 0; hiddenLayerIndex--) { for (int j = 0; j < network.Layers[hiddenLayerIndex].Neurons.Length; j++) { network.Layers[hiddenLayerIndex].Neurons[j].dEdz = 0; for (int k = 0; k < network.Layers[hiddenLayerIndex + 1].Neurons.Length; k++) { network.Layers[hiddenLayerIndex].Neurons[j].dEdz += network.Layers[hiddenLayerIndex + 1].Neurons[k].Weights[j]* network.Layers[hiddenLayerIndex + 1].Neurons[k].dEdz; } network.Layers[hiddenLayerIndex].Neurons[j].dEdz *= network.Layers[hiddenLayerIndex].Neurons[j].ActivationFunction. ComputeFirstDerivative( network.Layers[hiddenLayerIndex].Neurons[j].LastNET ); nablaBiases[hiddenLayerIndex][j] += _config.LearningRate* network.Layers[hiddenLayerIndex].Neurons[j].dEdz; for (int i = 0; i < network.Layers[hiddenLayerIndex].Neurons[j].Weights.Length; i++) { nablaWeights[hiddenLayerIndex][j][i] += _config.LearningRate * ( network.Layers[hiddenLayerIndex].Neurons[j].dEdz * (hiddenLayerIndex > 0 ? network.Layers[hiddenLayerIndex - 1].Neurons[i].LastState : data[inBatchIndex].Input[i]) + _config.RegularizationFactor * network.Layers[hiddenLayerIndex].Neurons[j].Weights[i] / data.Count ); } } }
, ( ), :
//recalculating error on all data //real error currentError = 0; for (int i = 0; i < data.Count; i++) { double[] realOutput = network.ComputeOutput(data[i].Input); currentError += _config.ErrorFunction.Calculate(data[i].Output, realOutput); } currentError *= 1d/data.Count; //regularization term if (Math.Abs(_config.RegularizationFactor - 0d) > Double.Epsilon) { double reg = 0; for (int layerIndex = 0; layerIndex < network.Layers.Length; layerIndex++) { for (int neuronIndex = 0; neuronIndex < network.Layers[layerIndex].Neurons.Length; neuronIndex++) { for (int weightIndex = 0; weightIndex < network.Layers[layerIndex].Neurons[neuronIndex].Weights.Length; weightIndex++) { reg += network.Layers[layerIndex].Neurons[neuronIndex].Weights[weightIndex] * network.Layers[layerIndex].Neurons[neuronIndex].Weights[weightIndex]; } } } currentError += _config.RegularizationFactor * reg / (2 * data.Count); } epochNumber++; Logger.Instance.Log("Eposh #" + epochNumber.ToString() + " finished; current error is " + currentError.ToString() + "; it takes: " + (DateTime.Now - dtStart).Duration().ToString());
, :
} while (epochNumber < _config.MaxEpoches && currentError > _config.MinError && Math.Abs(currentError - lastError) > _config.MinErrorChange);
. , , , . . , , , , , .
:
:
.
, , , , . -)
internal class BackpropagationFCNLearningAlgorithm : ILearningStrategy, public void Train(IMultilayerNeuralNetwork network, IList<DataItem> data).
( ) :
if (_config.BatchSize < 1 || _config.BatchSize > data.Count) { _config.BatchSize = data.Count; } double currentError = Single.MaxValue; double lastError = 0; int epochNumber = 0; Logger.Instance.Log("Start learning...");
, , :
do { //... } while (epochNumber < _config.MaxEpoches && currentError > _config.MinError && Math.Abs(currentError - lastError) > _config.MinErrorChange);
, , . , batch , .
lastError = currentError; DateTime dtStart = DateTime.Now; //preparation for epoche int[] trainingIndices = new int[data.Count]; for (int i = 0; i < data.Count; i++) { trainingIndices[i] = i; } if (_config.BatchSize > 0) { trainingIndices = Shuffle(trainingIndices); }
, , , :
//process data set int currentIndex = 0; do { #region initialize accumulated error for batch, for weights and biases double[][][] nablaWeights = new double[network.Layers.Length][][]; double[][] nablaBiases = new double[network.Layers.Length][]; for (int i = 0; i < network.Layers.Length; i++) { nablaBiases[i] = new double[network.Layers[i].Neurons.Length]; nablaWeights[i] = new double[network.Layers[i].Neurons.Length][]; for (int j = 0; j < network.Layers[i].Neurons.Length; j++) { nablaBiases[i][j] = 0; nablaWeights[i][j] = new double[network.Layers[i].Neurons[j].Weights.Length]; for (int k = 0; k < network.Layers[i].Neurons[j].Weights.Length; k++) { nablaWeights[i][j][k] = 0; } } } #endregion //process one batch for (int inBatchIndex = currentIndex; inBatchIndex < currentIndex + _config.BatchSize && inBatchIndex < data.Count; inBatchIndex++) { //forward pass double[] realOutput = network.ComputeOutput(data[trainingIndices[inBatchIndex]].Input); //backward pass, error propagation //last layer //....................................... //hidden layers //....................................... } //update weights and bias for (int layerIndex = 0; layerIndex < network.Layers.Length; layerIndex++) { for (int neuronIndex = 0; neuronIndex < network.Layers[layerIndex].Neurons.Length; neuronIndex++) { network.Layers[layerIndex].Neurons[neuronIndex].Bias -= nablaBiases[layerIndex][neuronIndex]; for (int weightIndex = 0; weightIndex < network.Layers[layerIndex].Neurons[neuronIndex].Weights.Length; weightIndex++) { network.Layers[layerIndex].Neurons[neuronIndex].Weights[weightIndex] -= nablaWeights[layerIndex][neuronIndex][weightIndex]; } } } currentIndex += _config.BatchSize; } while (currentIndex < data.Count);
:
"", ( , ) dE/dz
//last layer for (int j = 0; j < network.Layers[network.Layers.Length - 1].Neurons.Length; j++) { network.Layers[network.Layers.Length - 1].Neurons[j].dEdz = _config.ErrorFunction.CalculatePartialDerivaitveByV2Index(data[inBatchIndex].Output, realOutput, j) * network.Layers[network.Layers.Length - 1].Neurons[j].ActivationFunction. ComputeFirstDerivative(network.Layers[network.Layers.Length - 1].Neurons[j].LastNET); nablaBiases[network.Layers.Length - 1][j] += _config.LearningRate * network.Layers[network.Layers.Length - 1].Neurons[j].dEdz; for (int i = 0; i < network.Layers[network.Layers.Length - 1].Neurons[j].Weights.Length; i++) { nablaWeights[network.Layers.Length - 1][j][i] += _config.LearningRate*(network.Layers[network.Layers.Length - 1].Neurons[j].dEdz* (network.Layers.Length > 1 ? network.Layers[network.Layers.Length - 1 - 1].Neurons[i].LastState : data[inBatchIndex].Input[i]) + _config.RegularizationFactor * network.Layers[network.Layers.Length - 1].Neurons[j].Weights[i] / data.Count); } }
:
"", ( , ) dE/dz, ,
//hidden layers for (int hiddenLayerIndex = network.Layers.Length - 2; hiddenLayerIndex >= 0; hiddenLayerIndex--) { for (int j = 0; j < network.Layers[hiddenLayerIndex].Neurons.Length; j++) { network.Layers[hiddenLayerIndex].Neurons[j].dEdz = 0; for (int k = 0; k < network.Layers[hiddenLayerIndex + 1].Neurons.Length; k++) { network.Layers[hiddenLayerIndex].Neurons[j].dEdz += network.Layers[hiddenLayerIndex + 1].Neurons[k].Weights[j]* network.Layers[hiddenLayerIndex + 1].Neurons[k].dEdz; } network.Layers[hiddenLayerIndex].Neurons[j].dEdz *= network.Layers[hiddenLayerIndex].Neurons[j].ActivationFunction. ComputeFirstDerivative( network.Layers[hiddenLayerIndex].Neurons[j].LastNET ); nablaBiases[hiddenLayerIndex][j] += _config.LearningRate* network.Layers[hiddenLayerIndex].Neurons[j].dEdz; for (int i = 0; i < network.Layers[hiddenLayerIndex].Neurons[j].Weights.Length; i++) { nablaWeights[hiddenLayerIndex][j][i] += _config.LearningRate * ( network.Layers[hiddenLayerIndex].Neurons[j].dEdz * (hiddenLayerIndex > 0 ? network.Layers[hiddenLayerIndex - 1].Neurons[i].LastState : data[inBatchIndex].Input[i]) + _config.RegularizationFactor * network.Layers[hiddenLayerIndex].Neurons[j].Weights[i] / data.Count ); } } }
, ( ), :
//recalculating error on all data //real error currentError = 0; for (int i = 0; i < data.Count; i++) { double[] realOutput = network.ComputeOutput(data[i].Input); currentError += _config.ErrorFunction.Calculate(data[i].Output, realOutput); } currentError *= 1d/data.Count; //regularization term if (Math.Abs(_config.RegularizationFactor - 0d) > Double.Epsilon) { double reg = 0; for (int layerIndex = 0; layerIndex < network.Layers.Length; layerIndex++) { for (int neuronIndex = 0; neuronIndex < network.Layers[layerIndex].Neurons.Length; neuronIndex++) { for (int weightIndex = 0; weightIndex < network.Layers[layerIndex].Neurons[neuronIndex].Weights.Length; weightIndex++) { reg += network.Layers[layerIndex].Neurons[neuronIndex].Weights[weightIndex] * network.Layers[layerIndex].Neurons[neuronIndex].Weights[weightIndex]; } } } currentError += _config.RegularizationFactor * reg / (2 * data.Count); } epochNumber++; Logger.Instance.Log("Eposh #" + epochNumber.ToString() + " finished; current error is " + currentError.ToString() + "; it takes: " + (DateTime.Now - dtStart).Duration().ToString());
, :
} while (epochNumber < _config.MaxEpoches && currentError > _config.MinError && Math.Abs(currentError - lastError) > _config.MinErrorChange);
. , , , . . , , , , , .
:

:
.
, , , , . -)
internal class BackpropagationFCNLearningAlgorithm : ILearningStrategy, public void Train(IMultilayerNeuralNetwork network, IList<DataItem> data).
( ) :
if (_config.BatchSize < 1 || _config.BatchSize > data.Count) { _config.BatchSize = data.Count; } double currentError = Single.MaxValue; double lastError = 0; int epochNumber = 0; Logger.Instance.Log("Start learning...");
, , :
do { //... } while (epochNumber < _config.MaxEpoches && currentError > _config.MinError && Math.Abs(currentError - lastError) > _config.MinErrorChange);
, , . , batch , .
lastError = currentError; DateTime dtStart = DateTime.Now; //preparation for epoche int[] trainingIndices = new int[data.Count]; for (int i = 0; i < data.Count; i++) { trainingIndices[i] = i; } if (_config.BatchSize > 0) { trainingIndices = Shuffle(trainingIndices); }
, , , :
//process data set int currentIndex = 0; do { #region initialize accumulated error for batch, for weights and biases double[][][] nablaWeights = new double[network.Layers.Length][][]; double[][] nablaBiases = new double[network.Layers.Length][]; for (int i = 0; i < network.Layers.Length; i++) { nablaBiases[i] = new double[network.Layers[i].Neurons.Length]; nablaWeights[i] = new double[network.Layers[i].Neurons.Length][]; for (int j = 0; j < network.Layers[i].Neurons.Length; j++) { nablaBiases[i][j] = 0; nablaWeights[i][j] = new double[network.Layers[i].Neurons[j].Weights.Length]; for (int k = 0; k < network.Layers[i].Neurons[j].Weights.Length; k++) { nablaWeights[i][j][k] = 0; } } } #endregion //process one batch for (int inBatchIndex = currentIndex; inBatchIndex < currentIndex + _config.BatchSize && inBatchIndex < data.Count; inBatchIndex++) { //forward pass double[] realOutput = network.ComputeOutput(data[trainingIndices[inBatchIndex]].Input); //backward pass, error propagation //last layer //....................................... //hidden layers //....................................... } //update weights and bias for (int layerIndex = 0; layerIndex < network.Layers.Length; layerIndex++) { for (int neuronIndex = 0; neuronIndex < network.Layers[layerIndex].Neurons.Length; neuronIndex++) { network.Layers[layerIndex].Neurons[neuronIndex].Bias -= nablaBiases[layerIndex][neuronIndex]; for (int weightIndex = 0; weightIndex < network.Layers[layerIndex].Neurons[neuronIndex].Weights.Length; weightIndex++) { network.Layers[layerIndex].Neurons[neuronIndex].Weights[weightIndex] -= nablaWeights[layerIndex][neuronIndex][weightIndex]; } } } currentIndex += _config.BatchSize; } while (currentIndex < data.Count);
:
"", ( , ) dE/dz
//last layer for (int j = 0; j < network.Layers[network.Layers.Length - 1].Neurons.Length; j++) { network.Layers[network.Layers.Length - 1].Neurons[j].dEdz = _config.ErrorFunction.CalculatePartialDerivaitveByV2Index(data[inBatchIndex].Output, realOutput, j) * network.Layers[network.Layers.Length - 1].Neurons[j].ActivationFunction. ComputeFirstDerivative(network.Layers[network.Layers.Length - 1].Neurons[j].LastNET); nablaBiases[network.Layers.Length - 1][j] += _config.LearningRate * network.Layers[network.Layers.Length - 1].Neurons[j].dEdz; for (int i = 0; i < network.Layers[network.Layers.Length - 1].Neurons[j].Weights.Length; i++) { nablaWeights[network.Layers.Length - 1][j][i] += _config.LearningRate*(network.Layers[network.Layers.Length - 1].Neurons[j].dEdz* (network.Layers.Length > 1 ? network.Layers[network.Layers.Length - 1 - 1].Neurons[i].LastState : data[inBatchIndex].Input[i]) + _config.RegularizationFactor * network.Layers[network.Layers.Length - 1].Neurons[j].Weights[i] / data.Count); } }
:
"", ( , ) dE/dz, ,
//hidden layers for (int hiddenLayerIndex = network.Layers.Length - 2; hiddenLayerIndex >= 0; hiddenLayerIndex--) { for (int j = 0; j < network.Layers[hiddenLayerIndex].Neurons.Length; j++) { network.Layers[hiddenLayerIndex].Neurons[j].dEdz = 0; for (int k = 0; k < network.Layers[hiddenLayerIndex + 1].Neurons.Length; k++) { network.Layers[hiddenLayerIndex].Neurons[j].dEdz += network.Layers[hiddenLayerIndex + 1].Neurons[k].Weights[j]* network.Layers[hiddenLayerIndex + 1].Neurons[k].dEdz; } network.Layers[hiddenLayerIndex].Neurons[j].dEdz *= network.Layers[hiddenLayerIndex].Neurons[j].ActivationFunction. ComputeFirstDerivative( network.Layers[hiddenLayerIndex].Neurons[j].LastNET ); nablaBiases[hiddenLayerIndex][j] += _config.LearningRate* network.Layers[hiddenLayerIndex].Neurons[j].dEdz; for (int i = 0; i < network.Layers[hiddenLayerIndex].Neurons[j].Weights.Length; i++) { nablaWeights[hiddenLayerIndex][j][i] += _config.LearningRate * ( network.Layers[hiddenLayerIndex].Neurons[j].dEdz * (hiddenLayerIndex > 0 ? network.Layers[hiddenLayerIndex - 1].Neurons[i].LastState : data[inBatchIndex].Input[i]) + _config.RegularizationFactor * network.Layers[hiddenLayerIndex].Neurons[j].Weights[i] / data.Count ); } } }
, ( ), :
//recalculating error on all data //real error currentError = 0; for (int i = 0; i < data.Count; i++) { double[] realOutput = network.ComputeOutput(data[i].Input); currentError += _config.ErrorFunction.Calculate(data[i].Output, realOutput); } currentError *= 1d/data.Count; //regularization term if (Math.Abs(_config.RegularizationFactor - 0d) > Double.Epsilon) { double reg = 0; for (int layerIndex = 0; layerIndex < network.Layers.Length; layerIndex++) { for (int neuronIndex = 0; neuronIndex < network.Layers[layerIndex].Neurons.Length; neuronIndex++) { for (int weightIndex = 0; weightIndex < network.Layers[layerIndex].Neurons[neuronIndex].Weights.Length; weightIndex++) { reg += network.Layers[layerIndex].Neurons[neuronIndex].Weights[weightIndex] * network.Layers[layerIndex].Neurons[neuronIndex].Weights[weightIndex]; } } } currentError += _config.RegularizationFactor * reg / (2 * data.Count); } epochNumber++; Logger.Instance.Log("Eposh #" + epochNumber.ToString() + " finished; current error is " + currentError.ToString() + "; it takes: " + (DateTime.Now - dtStart).Duration().ToString());
, :
} while (epochNumber < _config.MaxEpoches && currentError > _config.MinError && Math.Abs(currentError - lastError) > _config.MinErrorChange);
. , , , . . , , , , , .
:

:
.
, , , , . -)
internal class BackpropagationFCNLearningAlgorithm : ILearningStrategy, public void Train(IMultilayerNeuralNetwork network, IList<DataItem> data).
( ) :
if (_config.BatchSize < 1 || _config.BatchSize > data.Count) { _config.BatchSize = data.Count; } double currentError = Single.MaxValue; double lastError = 0; int epochNumber = 0; Logger.Instance.Log("Start learning...");
, , :
do { //... } while (epochNumber < _config.MaxEpoches && currentError > _config.MinError && Math.Abs(currentError - lastError) > _config.MinErrorChange);
, , . , batch , .
lastError = currentError; DateTime dtStart = DateTime.Now; //preparation for epoche int[] trainingIndices = new int[data.Count]; for (int i = 0; i < data.Count; i++) { trainingIndices[i] = i; } if (_config.BatchSize > 0) { trainingIndices = Shuffle(trainingIndices); }
, , , :
//process data set int currentIndex = 0; do { #region initialize accumulated error for batch, for weights and biases double[][][] nablaWeights = new double[network.Layers.Length][][]; double[][] nablaBiases = new double[network.Layers.Length][]; for (int i = 0; i < network.Layers.Length; i++) { nablaBiases[i] = new double[network.Layers[i].Neurons.Length]; nablaWeights[i] = new double[network.Layers[i].Neurons.Length][]; for (int j = 0; j < network.Layers[i].Neurons.Length; j++) { nablaBiases[i][j] = 0; nablaWeights[i][j] = new double[network.Layers[i].Neurons[j].Weights.Length]; for (int k = 0; k < network.Layers[i].Neurons[j].Weights.Length; k++) { nablaWeights[i][j][k] = 0; } } } #endregion //process one batch for (int inBatchIndex = currentIndex; inBatchIndex < currentIndex + _config.BatchSize && inBatchIndex < data.Count; inBatchIndex++) { //forward pass double[] realOutput = network.ComputeOutput(data[trainingIndices[inBatchIndex]].Input); //backward pass, error propagation //last layer //....................................... //hidden layers //....................................... } //update weights and bias for (int layerIndex = 0; layerIndex < network.Layers.Length; layerIndex++) { for (int neuronIndex = 0; neuronIndex < network.Layers[layerIndex].Neurons.Length; neuronIndex++) { network.Layers[layerIndex].Neurons[neuronIndex].Bias -= nablaBiases[layerIndex][neuronIndex]; for (int weightIndex = 0; weightIndex < network.Layers[layerIndex].Neurons[neuronIndex].Weights.Length; weightIndex++) { network.Layers[layerIndex].Neurons[neuronIndex].Weights[weightIndex] -= nablaWeights[layerIndex][neuronIndex][weightIndex]; } } } currentIndex += _config.BatchSize; } while (currentIndex < data.Count);
:
"", ( , ) dE/dz
//last layer for (int j = 0; j < network.Layers[network.Layers.Length - 1].Neurons.Length; j++) { network.Layers[network.Layers.Length - 1].Neurons[j].dEdz = _config.ErrorFunction.CalculatePartialDerivaitveByV2Index(data[inBatchIndex].Output, realOutput, j) * network.Layers[network.Layers.Length - 1].Neurons[j].ActivationFunction. ComputeFirstDerivative(network.Layers[network.Layers.Length - 1].Neurons[j].LastNET); nablaBiases[network.Layers.Length - 1][j] += _config.LearningRate * network.Layers[network.Layers.Length - 1].Neurons[j].dEdz; for (int i = 0; i < network.Layers[network.Layers.Length - 1].Neurons[j].Weights.Length; i++) { nablaWeights[network.Layers.Length - 1][j][i] += _config.LearningRate*(network.Layers[network.Layers.Length - 1].Neurons[j].dEdz* (network.Layers.Length > 1 ? network.Layers[network.Layers.Length - 1 - 1].Neurons[i].LastState : data[inBatchIndex].Input[i]) + _config.RegularizationFactor * network.Layers[network.Layers.Length - 1].Neurons[j].Weights[i] / data.Count); } }
:
"", ( , ) dE/dz, ,
//hidden layers for (int hiddenLayerIndex = network.Layers.Length - 2; hiddenLayerIndex >= 0; hiddenLayerIndex--) { for (int j = 0; j < network.Layers[hiddenLayerIndex].Neurons.Length; j++) { network.Layers[hiddenLayerIndex].Neurons[j].dEdz = 0; for (int k = 0; k < network.Layers[hiddenLayerIndex + 1].Neurons.Length; k++) { network.Layers[hiddenLayerIndex].Neurons[j].dEdz += network.Layers[hiddenLayerIndex + 1].Neurons[k].Weights[j]* network.Layers[hiddenLayerIndex + 1].Neurons[k].dEdz; } network.Layers[hiddenLayerIndex].Neurons[j].dEdz *= network.Layers[hiddenLayerIndex].Neurons[j].ActivationFunction. ComputeFirstDerivative( network.Layers[hiddenLayerIndex].Neurons[j].LastNET ); nablaBiases[hiddenLayerIndex][j] += _config.LearningRate* network.Layers[hiddenLayerIndex].Neurons[j].dEdz; for (int i = 0; i < network.Layers[hiddenLayerIndex].Neurons[j].Weights.Length; i++) { nablaWeights[hiddenLayerIndex][j][i] += _config.LearningRate * ( network.Layers[hiddenLayerIndex].Neurons[j].dEdz * (hiddenLayerIndex > 0 ? network.Layers[hiddenLayerIndex - 1].Neurons[i].LastState : data[inBatchIndex].Input[i]) + _config.RegularizationFactor * network.Layers[hiddenLayerIndex].Neurons[j].Weights[i] / data.Count ); } } }
, ( ), :
//recalculating error on all data //real error currentError = 0; for (int i = 0; i < data.Count; i++) { double[] realOutput = network.ComputeOutput(data[i].Input); currentError += _config.ErrorFunction.Calculate(data[i].Output, realOutput); } currentError *= 1d/data.Count; //regularization term if (Math.Abs(_config.RegularizationFactor - 0d) > Double.Epsilon) { double reg = 0; for (int layerIndex = 0; layerIndex < network.Layers.Length; layerIndex++) { for (int neuronIndex = 0; neuronIndex < network.Layers[layerIndex].Neurons.Length; neuronIndex++) { for (int weightIndex = 0; weightIndex < network.Layers[layerIndex].Neurons[neuronIndex].Weights.Length; weightIndex++) { reg += network.Layers[layerIndex].Neurons[neuronIndex].Weights[weightIndex] * network.Layers[layerIndex].Neurons[neuronIndex].Weights[weightIndex]; } } } currentError += _config.RegularizationFactor * reg / (2 * data.Count); } epochNumber++; Logger.Instance.Log("Eposh #" + epochNumber.ToString() + " finished; current error is " + currentError.ToString() + "; it takes: " + (DateTime.Now - dtStart).Duration().ToString());
, :
} while (epochNumber < _config.MaxEpoches && currentError > _config.MinError && Math.Abs(currentError - lastError) > _config.MinErrorChange);
. , , , . . , , , , , .
:

:
.
, , , , . -)
internal class BackpropagationFCNLearningAlgorithm : ILearningStrategy, public void Train(IMultilayerNeuralNetwork network, IList<DataItem> data).
( ) :
if (_config.BatchSize < 1 || _config.BatchSize > data.Count) { _config.BatchSize = data.Count; } double currentError = Single.MaxValue; double lastError = 0; int epochNumber = 0; Logger.Instance.Log("Start learning...");
, , :
do { //... } while (epochNumber < _config.MaxEpoches && currentError > _config.MinError && Math.Abs(currentError - lastError) > _config.MinErrorChange);
, , . , batch , .
lastError = currentError; DateTime dtStart = DateTime.Now; //preparation for epoche int[] trainingIndices = new int[data.Count]; for (int i = 0; i < data.Count; i++) { trainingIndices[i] = i; } if (_config.BatchSize > 0) { trainingIndices = Shuffle(trainingIndices); }
, , , :
//process data set int currentIndex = 0; do { #region initialize accumulated error for batch, for weights and biases double[][][] nablaWeights = new double[network.Layers.Length][][]; double[][] nablaBiases = new double[network.Layers.Length][]; for (int i = 0; i < network.Layers.Length; i++) { nablaBiases[i] = new double[network.Layers[i].Neurons.Length]; nablaWeights[i] = new double[network.Layers[i].Neurons.Length][]; for (int j = 0; j < network.Layers[i].Neurons.Length; j++) { nablaBiases[i][j] = 0; nablaWeights[i][j] = new double[network.Layers[i].Neurons[j].Weights.Length]; for (int k = 0; k < network.Layers[i].Neurons[j].Weights.Length; k++) { nablaWeights[i][j][k] = 0; } } } #endregion //process one batch for (int inBatchIndex = currentIndex; inBatchIndex < currentIndex + _config.BatchSize && inBatchIndex < data.Count; inBatchIndex++) { //forward pass double[] realOutput = network.ComputeOutput(data[trainingIndices[inBatchIndex]].Input); //backward pass, error propagation //last layer //....................................... //hidden layers //....................................... } //update weights and bias for (int layerIndex = 0; layerIndex < network.Layers.Length; layerIndex++) { for (int neuronIndex = 0; neuronIndex < network.Layers[layerIndex].Neurons.Length; neuronIndex++) { network.Layers[layerIndex].Neurons[neuronIndex].Bias -= nablaBiases[layerIndex][neuronIndex]; for (int weightIndex = 0; weightIndex < network.Layers[layerIndex].Neurons[neuronIndex].Weights.Length; weightIndex++) { network.Layers[layerIndex].Neurons[neuronIndex].Weights[weightIndex] -= nablaWeights[layerIndex][neuronIndex][weightIndex]; } } } currentIndex += _config.BatchSize; } while (currentIndex < data.Count);
:
"", ( , ) dE/dz
//last layer for (int j = 0; j < network.Layers[network.Layers.Length - 1].Neurons.Length; j++) { network.Layers[network.Layers.Length - 1].Neurons[j].dEdz = _config.ErrorFunction.CalculatePartialDerivaitveByV2Index(data[inBatchIndex].Output, realOutput, j) * network.Layers[network.Layers.Length - 1].Neurons[j].ActivationFunction. ComputeFirstDerivative(network.Layers[network.Layers.Length - 1].Neurons[j].LastNET); nablaBiases[network.Layers.Length - 1][j] += _config.LearningRate * network.Layers[network.Layers.Length - 1].Neurons[j].dEdz; for (int i = 0; i < network.Layers[network.Layers.Length - 1].Neurons[j].Weights.Length; i++) { nablaWeights[network.Layers.Length - 1][j][i] += _config.LearningRate*(network.Layers[network.Layers.Length - 1].Neurons[j].dEdz* (network.Layers.Length > 1 ? network.Layers[network.Layers.Length - 1 - 1].Neurons[i].LastState : data[inBatchIndex].Input[i]) + _config.RegularizationFactor * network.Layers[network.Layers.Length - 1].Neurons[j].Weights[i] / data.Count); } }
:
"", ( , ) dE/dz, ,
//hidden layers for (int hiddenLayerIndex = network.Layers.Length - 2; hiddenLayerIndex >= 0; hiddenLayerIndex--) { for (int j = 0; j < network.Layers[hiddenLayerIndex].Neurons.Length; j++) { network.Layers[hiddenLayerIndex].Neurons[j].dEdz = 0; for (int k = 0; k < network.Layers[hiddenLayerIndex + 1].Neurons.Length; k++) { network.Layers[hiddenLayerIndex].Neurons[j].dEdz += network.Layers[hiddenLayerIndex + 1].Neurons[k].Weights[j]* network.Layers[hiddenLayerIndex + 1].Neurons[k].dEdz; } network.Layers[hiddenLayerIndex].Neurons[j].dEdz *= network.Layers[hiddenLayerIndex].Neurons[j].ActivationFunction. ComputeFirstDerivative( network.Layers[hiddenLayerIndex].Neurons[j].LastNET ); nablaBiases[hiddenLayerIndex][j] += _config.LearningRate* network.Layers[hiddenLayerIndex].Neurons[j].dEdz; for (int i = 0; i < network.Layers[hiddenLayerIndex].Neurons[j].Weights.Length; i++) { nablaWeights[hiddenLayerIndex][j][i] += _config.LearningRate * ( network.Layers[hiddenLayerIndex].Neurons[j].dEdz * (hiddenLayerIndex > 0 ? network.Layers[hiddenLayerIndex - 1].Neurons[i].LastState : data[inBatchIndex].Input[i]) + _config.RegularizationFactor * network.Layers[hiddenLayerIndex].Neurons[j].Weights[i] / data.Count ); } } }
, ( ), :
//recalculating error on all data //real error currentError = 0; for (int i = 0; i < data.Count; i++) { double[] realOutput = network.ComputeOutput(data[i].Input); currentError += _config.ErrorFunction.Calculate(data[i].Output, realOutput); } currentError *= 1d/data.Count; //regularization term if (Math.Abs(_config.RegularizationFactor - 0d) > Double.Epsilon) { double reg = 0; for (int layerIndex = 0; layerIndex < network.Layers.Length; layerIndex++) { for (int neuronIndex = 0; neuronIndex < network.Layers[layerIndex].Neurons.Length; neuronIndex++) { for (int weightIndex = 0; weightIndex < network.Layers[layerIndex].Neurons[neuronIndex].Weights.Length; weightIndex++) { reg += network.Layers[layerIndex].Neurons[neuronIndex].Weights[weightIndex] * network.Layers[layerIndex].Neurons[neuronIndex].Weights[weightIndex]; } } } currentError += _config.RegularizationFactor * reg / (2 * data.Count); } epochNumber++; Logger.Instance.Log("Eposh #" + epochNumber.ToString() + " finished; current error is " + currentError.ToString() + "; it takes: " + (DateTime.Now - dtStart).Duration().ToString());
, :
} while (epochNumber < _config.MaxEpoches && currentError > _config.MinError && Math.Abs(currentError - lastError) > _config.MinErrorChange);
. , , , . . , , , , , .
:

:
.
, , , , . -)
internal class BackpropagationFCNLearningAlgorithm : ILearningStrategy, public void Train(IMultilayerNeuralNetwork network, IList<DataItem> data).
( ) :
if (_config.BatchSize < 1 || _config.BatchSize > data.Count) { _config.BatchSize = data.Count; } double currentError = Single.MaxValue; double lastError = 0; int epochNumber = 0; Logger.Instance.Log("Start learning...");
, , :
do { //... } while (epochNumber < _config.MaxEpoches && currentError > _config.MinError && Math.Abs(currentError - lastError) > _config.MinErrorChange);
, , . , batch , .
lastError = currentError; DateTime dtStart = DateTime.Now; //preparation for epoche int[] trainingIndices = new int[data.Count]; for (int i = 0; i < data.Count; i++) { trainingIndices[i] = i; } if (_config.BatchSize > 0) { trainingIndices = Shuffle(trainingIndices); }
, , , :
//process data set int currentIndex = 0; do { #region initialize accumulated error for batch, for weights and biases double[][][] nablaWeights = new double[network.Layers.Length][][]; double[][] nablaBiases = new double[network.Layers.Length][]; for (int i = 0; i < network.Layers.Length; i++) { nablaBiases[i] = new double[network.Layers[i].Neurons.Length]; nablaWeights[i] = new double[network.Layers[i].Neurons.Length][]; for (int j = 0; j < network.Layers[i].Neurons.Length; j++) { nablaBiases[i][j] = 0; nablaWeights[i][j] = new double[network.Layers[i].Neurons[j].Weights.Length]; for (int k = 0; k < network.Layers[i].Neurons[j].Weights.Length; k++) { nablaWeights[i][j][k] = 0; } } } #endregion //process one batch for (int inBatchIndex = currentIndex; inBatchIndex < currentIndex + _config.BatchSize && inBatchIndex < data.Count; inBatchIndex++) { //forward pass double[] realOutput = network.ComputeOutput(data[trainingIndices[inBatchIndex]].Input); //backward pass, error propagation //last layer //....................................... //hidden layers //....................................... } //update weights and bias for (int layerIndex = 0; layerIndex < network.Layers.Length; layerIndex++) { for (int neuronIndex = 0; neuronIndex < network.Layers[layerIndex].Neurons.Length; neuronIndex++) { network.Layers[layerIndex].Neurons[neuronIndex].Bias -= nablaBiases[layerIndex][neuronIndex]; for (int weightIndex = 0; weightIndex < network.Layers[layerIndex].Neurons[neuronIndex].Weights.Length; weightIndex++) { network.Layers[layerIndex].Neurons[neuronIndex].Weights[weightIndex] -= nablaWeights[layerIndex][neuronIndex][weightIndex]; } } } currentIndex += _config.BatchSize; } while (currentIndex < data.Count);
:
"", ( , ) dE/dz
//last layer for (int j = 0; j < network.Layers[network.Layers.Length - 1].Neurons.Length; j++) { network.Layers[network.Layers.Length - 1].Neurons[j].dEdz = _config.ErrorFunction.CalculatePartialDerivaitveByV2Index(data[inBatchIndex].Output, realOutput, j) * network.Layers[network.Layers.Length - 1].Neurons[j].ActivationFunction. ComputeFirstDerivative(network.Layers[network.Layers.Length - 1].Neurons[j].LastNET); nablaBiases[network.Layers.Length - 1][j] += _config.LearningRate * network.Layers[network.Layers.Length - 1].Neurons[j].dEdz; for (int i = 0; i < network.Layers[network.Layers.Length - 1].Neurons[j].Weights.Length; i++) { nablaWeights[network.Layers.Length - 1][j][i] += _config.LearningRate*(network.Layers[network.Layers.Length - 1].Neurons[j].dEdz* (network.Layers.Length > 1 ? network.Layers[network.Layers.Length - 1 - 1].Neurons[i].LastState : data[inBatchIndex].Input[i]) + _config.RegularizationFactor * network.Layers[network.Layers.Length - 1].Neurons[j].Weights[i] / data.Count); } }
:
"", ( , ) dE/dz, ,
//hidden layers for (int hiddenLayerIndex = network.Layers.Length - 2; hiddenLayerIndex >= 0; hiddenLayerIndex--) { for (int j = 0; j < network.Layers[hiddenLayerIndex].Neurons.Length; j++) { network.Layers[hiddenLayerIndex].Neurons[j].dEdz = 0; for (int k = 0; k < network.Layers[hiddenLayerIndex + 1].Neurons.Length; k++) { network.Layers[hiddenLayerIndex].Neurons[j].dEdz += network.Layers[hiddenLayerIndex + 1].Neurons[k].Weights[j]* network.Layers[hiddenLayerIndex + 1].Neurons[k].dEdz; } network.Layers[hiddenLayerIndex].Neurons[j].dEdz *= network.Layers[hiddenLayerIndex].Neurons[j].ActivationFunction. ComputeFirstDerivative( network.Layers[hiddenLayerIndex].Neurons[j].LastNET ); nablaBiases[hiddenLayerIndex][j] += _config.LearningRate* network.Layers[hiddenLayerIndex].Neurons[j].dEdz; for (int i = 0; i < network.Layers[hiddenLayerIndex].Neurons[j].Weights.Length; i++) { nablaWeights[hiddenLayerIndex][j][i] += _config.LearningRate * ( network.Layers[hiddenLayerIndex].Neurons[j].dEdz * (hiddenLayerIndex > 0 ? network.Layers[hiddenLayerIndex - 1].Neurons[i].LastState : data[inBatchIndex].Input[i]) + _config.RegularizationFactor * network.Layers[hiddenLayerIndex].Neurons[j].Weights[i] / data.Count ); } } }
, ( ), :
//recalculating error on all data //real error currentError = 0; for (int i = 0; i < data.Count; i++) { double[] realOutput = network.ComputeOutput(data[i].Input); currentError += _config.ErrorFunction.Calculate(data[i].Output, realOutput); } currentError *= 1d/data.Count; //regularization term if (Math.Abs(_config.RegularizationFactor - 0d) > Double.Epsilon) { double reg = 0; for (int layerIndex = 0; layerIndex < network.Layers.Length; layerIndex++) { for (int neuronIndex = 0; neuronIndex < network.Layers[layerIndex].Neurons.Length; neuronIndex++) { for (int weightIndex = 0; weightIndex < network.Layers[layerIndex].Neurons[neuronIndex].Weights.Length; weightIndex++) { reg += network.Layers[layerIndex].Neurons[neuronIndex].Weights[weightIndex] * network.Layers[layerIndex].Neurons[neuronIndex].Weights[weightIndex]; } } } currentError += _config.RegularizationFactor * reg / (2 * data.Count); } epochNumber++; Logger.Instance.Log("Eposh #" + epochNumber.ToString() + " finished; current error is " + currentError.ToString() + "; it takes: " + (DateTime.Now - dtStart).Duration().ToString());
, :
} while (epochNumber < _config.MaxEpoches && currentError > _config.MinError && Math.Abs(currentError - lastError) > _config.MinErrorChange);
. , , , . . , , , , , .
:

:
.
, , , , . -)
internal class BackpropagationFCNLearningAlgorithm : ILearningStrategy, public void Train(IMultilayerNeuralNetwork network, IList<DataItem> data).
( ) :
if (_config.BatchSize < 1 || _config.BatchSize > data.Count) { _config.BatchSize = data.Count; } double currentError = Single.MaxValue; double lastError = 0; int epochNumber = 0; Logger.Instance.Log("Start learning...");
, , :
do { //... } while (epochNumber < _config.MaxEpoches && currentError > _config.MinError && Math.Abs(currentError - lastError) > _config.MinErrorChange);
, , . , batch , .
lastError = currentError; DateTime dtStart = DateTime.Now; //preparation for epoche int[] trainingIndices = new int[data.Count]; for (int i = 0; i < data.Count; i++) { trainingIndices[i] = i; } if (_config.BatchSize > 0) { trainingIndices = Shuffle(trainingIndices); }
, , , :
//process data set int currentIndex = 0; do { #region initialize accumulated error for batch, for weights and biases double[][][] nablaWeights = new double[network.Layers.Length][][]; double[][] nablaBiases = new double[network.Layers.Length][]; for (int i = 0; i < network.Layers.Length; i++) { nablaBiases[i] = new double[network.Layers[i].Neurons.Length]; nablaWeights[i] = new double[network.Layers[i].Neurons.Length][]; for (int j = 0; j < network.Layers[i].Neurons.Length; j++) { nablaBiases[i][j] = 0; nablaWeights[i][j] = new double[network.Layers[i].Neurons[j].Weights.Length]; for (int k = 0; k < network.Layers[i].Neurons[j].Weights.Length; k++) { nablaWeights[i][j][k] = 0; } } } #endregion //process one batch for (int inBatchIndex = currentIndex; inBatchIndex < currentIndex + _config.BatchSize && inBatchIndex < data.Count; inBatchIndex++) { //forward pass double[] realOutput = network.ComputeOutput(data[trainingIndices[inBatchIndex]].Input); //backward pass, error propagation //last layer //....................................... //hidden layers //....................................... } //update weights and bias for (int layerIndex = 0; layerIndex < network.Layers.Length; layerIndex++) { for (int neuronIndex = 0; neuronIndex < network.Layers[layerIndex].Neurons.Length; neuronIndex++) { network.Layers[layerIndex].Neurons[neuronIndex].Bias -= nablaBiases[layerIndex][neuronIndex]; for (int weightIndex = 0; weightIndex < network.Layers[layerIndex].Neurons[neuronIndex].Weights.Length; weightIndex++) { network.Layers[layerIndex].Neurons[neuronIndex].Weights[weightIndex] -= nablaWeights[layerIndex][neuronIndex][weightIndex]; } } } currentIndex += _config.BatchSize; } while (currentIndex < data.Count);
:
"", ( , ) dE/dz
//last layer for (int j = 0; j < network.Layers[network.Layers.Length - 1].Neurons.Length; j++) { network.Layers[network.Layers.Length - 1].Neurons[j].dEdz = _config.ErrorFunction.CalculatePartialDerivaitveByV2Index(data[inBatchIndex].Output, realOutput, j) * network.Layers[network.Layers.Length - 1].Neurons[j].ActivationFunction. ComputeFirstDerivative(network.Layers[network.Layers.Length - 1].Neurons[j].LastNET); nablaBiases[network.Layers.Length - 1][j] += _config.LearningRate * network.Layers[network.Layers.Length - 1].Neurons[j].dEdz; for (int i = 0; i < network.Layers[network.Layers.Length - 1].Neurons[j].Weights.Length; i++) { nablaWeights[network.Layers.Length - 1][j][i] += _config.LearningRate*(network.Layers[network.Layers.Length - 1].Neurons[j].dEdz* (network.Layers.Length > 1 ? network.Layers[network.Layers.Length - 1 - 1].Neurons[i].LastState : data[inBatchIndex].Input[i]) + _config.RegularizationFactor * network.Layers[network.Layers.Length - 1].Neurons[j].Weights[i] / data.Count); } }
:
"", ( , ) dE/dz, ,
//hidden layers for (int hiddenLayerIndex = network.Layers.Length - 2; hiddenLayerIndex >= 0; hiddenLayerIndex--) { for (int j = 0; j < network.Layers[hiddenLayerIndex].Neurons.Length; j++) { network.Layers[hiddenLayerIndex].Neurons[j].dEdz = 0; for (int k = 0; k < network.Layers[hiddenLayerIndex + 1].Neurons.Length; k++) { network.Layers[hiddenLayerIndex].Neurons[j].dEdz += network.Layers[hiddenLayerIndex + 1].Neurons[k].Weights[j]* network.Layers[hiddenLayerIndex + 1].Neurons[k].dEdz; } network.Layers[hiddenLayerIndex].Neurons[j].dEdz *= network.Layers[hiddenLayerIndex].Neurons[j].ActivationFunction. ComputeFirstDerivative( network.Layers[hiddenLayerIndex].Neurons[j].LastNET ); nablaBiases[hiddenLayerIndex][j] += _config.LearningRate* network.Layers[hiddenLayerIndex].Neurons[j].dEdz; for (int i = 0; i < network.Layers[hiddenLayerIndex].Neurons[j].Weights.Length; i++) { nablaWeights[hiddenLayerIndex][j][i] += _config.LearningRate * ( network.Layers[hiddenLayerIndex].Neurons[j].dEdz * (hiddenLayerIndex > 0 ? network.Layers[hiddenLayerIndex - 1].Neurons[i].LastState : data[inBatchIndex].Input[i]) + _config.RegularizationFactor * network.Layers[hiddenLayerIndex].Neurons[j].Weights[i] / data.Count ); } } }
, ( ), :
//recalculating error on all data //real error currentError = 0; for (int i = 0; i < data.Count; i++) { double[] realOutput = network.ComputeOutput(data[i].Input); currentError += _config.ErrorFunction.Calculate(data[i].Output, realOutput); } currentError *= 1d/data.Count; //regularization term if (Math.Abs(_config.RegularizationFactor - 0d) > Double.Epsilon) { double reg = 0; for (int layerIndex = 0; layerIndex < network.Layers.Length; layerIndex++) { for (int neuronIndex = 0; neuronIndex < network.Layers[layerIndex].Neurons.Length; neuronIndex++) { for (int weightIndex = 0; weightIndex < network.Layers[layerIndex].Neurons[neuronIndex].Weights.Length; weightIndex++) { reg += network.Layers[layerIndex].Neurons[neuronIndex].Weights[weightIndex] * network.Layers[layerIndex].Neurons[neuronIndex].Weights[weightIndex]; } } } currentError += _config.RegularizationFactor * reg / (2 * data.Count); } epochNumber++; Logger.Instance.Log("Eposh #" + epochNumber.ToString() + " finished; current error is " + currentError.ToString() + "; it takes: " + (DateTime.Now - dtStart).Duration().ToString());
, :
} while (epochNumber < _config.MaxEpoches && currentError > _config.MinError && Math.Abs(currentError - lastError) > _config.MinErrorChange);
. , , , . . , , , , , .
:

:
.
, , , , . -)
internal class BackpropagationFCNLearningAlgorithm : ILearningStrategy, public void Train(IMultilayerNeuralNetwork network, IList<DataItem> data).
( ) :
if (_config.BatchSize < 1 || _config.BatchSize > data.Count) { _config.BatchSize = data.Count; } double currentError = Single.MaxValue; double lastError = 0; int epochNumber = 0; Logger.Instance.Log("Start learning...");
, , :
do { //... } while (epochNumber < _config.MaxEpoches && currentError > _config.MinError && Math.Abs(currentError - lastError) > _config.MinErrorChange);
, , . , batch , .
lastError = currentError; DateTime dtStart = DateTime.Now; //preparation for epoche int[] trainingIndices = new int[data.Count]; for (int i = 0; i < data.Count; i++) { trainingIndices[i] = i; } if (_config.BatchSize > 0) { trainingIndices = Shuffle(trainingIndices); }
, , , :
//process data set int currentIndex = 0; do { #region initialize accumulated error for batch, for weights and biases double[][][] nablaWeights = new double[network.Layers.Length][][]; double[][] nablaBiases = new double[network.Layers.Length][]; for (int i = 0; i < network.Layers.Length; i++) { nablaBiases[i] = new double[network.Layers[i].Neurons.Length]; nablaWeights[i] = new double[network.Layers[i].Neurons.Length][]; for (int j = 0; j < network.Layers[i].Neurons.Length; j++) { nablaBiases[i][j] = 0; nablaWeights[i][j] = new double[network.Layers[i].Neurons[j].Weights.Length]; for (int k = 0; k < network.Layers[i].Neurons[j].Weights.Length; k++) { nablaWeights[i][j][k] = 0; } } } #endregion //process one batch for (int inBatchIndex = currentIndex; inBatchIndex < currentIndex + _config.BatchSize && inBatchIndex < data.Count; inBatchIndex++) { //forward pass double[] realOutput = network.ComputeOutput(data[trainingIndices[inBatchIndex]].Input); //backward pass, error propagation //last layer //....................................... //hidden layers //....................................... } //update weights and bias for (int layerIndex = 0; layerIndex < network.Layers.Length; layerIndex++) { for (int neuronIndex = 0; neuronIndex < network.Layers[layerIndex].Neurons.Length; neuronIndex++) { network.Layers[layerIndex].Neurons[neuronIndex].Bias -= nablaBiases[layerIndex][neuronIndex]; for (int weightIndex = 0; weightIndex < network.Layers[layerIndex].Neurons[neuronIndex].Weights.Length; weightIndex++) { network.Layers[layerIndex].Neurons[neuronIndex].Weights[weightIndex] -= nablaWeights[layerIndex][neuronIndex][weightIndex]; } } } currentIndex += _config.BatchSize; } while (currentIndex < data.Count);
:
"", ( , ) dE/dz
//last layer for (int j = 0; j < network.Layers[network.Layers.Length - 1].Neurons.Length; j++) { network.Layers[network.Layers.Length - 1].Neurons[j].dEdz = _config.ErrorFunction.CalculatePartialDerivaitveByV2Index(data[inBatchIndex].Output, realOutput, j) * network.Layers[network.Layers.Length - 1].Neurons[j].ActivationFunction. ComputeFirstDerivative(network.Layers[network.Layers.Length - 1].Neurons[j].LastNET); nablaBiases[network.Layers.Length - 1][j] += _config.LearningRate * network.Layers[network.Layers.Length - 1].Neurons[j].dEdz; for (int i = 0; i < network.Layers[network.Layers.Length - 1].Neurons[j].Weights.Length; i++) { nablaWeights[network.Layers.Length - 1][j][i] += _config.LearningRate*(network.Layers[network.Layers.Length - 1].Neurons[j].dEdz* (network.Layers.Length > 1 ? network.Layers[network.Layers.Length - 1 - 1].Neurons[i].LastState : data[inBatchIndex].Input[i]) + _config.RegularizationFactor * network.Layers[network.Layers.Length - 1].Neurons[j].Weights[i] / data.Count); } }
:
"", ( , ) dE/dz, ,
//hidden layers for (int hiddenLayerIndex = network.Layers.Length - 2; hiddenLayerIndex >= 0; hiddenLayerIndex--) { for (int j = 0; j < network.Layers[hiddenLayerIndex].Neurons.Length; j++) { network.Layers[hiddenLayerIndex].Neurons[j].dEdz = 0; for (int k = 0; k < network.Layers[hiddenLayerIndex + 1].Neurons.Length; k++) { network.Layers[hiddenLayerIndex].Neurons[j].dEdz += network.Layers[hiddenLayerIndex + 1].Neurons[k].Weights[j]* network.Layers[hiddenLayerIndex + 1].Neurons[k].dEdz; } network.Layers[hiddenLayerIndex].Neurons[j].dEdz *= network.Layers[hiddenLayerIndex].Neurons[j].ActivationFunction. ComputeFirstDerivative( network.Layers[hiddenLayerIndex].Neurons[j].LastNET ); nablaBiases[hiddenLayerIndex][j] += _config.LearningRate* network.Layers[hiddenLayerIndex].Neurons[j].dEdz; for (int i = 0; i < network.Layers[hiddenLayerIndex].Neurons[j].Weights.Length; i++) { nablaWeights[hiddenLayerIndex][j][i] += _config.LearningRate * ( network.Layers[hiddenLayerIndex].Neurons[j].dEdz * (hiddenLayerIndex > 0 ? network.Layers[hiddenLayerIndex - 1].Neurons[i].LastState : data[inBatchIndex].Input[i]) + _config.RegularizationFactor * network.Layers[hiddenLayerIndex].Neurons[j].Weights[i] / data.Count ); } } }
, ( ), :
//recalculating error on all data //real error currentError = 0; for (int i = 0; i < data.Count; i++) { double[] realOutput = network.ComputeOutput(data[i].Input); currentError += _config.ErrorFunction.Calculate(data[i].Output, realOutput); } currentError *= 1d/data.Count; //regularization term if (Math.Abs(_config.RegularizationFactor - 0d) > Double.Epsilon) { double reg = 0; for (int layerIndex = 0; layerIndex < network.Layers.Length; layerIndex++) { for (int neuronIndex = 0; neuronIndex < network.Layers[layerIndex].Neurons.Length; neuronIndex++) { for (int weightIndex = 0; weightIndex < network.Layers[layerIndex].Neurons[neuronIndex].Weights.Length; weightIndex++) { reg += network.Layers[layerIndex].Neurons[neuronIndex].Weights[weightIndex] * network.Layers[layerIndex].Neurons[neuronIndex].Weights[weightIndex]; } } } currentError += _config.RegularizationFactor * reg / (2 * data.Count); } epochNumber++; Logger.Instance.Log("Eposh #" + epochNumber.ToString() + " finished; current error is " + currentError.ToString() + "; it takes: " + (DateTime.Now - dtStart).Duration().ToString());
, :
} while (epochNumber < _config.MaxEpoches && currentError > _config.MinError && Math.Abs(currentError - lastError) > _config.MinErrorChange);
. , , , . . , , , , , .
:
:
.
, , , , . -)
internal class BackpropagationFCNLearningAlgorithm : ILearningStrategy, public void Train(IMultilayerNeuralNetwork network, IList<DataItem> data).
( ) :
if (_config.BatchSize < 1 || _config.BatchSize > data.Count) { _config.BatchSize = data.Count; } double currentError = Single.MaxValue; double lastError = 0; int epochNumber = 0; Logger.Instance.Log("Start learning...");
, , :
do { //... } while (epochNumber < _config.MaxEpoches && currentError > _config.MinError && Math.Abs(currentError - lastError) > _config.MinErrorChange);
, , . , batch , .
lastError = currentError; DateTime dtStart = DateTime.Now; //preparation for epoche int[] trainingIndices = new int[data.Count]; for (int i = 0; i < data.Count; i++) { trainingIndices[i] = i; } if (_config.BatchSize > 0) { trainingIndices = Shuffle(trainingIndices); }
, , , :
//process data set int currentIndex = 0; do { #region initialize accumulated error for batch, for weights and biases double[][][] nablaWeights = new double[network.Layers.Length][][]; double[][] nablaBiases = new double[network.Layers.Length][]; for (int i = 0; i < network.Layers.Length; i++) { nablaBiases[i] = new double[network.Layers[i].Neurons.Length]; nablaWeights[i] = new double[network.Layers[i].Neurons.Length][]; for (int j = 0; j < network.Layers[i].Neurons.Length; j++) { nablaBiases[i][j] = 0; nablaWeights[i][j] = new double[network.Layers[i].Neurons[j].Weights.Length]; for (int k = 0; k < network.Layers[i].Neurons[j].Weights.Length; k++) { nablaWeights[i][j][k] = 0; } } } #endregion //process one batch for (int inBatchIndex = currentIndex; inBatchIndex < currentIndex + _config.BatchSize && inBatchIndex < data.Count; inBatchIndex++) { //forward pass double[] realOutput = network.ComputeOutput(data[trainingIndices[inBatchIndex]].Input); //backward pass, error propagation //last layer //....................................... //hidden layers //....................................... } //update weights and bias for (int layerIndex = 0; layerIndex < network.Layers.Length; layerIndex++) { for (int neuronIndex = 0; neuronIndex < network.Layers[layerIndex].Neurons.Length; neuronIndex++) { network.Layers[layerIndex].Neurons[neuronIndex].Bias -= nablaBiases[layerIndex][neuronIndex]; for (int weightIndex = 0; weightIndex < network.Layers[layerIndex].Neurons[neuronIndex].Weights.Length; weightIndex++) { network.Layers[layerIndex].Neurons[neuronIndex].Weights[weightIndex] -= nablaWeights[layerIndex][neuronIndex][weightIndex]; } } } currentIndex += _config.BatchSize; } while (currentIndex < data.Count);
:
"", ( , ) dE/dz
//last layer for (int j = 0; j < network.Layers[network.Layers.Length - 1].Neurons.Length; j++) { network.Layers[network.Layers.Length - 1].Neurons[j].dEdz = _config.ErrorFunction.CalculatePartialDerivaitveByV2Index(data[inBatchIndex].Output, realOutput, j) * network.Layers[network.Layers.Length - 1].Neurons[j].ActivationFunction. ComputeFirstDerivative(network.Layers[network.Layers.Length - 1].Neurons[j].LastNET); nablaBiases[network.Layers.Length - 1][j] += _config.LearningRate * network.Layers[network.Layers.Length - 1].Neurons[j].dEdz; for (int i = 0; i < network.Layers[network.Layers.Length - 1].Neurons[j].Weights.Length; i++) { nablaWeights[network.Layers.Length - 1][j][i] += _config.LearningRate*(network.Layers[network.Layers.Length - 1].Neurons[j].dEdz* (network.Layers.Length > 1 ? network.Layers[network.Layers.Length - 1 - 1].Neurons[i].LastState : data[inBatchIndex].Input[i]) + _config.RegularizationFactor * network.Layers[network.Layers.Length - 1].Neurons[j].Weights[i] / data.Count); } }
:
"", ( , ) dE/dz, ,
//hidden layers for (int hiddenLayerIndex = network.Layers.Length - 2; hiddenLayerIndex >= 0; hiddenLayerIndex--) { for (int j = 0; j < network.Layers[hiddenLayerIndex].Neurons.Length; j++) { network.Layers[hiddenLayerIndex].Neurons[j].dEdz = 0; for (int k = 0; k < network.Layers[hiddenLayerIndex + 1].Neurons.Length; k++) { network.Layers[hiddenLayerIndex].Neurons[j].dEdz += network.Layers[hiddenLayerIndex + 1].Neurons[k].Weights[j]* network.Layers[hiddenLayerIndex + 1].Neurons[k].dEdz; } network.Layers[hiddenLayerIndex].Neurons[j].dEdz *= network.Layers[hiddenLayerIndex].Neurons[j].ActivationFunction. ComputeFirstDerivative( network.Layers[hiddenLayerIndex].Neurons[j].LastNET ); nablaBiases[hiddenLayerIndex][j] += _config.LearningRate* network.Layers[hiddenLayerIndex].Neurons[j].dEdz; for (int i = 0; i < network.Layers[hiddenLayerIndex].Neurons[j].Weights.Length; i++) { nablaWeights[hiddenLayerIndex][j][i] += _config.LearningRate * ( network.Layers[hiddenLayerIndex].Neurons[j].dEdz * (hiddenLayerIndex > 0 ? network.Layers[hiddenLayerIndex - 1].Neurons[i].LastState : data[inBatchIndex].Input[i]) + _config.RegularizationFactor * network.Layers[hiddenLayerIndex].Neurons[j].Weights[i] / data.Count ); } } }
, ( ), :
//recalculating error on all data //real error currentError = 0; for (int i = 0; i < data.Count; i++) { double[] realOutput = network.ComputeOutput(data[i].Input); currentError += _config.ErrorFunction.Calculate(data[i].Output, realOutput); } currentError *= 1d/data.Count; //regularization term if (Math.Abs(_config.RegularizationFactor - 0d) > Double.Epsilon) { double reg = 0; for (int layerIndex = 0; layerIndex < network.Layers.Length; layerIndex++) { for (int neuronIndex = 0; neuronIndex < network.Layers[layerIndex].Neurons.Length; neuronIndex++) { for (int weightIndex = 0; weightIndex < network.Layers[layerIndex].Neurons[neuronIndex].Weights.Length; weightIndex++) { reg += network.Layers[layerIndex].Neurons[neuronIndex].Weights[weightIndex] * network.Layers[layerIndex].Neurons[neuronIndex].Weights[weightIndex]; } } } currentError += _config.RegularizationFactor * reg / (2 * data.Count); } epochNumber++; Logger.Instance.Log("Eposh #" + epochNumber.ToString() + " finished; current error is " + currentError.ToString() + "; it takes: " + (DateTime.Now - dtStart).Duration().ToString());
, :
} while (epochNumber < _config.MaxEpoches && currentError > _config.MinError && Math.Abs(currentError - lastError) > _config.MinErrorChange);
. , , , . . , , , , , .
:

:
.
, , , , . -)
internal class BackpropagationFCNLearningAlgorithm : ILearningStrategy, public void Train(IMultilayerNeuralNetwork network, IList<DataItem> data).
( ) :
if (_config.BatchSize < 1 || _config.BatchSize > data.Count) { _config.BatchSize = data.Count; } double currentError = Single.MaxValue; double lastError = 0; int epochNumber = 0; Logger.Instance.Log("Start learning...");
, , :
do { //... } while (epochNumber < _config.MaxEpoches && currentError > _config.MinError && Math.Abs(currentError - lastError) > _config.MinErrorChange);
, , . , batch , .
lastError = currentError; DateTime dtStart = DateTime.Now; //preparation for epoche int[] trainingIndices = new int[data.Count]; for (int i = 0; i < data.Count; i++) { trainingIndices[i] = i; } if (_config.BatchSize > 0) { trainingIndices = Shuffle(trainingIndices); }
, , , :
//process data set int currentIndex = 0; do { #region initialize accumulated error for batch, for weights and biases double[][][] nablaWeights = new double[network.Layers.Length][][]; double[][] nablaBiases = new double[network.Layers.Length][]; for (int i = 0; i < network.Layers.Length; i++) { nablaBiases[i] = new double[network.Layers[i].Neurons.Length]; nablaWeights[i] = new double[network.Layers[i].Neurons.Length][]; for (int j = 0; j < network.Layers[i].Neurons.Length; j++) { nablaBiases[i][j] = 0; nablaWeights[i][j] = new double[network.Layers[i].Neurons[j].Weights.Length]; for (int k = 0; k < network.Layers[i].Neurons[j].Weights.Length; k++) { nablaWeights[i][j][k] = 0; } } } #endregion //process one batch for (int inBatchIndex = currentIndex; inBatchIndex < currentIndex + _config.BatchSize && inBatchIndex < data.Count; inBatchIndex++) { //forward pass double[] realOutput = network.ComputeOutput(data[trainingIndices[inBatchIndex]].Input); //backward pass, error propagation //last layer //....................................... //hidden layers //....................................... } //update weights and bias for (int layerIndex = 0; layerIndex < network.Layers.Length; layerIndex++) { for (int neuronIndex = 0; neuronIndex < network.Layers[layerIndex].Neurons.Length; neuronIndex++) { network.Layers[layerIndex].Neurons[neuronIndex].Bias -= nablaBiases[layerIndex][neuronIndex]; for (int weightIndex = 0; weightIndex < network.Layers[layerIndex].Neurons[neuronIndex].Weights.Length; weightIndex++) { network.Layers[layerIndex].Neurons[neuronIndex].Weights[weightIndex] -= nablaWeights[layerIndex][neuronIndex][weightIndex]; } } } currentIndex += _config.BatchSize; } while (currentIndex < data.Count);
:
"", ( , ) dE/dz
//last layer for (int j = 0; j < network.Layers[network.Layers.Length - 1].Neurons.Length; j++) { network.Layers[network.Layers.Length - 1].Neurons[j].dEdz = _config.ErrorFunction.CalculatePartialDerivaitveByV2Index(data[inBatchIndex].Output, realOutput, j) * network.Layers[network.Layers.Length - 1].Neurons[j].ActivationFunction. ComputeFirstDerivative(network.Layers[network.Layers.Length - 1].Neurons[j].LastNET); nablaBiases[network.Layers.Length - 1][j] += _config.LearningRate * network.Layers[network.Layers.Length - 1].Neurons[j].dEdz; for (int i = 0; i < network.Layers[network.Layers.Length - 1].Neurons[j].Weights.Length; i++) { nablaWeights[network.Layers.Length - 1][j][i] += _config.LearningRate*(network.Layers[network.Layers.Length - 1].Neurons[j].dEdz* (network.Layers.Length > 1 ? network.Layers[network.Layers.Length - 1 - 1].Neurons[i].LastState : data[inBatchIndex].Input[i]) + _config.RegularizationFactor * network.Layers[network.Layers.Length - 1].Neurons[j].Weights[i] / data.Count); } }
:
"", ( , ) dE/dz, ,
//hidden layers for (int hiddenLayerIndex = network.Layers.Length - 2; hiddenLayerIndex >= 0; hiddenLayerIndex--) { for (int j = 0; j < network.Layers[hiddenLayerIndex].Neurons.Length; j++) { network.Layers[hiddenLayerIndex].Neurons[j].dEdz = 0; for (int k = 0; k < network.Layers[hiddenLayerIndex + 1].Neurons.Length; k++) { network.Layers[hiddenLayerIndex].Neurons[j].dEdz += network.Layers[hiddenLayerIndex + 1].Neurons[k].Weights[j]* network.Layers[hiddenLayerIndex + 1].Neurons[k].dEdz; } network.Layers[hiddenLayerIndex].Neurons[j].dEdz *= network.Layers[hiddenLayerIndex].Neurons[j].ActivationFunction. ComputeFirstDerivative( network.Layers[hiddenLayerIndex].Neurons[j].LastNET ); nablaBiases[hiddenLayerIndex][j] += _config.LearningRate* network.Layers[hiddenLayerIndex].Neurons[j].dEdz; for (int i = 0; i < network.Layers[hiddenLayerIndex].Neurons[j].Weights.Length; i++) { nablaWeights[hiddenLayerIndex][j][i] += _config.LearningRate * ( network.Layers[hiddenLayerIndex].Neurons[j].dEdz * (hiddenLayerIndex > 0 ? network.Layers[hiddenLayerIndex - 1].Neurons[i].LastState : data[inBatchIndex].Input[i]) + _config.RegularizationFactor * network.Layers[hiddenLayerIndex].Neurons[j].Weights[i] / data.Count ); } } }
, ( ), :
//recalculating error on all data //real error currentError = 0; for (int i = 0; i < data.Count; i++) { double[] realOutput = network.ComputeOutput(data[i].Input); currentError += _config.ErrorFunction.Calculate(data[i].Output, realOutput); } currentError *= 1d/data.Count; //regularization term if (Math.Abs(_config.RegularizationFactor - 0d) > Double.Epsilon) { double reg = 0; for (int layerIndex = 0; layerIndex < network.Layers.Length; layerIndex++) { for (int neuronIndex = 0; neuronIndex < network.Layers[layerIndex].Neurons.Length; neuronIndex++) { for (int weightIndex = 0; weightIndex < network.Layers[layerIndex].Neurons[neuronIndex].Weights.Length; weightIndex++) { reg += network.Layers[layerIndex].Neurons[neuronIndex].Weights[weightIndex] * network.Layers[layerIndex].Neurons[neuronIndex].Weights[weightIndex]; } } } currentError += _config.RegularizationFactor * reg / (2 * data.Count); } epochNumber++; Logger.Instance.Log("Eposh #" + epochNumber.ToString() + " finished; current error is " + currentError.ToString() + "; it takes: " + (DateTime.Now - dtStart).Duration().ToString());
, :
} while (epochNumber < _config.MaxEpoches && currentError > _config.MinError && Math.Abs(currentError - lastError) > _config.MinErrorChange);
. , , , . . , , , , , .
:

:
.
, , , , . -)
internal class BackpropagationFCNLearningAlgorithm : ILearningStrategy, public void Train(IMultilayerNeuralNetwork network, IList<DataItem> data).
( ) :
if (_config.BatchSize < 1 || _config.BatchSize > data.Count) { _config.BatchSize = data.Count; } double currentError = Single.MaxValue; double lastError = 0; int epochNumber = 0; Logger.Instance.Log("Start learning...");
, , :
do { //... } while (epochNumber < _config.MaxEpoches && currentError > _config.MinError && Math.Abs(currentError - lastError) > _config.MinErrorChange);
, , . , batch , .
lastError = currentError; DateTime dtStart = DateTime.Now; //preparation for epoche int[] trainingIndices = new int[data.Count]; for (int i = 0; i < data.Count; i++) { trainingIndices[i] = i; } if (_config.BatchSize > 0) { trainingIndices = Shuffle(trainingIndices); }
, , , :
//process data set int currentIndex = 0; do { #region initialize accumulated error for batch, for weights and biases double[][][] nablaWeights = new double[network.Layers.Length][][]; double[][] nablaBiases = new double[network.Layers.Length][]; for (int i = 0; i < network.Layers.Length; i++) { nablaBiases[i] = new double[network.Layers[i].Neurons.Length]; nablaWeights[i] = new double[network.Layers[i].Neurons.Length][]; for (int j = 0; j < network.Layers[i].Neurons.Length; j++) { nablaBiases[i][j] = 0; nablaWeights[i][j] = new double[network.Layers[i].Neurons[j].Weights.Length]; for (int k = 0; k < network.Layers[i].Neurons[j].Weights.Length; k++) { nablaWeights[i][j][k] = 0; } } } #endregion //process one batch for (int inBatchIndex = currentIndex; inBatchIndex < currentIndex + _config.BatchSize && inBatchIndex < data.Count; inBatchIndex++) { //forward pass double[] realOutput = network.ComputeOutput(data[trainingIndices[inBatchIndex]].Input); //backward pass, error propagation //last layer //....................................... //hidden layers //....................................... } //update weights and bias for (int layerIndex = 0; layerIndex < network.Layers.Length; layerIndex++) { for (int neuronIndex = 0; neuronIndex < network.Layers[layerIndex].Neurons.Length; neuronIndex++) { network.Layers[layerIndex].Neurons[neuronIndex].Bias -= nablaBiases[layerIndex][neuronIndex]; for (int weightIndex = 0; weightIndex < network.Layers[layerIndex].Neurons[neuronIndex].Weights.Length; weightIndex++) { network.Layers[layerIndex].Neurons[neuronIndex].Weights[weightIndex] -= nablaWeights[layerIndex][neuronIndex][weightIndex]; } } } currentIndex += _config.BatchSize; } while (currentIndex < data.Count);
:
"", ( , ) dE/dz
//last layer for (int j = 0; j < network.Layers[network.Layers.Length - 1].Neurons.Length; j++) { network.Layers[network.Layers.Length - 1].Neurons[j].dEdz = _config.ErrorFunction.CalculatePartialDerivaitveByV2Index(data[inBatchIndex].Output, realOutput, j) * network.Layers[network.Layers.Length - 1].Neurons[j].ActivationFunction. ComputeFirstDerivative(network.Layers[network.Layers.Length - 1].Neurons[j].LastNET); nablaBiases[network.Layers.Length - 1][j] += _config.LearningRate * network.Layers[network.Layers.Length - 1].Neurons[j].dEdz; for (int i = 0; i < network.Layers[network.Layers.Length - 1].Neurons[j].Weights.Length; i++) { nablaWeights[network.Layers.Length - 1][j][i] += _config.LearningRate*(network.Layers[network.Layers.Length - 1].Neurons[j].dEdz* (network.Layers.Length > 1 ? network.Layers[network.Layers.Length - 1 - 1].Neurons[i].LastState : data[inBatchIndex].Input[i]) + _config.RegularizationFactor * network.Layers[network.Layers.Length - 1].Neurons[j].Weights[i] / data.Count); } }
:
"", ( , ) dE/dz, ,
//hidden layers for (int hiddenLayerIndex = network.Layers.Length - 2; hiddenLayerIndex >= 0; hiddenLayerIndex--) { for (int j = 0; j < network.Layers[hiddenLayerIndex].Neurons.Length; j++) { network.Layers[hiddenLayerIndex].Neurons[j].dEdz = 0; for (int k = 0; k < network.Layers[hiddenLayerIndex + 1].Neurons.Length; k++) { network.Layers[hiddenLayerIndex].Neurons[j].dEdz += network.Layers[hiddenLayerIndex + 1].Neurons[k].Weights[j]* network.Layers[hiddenLayerIndex + 1].Neurons[k].dEdz; } network.Layers[hiddenLayerIndex].Neurons[j].dEdz *= network.Layers[hiddenLayerIndex].Neurons[j].ActivationFunction. ComputeFirstDerivative( network.Layers[hiddenLayerIndex].Neurons[j].LastNET ); nablaBiases[hiddenLayerIndex][j] += _config.LearningRate* network.Layers[hiddenLayerIndex].Neurons[j].dEdz; for (int i = 0; i < network.Layers[hiddenLayerIndex].Neurons[j].Weights.Length; i++) { nablaWeights[hiddenLayerIndex][j][i] += _config.LearningRate * ( network.Layers[hiddenLayerIndex].Neurons[j].dEdz * (hiddenLayerIndex > 0 ? network.Layers[hiddenLayerIndex - 1].Neurons[i].LastState : data[inBatchIndex].Input[i]) + _config.RegularizationFactor * network.Layers[hiddenLayerIndex].Neurons[j].Weights[i] / data.Count ); } } }
, ( ), :
//recalculating error on all data //real error currentError = 0; for (int i = 0; i < data.Count; i++) { double[] realOutput = network.ComputeOutput(data[i].Input); currentError += _config.ErrorFunction.Calculate(data[i].Output, realOutput); } currentError *= 1d/data.Count; //regularization term if (Math.Abs(_config.RegularizationFactor - 0d) > Double.Epsilon) { double reg = 0; for (int layerIndex = 0; layerIndex < network.Layers.Length; layerIndex++) { for (int neuronIndex = 0; neuronIndex < network.Layers[layerIndex].Neurons.Length; neuronIndex++) { for (int weightIndex = 0; weightIndex < network.Layers[layerIndex].Neurons[neuronIndex].Weights.Length; weightIndex++) { reg += network.Layers[layerIndex].Neurons[neuronIndex].Weights[weightIndex] * network.Layers[layerIndex].Neurons[neuronIndex].Weights[weightIndex]; } } } currentError += _config.RegularizationFactor * reg / (2 * data.Count); } epochNumber++; Logger.Instance.Log("Eposh #" + epochNumber.ToString() + " finished; current error is " + currentError.ToString() + "; it takes: " + (DateTime.Now - dtStart).Duration().ToString());
, :
} while (epochNumber < _config.MaxEpoches && currentError > _config.MinError && Math.Abs(currentError - lastError) > _config.MinErrorChange);
. , , , . . , , , , , .
:

:
.
, , , , . -)
internal class BackpropagationFCNLearningAlgorithm : ILearningStrategy, public void Train(IMultilayerNeuralNetwork network, IList<DataItem> data).
( ) :
if (_config.BatchSize < 1 || _config.BatchSize > data.Count) { _config.BatchSize = data.Count; } double currentError = Single.MaxValue; double lastError = 0; int epochNumber = 0; Logger.Instance.Log("Start learning...");
, , :
do { //... } while (epochNumber < _config.MaxEpoches && currentError > _config.MinError && Math.Abs(currentError - lastError) > _config.MinErrorChange);
, , . , batch , .
lastError = currentError; DateTime dtStart = DateTime.Now; //preparation for epoche int[] trainingIndices = new int[data.Count]; for (int i = 0; i < data.Count; i++) { trainingIndices[i] = i; } if (_config.BatchSize > 0) { trainingIndices = Shuffle(trainingIndices); }
, , , :
//process data set int currentIndex = 0; do { #region initialize accumulated error for batch, for weights and biases double[][][] nablaWeights = new double[network.Layers.Length][][]; double[][] nablaBiases = new double[network.Layers.Length][]; for (int i = 0; i < network.Layers.Length; i++) { nablaBiases[i] = new double[network.Layers[i].Neurons.Length]; nablaWeights[i] = new double[network.Layers[i].Neurons.Length][]; for (int j = 0; j < network.Layers[i].Neurons.Length; j++) { nablaBiases[i][j] = 0; nablaWeights[i][j] = new double[network.Layers[i].Neurons[j].Weights.Length]; for (int k = 0; k < network.Layers[i].Neurons[j].Weights.Length; k++) { nablaWeights[i][j][k] = 0; } } } #endregion //process one batch for (int inBatchIndex = currentIndex; inBatchIndex < currentIndex + _config.BatchSize && inBatchIndex < data.Count; inBatchIndex++) { //forward pass double[] realOutput = network.ComputeOutput(data[trainingIndices[inBatchIndex]].Input); //backward pass, error propagation //last layer //....................................... //hidden layers //....................................... } //update weights and bias for (int layerIndex = 0; layerIndex < network.Layers.Length; layerIndex++) { for (int neuronIndex = 0; neuronIndex < network.Layers[layerIndex].Neurons.Length; neuronIndex++) { network.Layers[layerIndex].Neurons[neuronIndex].Bias -= nablaBiases[layerIndex][neuronIndex]; for (int weightIndex = 0; weightIndex < network.Layers[layerIndex].Neurons[neuronIndex].Weights.Length; weightIndex++) { network.Layers[layerIndex].Neurons[neuronIndex].Weights[weightIndex] -= nablaWeights[layerIndex][neuronIndex][weightIndex]; } } } currentIndex += _config.BatchSize; } while (currentIndex < data.Count);
:
"", ( , ) dE/dz
//last layer for (int j = 0; j < network.Layers[network.Layers.Length - 1].Neurons.Length; j++) { network.Layers[network.Layers.Length - 1].Neurons[j].dEdz = _config.ErrorFunction.CalculatePartialDerivaitveByV2Index(data[inBatchIndex].Output, realOutput, j) * network.Layers[network.Layers.Length - 1].Neurons[j].ActivationFunction. ComputeFirstDerivative(network.Layers[network.Layers.Length - 1].Neurons[j].LastNET); nablaBiases[network.Layers.Length - 1][j] += _config.LearningRate * network.Layers[network.Layers.Length - 1].Neurons[j].dEdz; for (int i = 0; i < network.Layers[network.Layers.Length - 1].Neurons[j].Weights.Length; i++) { nablaWeights[network.Layers.Length - 1][j][i] += _config.LearningRate*(network.Layers[network.Layers.Length - 1].Neurons[j].dEdz* (network.Layers.Length > 1 ? network.Layers[network.Layers.Length - 1 - 1].Neurons[i].LastState : data[inBatchIndex].Input[i]) + _config.RegularizationFactor * network.Layers[network.Layers.Length - 1].Neurons[j].Weights[i] / data.Count); } }
:
"", ( , ) dE/dz, ,
//hidden layers for (int hiddenLayerIndex = network.Layers.Length - 2; hiddenLayerIndex >= 0; hiddenLayerIndex--) { for (int j = 0; j < network.Layers[hiddenLayerIndex].Neurons.Length; j++) { network.Layers[hiddenLayerIndex].Neurons[j].dEdz = 0; for (int k = 0; k < network.Layers[hiddenLayerIndex + 1].Neurons.Length; k++) { network.Layers[hiddenLayerIndex].Neurons[j].dEdz += network.Layers[hiddenLayerIndex + 1].Neurons[k].Weights[j]* network.Layers[hiddenLayerIndex + 1].Neurons[k].dEdz; } network.Layers[hiddenLayerIndex].Neurons[j].dEdz *= network.Layers[hiddenLayerIndex].Neurons[j].ActivationFunction. ComputeFirstDerivative( network.Layers[hiddenLayerIndex].Neurons[j].LastNET ); nablaBiases[hiddenLayerIndex][j] += _config.LearningRate* network.Layers[hiddenLayerIndex].Neurons[j].dEdz; for (int i = 0; i < network.Layers[hiddenLayerIndex].Neurons[j].Weights.Length; i++) { nablaWeights[hiddenLayerIndex][j][i] += _config.LearningRate * ( network.Layers[hiddenLayerIndex].Neurons[j].dEdz * (hiddenLayerIndex > 0 ? network.Layers[hiddenLayerIndex - 1].Neurons[i].LastState : data[inBatchIndex].Input[i]) + _config.RegularizationFactor * network.Layers[hiddenLayerIndex].Neurons[j].Weights[i] / data.Count ); } } }
, ( ), :
//recalculating error on all data //real error currentError = 0; for (int i = 0; i < data.Count; i++) { double[] realOutput = network.ComputeOutput(data[i].Input); currentError += _config.ErrorFunction.Calculate(data[i].Output, realOutput); } currentError *= 1d/data.Count; //regularization term if (Math.Abs(_config.RegularizationFactor - 0d) > Double.Epsilon) { double reg = 0; for (int layerIndex = 0; layerIndex < network.Layers.Length; layerIndex++) { for (int neuronIndex = 0; neuronIndex < network.Layers[layerIndex].Neurons.Length; neuronIndex++) { for (int weightIndex = 0; weightIndex < network.Layers[layerIndex].Neurons[neuronIndex].Weights.Length; weightIndex++) { reg += network.Layers[layerIndex].Neurons[neuronIndex].Weights[weightIndex] * network.Layers[layerIndex].Neurons[neuronIndex].Weights[weightIndex]; } } } currentError += _config.RegularizationFactor * reg / (2 * data.Count); } epochNumber++; Logger.Instance.Log("Eposh #" + epochNumber.ToString() + " finished; current error is " + currentError.ToString() + "; it takes: " + (DateTime.Now - dtStart).Duration().ToString());
, :
} while (epochNumber < _config.MaxEpoches && currentError > _config.MinError && Math.Abs(currentError - lastError) > _config.MinErrorChange);
. , , , . . , , , , , .
:

:
.
, , , , . -)
internal class BackpropagationFCNLearningAlgorithm : ILearningStrategy, public void Train(IMultilayerNeuralNetwork network, IList<DataItem> data).
( ) :
if (_config.BatchSize < 1 || _config.BatchSize > data.Count) { _config.BatchSize = data.Count; } double currentError = Single.MaxValue; double lastError = 0; int epochNumber = 0; Logger.Instance.Log("Start learning...");
, , :
do { //... } while (epochNumber < _config.MaxEpoches && currentError > _config.MinError && Math.Abs(currentError - lastError) > _config.MinErrorChange);
, , . , batch , .
lastError = currentError; DateTime dtStart = DateTime.Now; //preparation for epoche int[] trainingIndices = new int[data.Count]; for (int i = 0; i < data.Count; i++) { trainingIndices[i] = i; } if (_config.BatchSize > 0) { trainingIndices = Shuffle(trainingIndices); }
, , , :
//process data set int currentIndex = 0; do { #region initialize accumulated error for batch, for weights and biases double[][][] nablaWeights = new double[network.Layers.Length][][]; double[][] nablaBiases = new double[network.Layers.Length][]; for (int i = 0; i < network.Layers.Length; i++) { nablaBiases[i] = new double[network.Layers[i].Neurons.Length]; nablaWeights[i] = new double[network.Layers[i].Neurons.Length][]; for (int j = 0; j < network.Layers[i].Neurons.Length; j++) { nablaBiases[i][j] = 0; nablaWeights[i][j] = new double[network.Layers[i].Neurons[j].Weights.Length]; for (int k = 0; k < network.Layers[i].Neurons[j].Weights.Length; k++) { nablaWeights[i][j][k] = 0; } } } #endregion //process one batch for (int inBatchIndex = currentIndex; inBatchIndex < currentIndex + _config.BatchSize && inBatchIndex < data.Count; inBatchIndex++) { //forward pass double[] realOutput = network.ComputeOutput(data[trainingIndices[inBatchIndex]].Input); //backward pass, error propagation //last layer //....................................... //hidden layers //....................................... } //update weights and bias for (int layerIndex = 0; layerIndex < network.Layers.Length; layerIndex++) { for (int neuronIndex = 0; neuronIndex < network.Layers[layerIndex].Neurons.Length; neuronIndex++) { network.Layers[layerIndex].Neurons[neuronIndex].Bias -= nablaBiases[layerIndex][neuronIndex]; for (int weightIndex = 0; weightIndex < network.Layers[layerIndex].Neurons[neuronIndex].Weights.Length; weightIndex++) { network.Layers[layerIndex].Neurons[neuronIndex].Weights[weightIndex] -= nablaWeights[layerIndex][neuronIndex][weightIndex]; } } } currentIndex += _config.BatchSize; } while (currentIndex < data.Count);
:
"", ( , ) dE/dz
//last layer for (int j = 0; j < network.Layers[network.Layers.Length - 1].Neurons.Length; j++) { network.Layers[network.Layers.Length - 1].Neurons[j].dEdz = _config.ErrorFunction.CalculatePartialDerivaitveByV2Index(data[inBatchIndex].Output, realOutput, j) * network.Layers[network.Layers.Length - 1].Neurons[j].ActivationFunction. ComputeFirstDerivative(network.Layers[network.Layers.Length - 1].Neurons[j].LastNET); nablaBiases[network.Layers.Length - 1][j] += _config.LearningRate * network.Layers[network.Layers.Length - 1].Neurons[j].dEdz; for (int i = 0; i < network.Layers[network.Layers.Length - 1].Neurons[j].Weights.Length; i++) { nablaWeights[network.Layers.Length - 1][j][i] += _config.LearningRate*(network.Layers[network.Layers.Length - 1].Neurons[j].dEdz* (network.Layers.Length > 1 ? network.Layers[network.Layers.Length - 1 - 1].Neurons[i].LastState : data[inBatchIndex].Input[i]) + _config.RegularizationFactor * network.Layers[network.Layers.Length - 1].Neurons[j].Weights[i] / data.Count); } }
:
"", ( , ) dE/dz, ,
//hidden layers for (int hiddenLayerIndex = network.Layers.Length - 2; hiddenLayerIndex >= 0; hiddenLayerIndex--) { for (int j = 0; j < network.Layers[hiddenLayerIndex].Neurons.Length; j++) { network.Layers[hiddenLayerIndex].Neurons[j].dEdz = 0; for (int k = 0; k < network.Layers[hiddenLayerIndex + 1].Neurons.Length; k++) { network.Layers[hiddenLayerIndex].Neurons[j].dEdz += network.Layers[hiddenLayerIndex + 1].Neurons[k].Weights[j]* network.Layers[hiddenLayerIndex + 1].Neurons[k].dEdz; } network.Layers[hiddenLayerIndex].Neurons[j].dEdz *= network.Layers[hiddenLayerIndex].Neurons[j].ActivationFunction. ComputeFirstDerivative( network.Layers[hiddenLayerIndex].Neurons[j].LastNET ); nablaBiases[hiddenLayerIndex][j] += _config.LearningRate* network.Layers[hiddenLayerIndex].Neurons[j].dEdz; for (int i = 0; i < network.Layers[hiddenLayerIndex].Neurons[j].Weights.Length; i++) { nablaWeights[hiddenLayerIndex][j][i] += _config.LearningRate * ( network.Layers[hiddenLayerIndex].Neurons[j].dEdz * (hiddenLayerIndex > 0 ? network.Layers[hiddenLayerIndex - 1].Neurons[i].LastState : data[inBatchIndex].Input[i]) + _config.RegularizationFactor * network.Layers[hiddenLayerIndex].Neurons[j].Weights[i] / data.Count ); } } }
, ( ), :
//recalculating error on all data //real error currentError = 0; for (int i = 0; i < data.Count; i++) { double[] realOutput = network.ComputeOutput(data[i].Input); currentError += _config.ErrorFunction.Calculate(data[i].Output, realOutput); } currentError *= 1d/data.Count; //regularization term if (Math.Abs(_config.RegularizationFactor - 0d) > Double.Epsilon) { double reg = 0; for (int layerIndex = 0; layerIndex < network.Layers.Length; layerIndex++) { for (int neuronIndex = 0; neuronIndex < network.Layers[layerIndex].Neurons.Length; neuronIndex++) { for (int weightIndex = 0; weightIndex < network.Layers[layerIndex].Neurons[neuronIndex].Weights.Length; weightIndex++) { reg += network.Layers[layerIndex].Neurons[neuronIndex].Weights[weightIndex] * network.Layers[layerIndex].Neurons[neuronIndex].Weights[weightIndex]; } } } currentError += _config.RegularizationFactor * reg / (2 * data.Count); } epochNumber++; Logger.Instance.Log("Eposh #" + epochNumber.ToString() + " finished; current error is " + currentError.ToString() + "; it takes: " + (DateTime.Now - dtStart).Duration().ToString());
, :
} while (epochNumber < _config.MaxEpoches && currentError > _config.MinError && Math.Abs(currentError - lastError) > _config.MinErrorChange);
. , , , . . , , , , , .
:

:
.
, , , , . -)
internal class BackpropagationFCNLearningAlgorithm : ILearningStrategy, public void Train(IMultilayerNeuralNetwork network, IList<DataItem> data).
( ) :
if (_config.BatchSize < 1 || _config.BatchSize > data.Count) { _config.BatchSize = data.Count; } double currentError = Single.MaxValue; double lastError = 0; int epochNumber = 0; Logger.Instance.Log("Start learning...");
, , :
do { //... } while (epochNumber < _config.MaxEpoches && currentError > _config.MinError && Math.Abs(currentError - lastError) > _config.MinErrorChange);
, , . , batch , .
lastError = currentError; DateTime dtStart = DateTime.Now; //preparation for epoche int[] trainingIndices = new int[data.Count]; for (int i = 0; i < data.Count; i++) { trainingIndices[i] = i; } if (_config.BatchSize > 0) { trainingIndices = Shuffle(trainingIndices); }
, , , :
//process data set int currentIndex = 0; do { #region initialize accumulated error for batch, for weights and biases double[][][] nablaWeights = new double[network.Layers.Length][][]; double[][] nablaBiases = new double[network.Layers.Length][]; for (int i = 0; i < network.Layers.Length; i++) { nablaBiases[i] = new double[network.Layers[i].Neurons.Length]; nablaWeights[i] = new double[network.Layers[i].Neurons.Length][]; for (int j = 0; j < network.Layers[i].Neurons.Length; j++) { nablaBiases[i][j] = 0; nablaWeights[i][j] = new double[network.Layers[i].Neurons[j].Weights.Length]; for (int k = 0; k < network.Layers[i].Neurons[j].Weights.Length; k++) { nablaWeights[i][j][k] = 0; } } } #endregion //process one batch for (int inBatchIndex = currentIndex; inBatchIndex < currentIndex + _config.BatchSize && inBatchIndex < data.Count; inBatchIndex++) { //forward pass double[] realOutput = network.ComputeOutput(data[trainingIndices[inBatchIndex]].Input); //backward pass, error propagation //last layer //....................................... //hidden layers //....................................... } //update weights and bias for (int layerIndex = 0; layerIndex < network.Layers.Length; layerIndex++) { for (int neuronIndex = 0; neuronIndex < network.Layers[layerIndex].Neurons.Length; neuronIndex++) { network.Layers[layerIndex].Neurons[neuronIndex].Bias -= nablaBiases[layerIndex][neuronIndex]; for (int weightIndex = 0; weightIndex < network.Layers[layerIndex].Neurons[neuronIndex].Weights.Length; weightIndex++) { network.Layers[layerIndex].Neurons[neuronIndex].Weights[weightIndex] -= nablaWeights[layerIndex][neuronIndex][weightIndex]; } } } currentIndex += _config.BatchSize; } while (currentIndex < data.Count);
:
"", ( , ) dE/dz
//last layer for (int j = 0; j < network.Layers[network.Layers.Length - 1].Neurons.Length; j++) { network.Layers[network.Layers.Length - 1].Neurons[j].dEdz = _config.ErrorFunction.CalculatePartialDerivaitveByV2Index(data[inBatchIndex].Output, realOutput, j) * network.Layers[network.Layers.Length - 1].Neurons[j].ActivationFunction. ComputeFirstDerivative(network.Layers[network.Layers.Length - 1].Neurons[j].LastNET); nablaBiases[network.Layers.Length - 1][j] += _config.LearningRate * network.Layers[network.Layers.Length - 1].Neurons[j].dEdz; for (int i = 0; i < network.Layers[network.Layers.Length - 1].Neurons[j].Weights.Length; i++) { nablaWeights[network.Layers.Length - 1][j][i] += _config.LearningRate*(network.Layers[network.Layers.Length - 1].Neurons[j].dEdz* (network.Layers.Length > 1 ? network.Layers[network.Layers.Length - 1 - 1].Neurons[i].LastState : data[inBatchIndex].Input[i]) + _config.RegularizationFactor * network.Layers[network.Layers.Length - 1].Neurons[j].Weights[i] / data.Count); } }
:
"", ( , ) dE/dz, ,
//hidden layers for (int hiddenLayerIndex = network.Layers.Length - 2; hiddenLayerIndex >= 0; hiddenLayerIndex--) { for (int j = 0; j < network.Layers[hiddenLayerIndex].Neurons.Length; j++) { network.Layers[hiddenLayerIndex].Neurons[j].dEdz = 0; for (int k = 0; k < network.Layers[hiddenLayerIndex + 1].Neurons.Length; k++) { network.Layers[hiddenLayerIndex].Neurons[j].dEdz += network.Layers[hiddenLayerIndex + 1].Neurons[k].Weights[j]* network.Layers[hiddenLayerIndex + 1].Neurons[k].dEdz; } network.Layers[hiddenLayerIndex].Neurons[j].dEdz *= network.Layers[hiddenLayerIndex].Neurons[j].ActivationFunction. ComputeFirstDerivative( network.Layers[hiddenLayerIndex].Neurons[j].LastNET ); nablaBiases[hiddenLayerIndex][j] += _config.LearningRate* network.Layers[hiddenLayerIndex].Neurons[j].dEdz; for (int i = 0; i < network.Layers[hiddenLayerIndex].Neurons[j].Weights.Length; i++) { nablaWeights[hiddenLayerIndex][j][i] += _config.LearningRate * ( network.Layers[hiddenLayerIndex].Neurons[j].dEdz * (hiddenLayerIndex > 0 ? network.Layers[hiddenLayerIndex - 1].Neurons[i].LastState : data[inBatchIndex].Input[i]) + _config.RegularizationFactor * network.Layers[hiddenLayerIndex].Neurons[j].Weights[i] / data.Count ); } } }
, ( ), :
//recalculating error on all data //real error currentError = 0; for (int i = 0; i < data.Count; i++) { double[] realOutput = network.ComputeOutput(data[i].Input); currentError += _config.ErrorFunction.Calculate(data[i].Output, realOutput); } currentError *= 1d/data.Count; //regularization term if (Math.Abs(_config.RegularizationFactor - 0d) > Double.Epsilon) { double reg = 0; for (int layerIndex = 0; layerIndex < network.Layers.Length; layerIndex++) { for (int neuronIndex = 0; neuronIndex < network.Layers[layerIndex].Neurons.Length; neuronIndex++) { for (int weightIndex = 0; weightIndex < network.Layers[layerIndex].Neurons[neuronIndex].Weights.Length; weightIndex++) { reg += network.Layers[layerIndex].Neurons[neuronIndex].Weights[weightIndex] * network.Layers[layerIndex].Neurons[neuronIndex].Weights[weightIndex]; } } } currentError += _config.RegularizationFactor * reg / (2 * data.Count); } epochNumber++; Logger.Instance.Log("Eposh #" + epochNumber.ToString() + " finished; current error is " + currentError.ToString() + "; it takes: " + (DateTime.Now - dtStart).Duration().ToString());
, :
} while (epochNumber < _config.MaxEpoches && currentError > _config.MinError && Math.Abs(currentError - lastError) > _config.MinErrorChange);
. , , , . . , , , , , .
:

:
.
, , , , . -)
internal class BackpropagationFCNLearningAlgorithm : ILearningStrategy, public void Train(IMultilayerNeuralNetwork network, IList<DataItem> data).
( ) :
if (_config.BatchSize < 1 || _config.BatchSize > data.Count) { _config.BatchSize = data.Count; } double currentError = Single.MaxValue; double lastError = 0; int epochNumber = 0; Logger.Instance.Log("Start learning...");
, , :
do { //... } while (epochNumber < _config.MaxEpoches && currentError > _config.MinError && Math.Abs(currentError - lastError) > _config.MinErrorChange);
, , . , batch , .
lastError = currentError; DateTime dtStart = DateTime.Now; //preparation for epoche int[] trainingIndices = new int[data.Count]; for (int i = 0; i < data.Count; i++) { trainingIndices[i] = i; } if (_config.BatchSize > 0) { trainingIndices = Shuffle(trainingIndices); }
, , , :
//process data set int currentIndex = 0; do { #region initialize accumulated error for batch, for weights and biases double[][][] nablaWeights = new double[network.Layers.Length][][]; double[][] nablaBiases = new double[network.Layers.Length][]; for (int i = 0; i < network.Layers.Length; i++) { nablaBiases[i] = new double[network.Layers[i].Neurons.Length]; nablaWeights[i] = new double[network.Layers[i].Neurons.Length][]; for (int j = 0; j < network.Layers[i].Neurons.Length; j++) { nablaBiases[i][j] = 0; nablaWeights[i][j] = new double[network.Layers[i].Neurons[j].Weights.Length]; for (int k = 0; k < network.Layers[i].Neurons[j].Weights.Length; k++) { nablaWeights[i][j][k] = 0; } } } #endregion //process one batch for (int inBatchIndex = currentIndex; inBatchIndex < currentIndex + _config.BatchSize && inBatchIndex < data.Count; inBatchIndex++) { //forward pass double[] realOutput = network.ComputeOutput(data[trainingIndices[inBatchIndex]].Input); //backward pass, error propagation //last layer //....................................... //hidden layers //....................................... } //update weights and bias for (int layerIndex = 0; layerIndex < network.Layers.Length; layerIndex++) { for (int neuronIndex = 0; neuronIndex < network.Layers[layerIndex].Neurons.Length; neuronIndex++) { network.Layers[layerIndex].Neurons[neuronIndex].Bias -= nablaBiases[layerIndex][neuronIndex]; for (int weightIndex = 0; weightIndex < network.Layers[layerIndex].Neurons[neuronIndex].Weights.Length; weightIndex++) { network.Layers[layerIndex].Neurons[neuronIndex].Weights[weightIndex] -= nablaWeights[layerIndex][neuronIndex][weightIndex]; } } } currentIndex += _config.BatchSize; } while (currentIndex < data.Count);
:
"", ( , ) dE/dz
//last layer for (int j = 0; j < network.Layers[network.Layers.Length - 1].Neurons.Length; j++) { network.Layers[network.Layers.Length - 1].Neurons[j].dEdz = _config.ErrorFunction.CalculatePartialDerivaitveByV2Index(data[inBatchIndex].Output, realOutput, j) * network.Layers[network.Layers.Length - 1].Neurons[j].ActivationFunction. ComputeFirstDerivative(network.Layers[network.Layers.Length - 1].Neurons[j].LastNET); nablaBiases[network.Layers.Length - 1][j] += _config.LearningRate * network.Layers[network.Layers.Length - 1].Neurons[j].dEdz; for (int i = 0; i < network.Layers[network.Layers.Length - 1].Neurons[j].Weights.Length; i++) { nablaWeights[network.Layers.Length - 1][j][i] += _config.LearningRate*(network.Layers[network.Layers.Length - 1].Neurons[j].dEdz* (network.Layers.Length > 1 ? network.Layers[network.Layers.Length - 1 - 1].Neurons[i].LastState : data[inBatchIndex].Input[i]) + _config.RegularizationFactor * network.Layers[network.Layers.Length - 1].Neurons[j].Weights[i] / data.Count); } }
:
"", ( , ) dE/dz, ,
//hidden layers for (int hiddenLayerIndex = network.Layers.Length - 2; hiddenLayerIndex >= 0; hiddenLayerIndex--) { for (int j = 0; j < network.Layers[hiddenLayerIndex].Neurons.Length; j++) { network.Layers[hiddenLayerIndex].Neurons[j].dEdz = 0; for (int k = 0; k < network.Layers[hiddenLayerIndex + 1].Neurons.Length; k++) { network.Layers[hiddenLayerIndex].Neurons[j].dEdz += network.Layers[hiddenLayerIndex + 1].Neurons[k].Weights[j]* network.Layers[hiddenLayerIndex + 1].Neurons[k].dEdz; } network.Layers[hiddenLayerIndex].Neurons[j].dEdz *= network.Layers[hiddenLayerIndex].Neurons[j].ActivationFunction. ComputeFirstDerivative( network.Layers[hiddenLayerIndex].Neurons[j].LastNET ); nablaBiases[hiddenLayerIndex][j] += _config.LearningRate* network.Layers[hiddenLayerIndex].Neurons[j].dEdz; for (int i = 0; i < network.Layers[hiddenLayerIndex].Neurons[j].Weights.Length; i++) { nablaWeights[hiddenLayerIndex][j][i] += _config.LearningRate * ( network.Layers[hiddenLayerIndex].Neurons[j].dEdz * (hiddenLayerIndex > 0 ? network.Layers[hiddenLayerIndex - 1].Neurons[i].LastState : data[inBatchIndex].Input[i]) + _config.RegularizationFactor * network.Layers[hiddenLayerIndex].Neurons[j].Weights[i] / data.Count ); } } }
, ( ), :
//recalculating error on all data //real error currentError = 0; for (int i = 0; i < data.Count; i++) { double[] realOutput = network.ComputeOutput(data[i].Input); currentError += _config.ErrorFunction.Calculate(data[i].Output, realOutput); } currentError *= 1d/data.Count; //regularization term if (Math.Abs(_config.RegularizationFactor - 0d) > Double.Epsilon) { double reg = 0; for (int layerIndex = 0; layerIndex < network.Layers.Length; layerIndex++) { for (int neuronIndex = 0; neuronIndex < network.Layers[layerIndex].Neurons.Length; neuronIndex++) { for (int weightIndex = 0; weightIndex < network.Layers[layerIndex].Neurons[neuronIndex].Weights.Length; weightIndex++) { reg += network.Layers[layerIndex].Neurons[neuronIndex].Weights[weightIndex] * network.Layers[layerIndex].Neurons[neuronIndex].Weights[weightIndex]; } } } currentError += _config.RegularizationFactor * reg / (2 * data.Count); } epochNumber++; Logger.Instance.Log("Eposh #" + epochNumber.ToString() + " finished; current error is " + currentError.ToString() + "; it takes: " + (DateTime.Now - dtStart).Duration().ToString());
, :
} while (epochNumber < _config.MaxEpoches && currentError > _config.MinError && Math.Abs(currentError - lastError) > _config.MinErrorChange);
. , , , . . , , , , , .
:

:
.
, , , , . -)
internal class BackpropagationFCNLearningAlgorithm : ILearningStrategy, public void Train(IMultilayerNeuralNetwork network, IList<DataItem> data).
( ) :
if (_config.BatchSize < 1 || _config.BatchSize > data.Count) { _config.BatchSize = data.Count; } double currentError = Single.MaxValue; double lastError = 0; int epochNumber = 0; Logger.Instance.Log("Start learning...");
, , :
do { //... } while (epochNumber < _config.MaxEpoches && currentError > _config.MinError && Math.Abs(currentError - lastError) > _config.MinErrorChange);
, , . , batch , .
lastError = currentError; DateTime dtStart = DateTime.Now; //preparation for epoche int[] trainingIndices = new int[data.Count]; for (int i = 0; i < data.Count; i++) { trainingIndices[i] = i; } if (_config.BatchSize > 0) { trainingIndices = Shuffle(trainingIndices); }
, , , :
//process data set int currentIndex = 0; do { #region initialize accumulated error for batch, for weights and biases double[][][] nablaWeights = new double[network.Layers.Length][][]; double[][] nablaBiases = new double[network.Layers.Length][]; for (int i = 0; i < network.Layers.Length; i++) { nablaBiases[i] = new double[network.Layers[i].Neurons.Length]; nablaWeights[i] = new double[network.Layers[i].Neurons.Length][]; for (int j = 0; j < network.Layers[i].Neurons.Length; j++) { nablaBiases[i][j] = 0; nablaWeights[i][j] = new double[network.Layers[i].Neurons[j].Weights.Length]; for (int k = 0; k < network.Layers[i].Neurons[j].Weights.Length; k++) { nablaWeights[i][j][k] = 0; } } } #endregion //process one batch for (int inBatchIndex = currentIndex; inBatchIndex < currentIndex + _config.BatchSize && inBatchIndex < data.Count; inBatchIndex++) { //forward pass double[] realOutput = network.ComputeOutput(data[trainingIndices[inBatchIndex]].Input); //backward pass, error propagation //last layer //....................................... //hidden layers //....................................... } //update weights and bias for (int layerIndex = 0; layerIndex < network.Layers.Length; layerIndex++) { for (int neuronIndex = 0; neuronIndex < network.Layers[layerIndex].Neurons.Length; neuronIndex++) { network.Layers[layerIndex].Neurons[neuronIndex].Bias -= nablaBiases[layerIndex][neuronIndex]; for (int weightIndex = 0; weightIndex < network.Layers[layerIndex].Neurons[neuronIndex].Weights.Length; weightIndex++) { network.Layers[layerIndex].Neurons[neuronIndex].Weights[weightIndex] -= nablaWeights[layerIndex][neuronIndex][weightIndex]; } } } currentIndex += _config.BatchSize; } while (currentIndex < data.Count);
:
"", ( , ) dE/dz
//last layer for (int j = 0; j < network.Layers[network.Layers.Length - 1].Neurons.Length; j++) { network.Layers[network.Layers.Length - 1].Neurons[j].dEdz = _config.ErrorFunction.CalculatePartialDerivaitveByV2Index(data[inBatchIndex].Output, realOutput, j) * network.Layers[network.Layers.Length - 1].Neurons[j].ActivationFunction. ComputeFirstDerivative(network.Layers[network.Layers.Length - 1].Neurons[j].LastNET); nablaBiases[network.Layers.Length - 1][j] += _config.LearningRate * network.Layers[network.Layers.Length - 1].Neurons[j].dEdz; for (int i = 0; i < network.Layers[network.Layers.Length - 1].Neurons[j].Weights.Length; i++) { nablaWeights[network.Layers.Length - 1][j][i] += _config.LearningRate*(network.Layers[network.Layers.Length - 1].Neurons[j].dEdz* (network.Layers.Length > 1 ? network.Layers[network.Layers.Length - 1 - 1].Neurons[i].LastState : data[inBatchIndex].Input[i]) + _config.RegularizationFactor * network.Layers[network.Layers.Length - 1].Neurons[j].Weights[i] / data.Count); } }
:
"", ( , ) dE/dz, ,
//hidden layers for (int hiddenLayerIndex = network.Layers.Length - 2; hiddenLayerIndex >= 0; hiddenLayerIndex--) { for (int j = 0; j < network.Layers[hiddenLayerIndex].Neurons.Length; j++) { network.Layers[hiddenLayerIndex].Neurons[j].dEdz = 0; for (int k = 0; k < network.Layers[hiddenLayerIndex + 1].Neurons.Length; k++) { network.Layers[hiddenLayerIndex].Neurons[j].dEdz += network.Layers[hiddenLayerIndex + 1].Neurons[k].Weights[j]* network.Layers[hiddenLayerIndex + 1].Neurons[k].dEdz; } network.Layers[hiddenLayerIndex].Neurons[j].dEdz *= network.Layers[hiddenLayerIndex].Neurons[j].ActivationFunction. ComputeFirstDerivative( network.Layers[hiddenLayerIndex].Neurons[j].LastNET ); nablaBiases[hiddenLayerIndex][j] += _config.LearningRate* network.Layers[hiddenLayerIndex].Neurons[j].dEdz; for (int i = 0; i < network.Layers[hiddenLayerIndex].Neurons[j].Weights.Length; i++) { nablaWeights[hiddenLayerIndex][j][i] += _config.LearningRate * ( network.Layers[hiddenLayerIndex].Neurons[j].dEdz * (hiddenLayerIndex > 0 ? network.Layers[hiddenLayerIndex - 1].Neurons[i].LastState : data[inBatchIndex].Input[i]) + _config.RegularizationFactor * network.Layers[hiddenLayerIndex].Neurons[j].Weights[i] / data.Count ); } } }
, ( ), :
//recalculating error on all data //real error currentError = 0; for (int i = 0; i < data.Count; i++) { double[] realOutput = network.ComputeOutput(data[i].Input); currentError += _config.ErrorFunction.Calculate(data[i].Output, realOutput); } currentError *= 1d/data.Count; //regularization term if (Math.Abs(_config.RegularizationFactor - 0d) > Double.Epsilon) { double reg = 0; for (int layerIndex = 0; layerIndex < network.Layers.Length; layerIndex++) { for (int neuronIndex = 0; neuronIndex < network.Layers[layerIndex].Neurons.Length; neuronIndex++) { for (int weightIndex = 0; weightIndex < network.Layers[layerIndex].Neurons[neuronIndex].Weights.Length; weightIndex++) { reg += network.Layers[layerIndex].Neurons[neuronIndex].Weights[weightIndex] * network.Layers[layerIndex].Neurons[neuronIndex].Weights[weightIndex]; } } } currentError += _config.RegularizationFactor * reg / (2 * data.Count); } epochNumber++; Logger.Instance.Log("Eposh #" + epochNumber.ToString() + " finished; current error is " + currentError.ToString() + "; it takes: " + (DateTime.Now - dtStart).Duration().ToString());
, :
} while (epochNumber < _config.MaxEpoches && currentError > _config.MinError && Math.Abs(currentError - lastError) > _config.MinErrorChange);
. , , , . . , , , , , .
:
:
.
, , , , . -)
internal class BackpropagationFCNLearningAlgorithm : ILearningStrategy, public void Train(IMultilayerNeuralNetwork network, IList<DataItem> data).
( ) :
if (_config.BatchSize < 1 || _config.BatchSize > data.Count) { _config.BatchSize = data.Count; } double currentError = Single.MaxValue; double lastError = 0; int epochNumber = 0; Logger.Instance.Log("Start learning...");
, , :
do { //... } while (epochNumber < _config.MaxEpoches && currentError > _config.MinError && Math.Abs(currentError - lastError) > _config.MinErrorChange);
, , . , batch , .
lastError = currentError; DateTime dtStart = DateTime.Now; //preparation for epoche int[] trainingIndices = new int[data.Count]; for (int i = 0; i < data.Count; i++) { trainingIndices[i] = i; } if (_config.BatchSize > 0) { trainingIndices = Shuffle(trainingIndices); }
, , , :
//process data set int currentIndex = 0; do { #region initialize accumulated error for batch, for weights and biases double[][][] nablaWeights = new double[network.Layers.Length][][]; double[][] nablaBiases = new double[network.Layers.Length][]; for (int i = 0; i < network.Layers.Length; i++) { nablaBiases[i] = new double[network.Layers[i].Neurons.Length]; nablaWeights[i] = new double[network.Layers[i].Neurons.Length][]; for (int j = 0; j < network.Layers[i].Neurons.Length; j++) { nablaBiases[i][j] = 0; nablaWeights[i][j] = new double[network.Layers[i].Neurons[j].Weights.Length]; for (int k = 0; k < network.Layers[i].Neurons[j].Weights.Length; k++) { nablaWeights[i][j][k] = 0; } } } #endregion //process one batch for (int inBatchIndex = currentIndex; inBatchIndex < currentIndex + _config.BatchSize && inBatchIndex < data.Count; inBatchIndex++) { //forward pass double[] realOutput = network.ComputeOutput(data[trainingIndices[inBatchIndex]].Input); //backward pass, error propagation //last layer //....................................... //hidden layers //....................................... } //update weights and bias for (int layerIndex = 0; layerIndex < network.Layers.Length; layerIndex++) { for (int neuronIndex = 0; neuronIndex < network.Layers[layerIndex].Neurons.Length; neuronIndex++) { network.Layers[layerIndex].Neurons[neuronIndex].Bias -= nablaBiases[layerIndex][neuronIndex]; for (int weightIndex = 0; weightIndex < network.Layers[layerIndex].Neurons[neuronIndex].Weights.Length; weightIndex++) { network.Layers[layerIndex].Neurons[neuronIndex].Weights[weightIndex] -= nablaWeights[layerIndex][neuronIndex][weightIndex]; } } } currentIndex += _config.BatchSize; } while (currentIndex < data.Count);
:
"", ( , ) dE/dz
//last layer for (int j = 0; j < network.Layers[network.Layers.Length - 1].Neurons.Length; j++) { network.Layers[network.Layers.Length - 1].Neurons[j].dEdz = _config.ErrorFunction.CalculatePartialDerivaitveByV2Index(data[inBatchIndex].Output, realOutput, j) * network.Layers[network.Layers.Length - 1].Neurons[j].ActivationFunction. ComputeFirstDerivative(network.Layers[network.Layers.Length - 1].Neurons[j].LastNET); nablaBiases[network.Layers.Length - 1][j] += _config.LearningRate * network.Layers[network.Layers.Length - 1].Neurons[j].dEdz; for (int i = 0; i < network.Layers[network.Layers.Length - 1].Neurons[j].Weights.Length; i++) { nablaWeights[network.Layers.Length - 1][j][i] += _config.LearningRate*(network.Layers[network.Layers.Length - 1].Neurons[j].dEdz* (network.Layers.Length > 1 ? network.Layers[network.Layers.Length - 1 - 1].Neurons[i].LastState : data[inBatchIndex].Input[i]) + _config.RegularizationFactor * network.Layers[network.Layers.Length - 1].Neurons[j].Weights[i] / data.Count); } }
:
"", ( , ) dE/dz, ,
//hidden layers for (int hiddenLayerIndex = network.Layers.Length - 2; hiddenLayerIndex >= 0; hiddenLayerIndex--) { for (int j = 0; j < network.Layers[hiddenLayerIndex].Neurons.Length; j++) { network.Layers[hiddenLayerIndex].Neurons[j].dEdz = 0; for (int k = 0; k < network.Layers[hiddenLayerIndex + 1].Neurons.Length; k++) { network.Layers[hiddenLayerIndex].Neurons[j].dEdz += network.Layers[hiddenLayerIndex + 1].Neurons[k].Weights[j]* network.Layers[hiddenLayerIndex + 1].Neurons[k].dEdz; } network.Layers[hiddenLayerIndex].Neurons[j].dEdz *= network.Layers[hiddenLayerIndex].Neurons[j].ActivationFunction. ComputeFirstDerivative( network.Layers[hiddenLayerIndex].Neurons[j].LastNET ); nablaBiases[hiddenLayerIndex][j] += _config.LearningRate* network.Layers[hiddenLayerIndex].Neurons[j].dEdz; for (int i = 0; i < network.Layers[hiddenLayerIndex].Neurons[j].Weights.Length; i++) { nablaWeights[hiddenLayerIndex][j][i] += _config.LearningRate * ( network.Layers[hiddenLayerIndex].Neurons[j].dEdz * (hiddenLayerIndex > 0 ? network.Layers[hiddenLayerIndex - 1].Neurons[i].LastState : data[inBatchIndex].Input[i]) + _config.RegularizationFactor * network.Layers[hiddenLayerIndex].Neurons[j].Weights[i] / data.Count ); } } }
, ( ), :
//recalculating error on all data //real error currentError = 0; for (int i = 0; i < data.Count; i++) { double[] realOutput = network.ComputeOutput(data[i].Input); currentError += _config.ErrorFunction.Calculate(data[i].Output, realOutput); } currentError *= 1d/data.Count; //regularization term if (Math.Abs(_config.RegularizationFactor - 0d) > Double.Epsilon) { double reg = 0; for (int layerIndex = 0; layerIndex < network.Layers.Length; layerIndex++) { for (int neuronIndex = 0; neuronIndex < network.Layers[layerIndex].Neurons.Length; neuronIndex++) { for (int weightIndex = 0; weightIndex < network.Layers[layerIndex].Neurons[neuronIndex].Weights.Length; weightIndex++) { reg += network.Layers[layerIndex].Neurons[neuronIndex].Weights[weightIndex] * network.Layers[layerIndex].Neurons[neuronIndex].Weights[weightIndex]; } } } currentError += _config.RegularizationFactor * reg / (2 * data.Count); } epochNumber++; Logger.Instance.Log("Eposh #" + epochNumber.ToString() + " finished; current error is " + currentError.ToString() + "; it takes: " + (DateTime.Now - dtStart).Duration().ToString());
, :
} while (epochNumber < _config.MaxEpoches && currentError > _config.MinError && Math.Abs(currentError - lastError) > _config.MinErrorChange);
. , , , . . , , , , , .
:

:
.
, , , , . -)
internal class BackpropagationFCNLearningAlgorithm : ILearningStrategy, public void Train(IMultilayerNeuralNetwork network, IList<DataItem> data).
( ) :
if (_config.BatchSize < 1 || _config.BatchSize > data.Count) { _config.BatchSize = data.Count; } double currentError = Single.MaxValue; double lastError = 0; int epochNumber = 0; Logger.Instance.Log("Start learning...");
, , :
do { //... } while (epochNumber < _config.MaxEpoches && currentError > _config.MinError && Math.Abs(currentError - lastError) > _config.MinErrorChange);
, , . , batch , .
lastError = currentError; DateTime dtStart = DateTime.Now; //preparation for epoche int[] trainingIndices = new int[data.Count]; for (int i = 0; i < data.Count; i++) { trainingIndices[i] = i; } if (_config.BatchSize > 0) { trainingIndices = Shuffle(trainingIndices); }
, , , :
//process data set int currentIndex = 0; do { #region initialize accumulated error for batch, for weights and biases double[][][] nablaWeights = new double[network.Layers.Length][][]; double[][] nablaBiases = new double[network.Layers.Length][]; for (int i = 0; i < network.Layers.Length; i++) { nablaBiases[i] = new double[network.Layers[i].Neurons.Length]; nablaWeights[i] = new double[network.Layers[i].Neurons.Length][]; for (int j = 0; j < network.Layers[i].Neurons.Length; j++) { nablaBiases[i][j] = 0; nablaWeights[i][j] = new double[network.Layers[i].Neurons[j].Weights.Length]; for (int k = 0; k < network.Layers[i].Neurons[j].Weights.Length; k++) { nablaWeights[i][j][k] = 0; } } } #endregion //process one batch for (int inBatchIndex = currentIndex; inBatchIndex < currentIndex + _config.BatchSize && inBatchIndex < data.Count; inBatchIndex++) { //forward pass double[] realOutput = network.ComputeOutput(data[trainingIndices[inBatchIndex]].Input); //backward pass, error propagation //last layer //....................................... //hidden layers //....................................... } //update weights and bias for (int layerIndex = 0; layerIndex < network.Layers.Length; layerIndex++) { for (int neuronIndex = 0; neuronIndex < network.Layers[layerIndex].Neurons.Length; neuronIndex++) { network.Layers[layerIndex].Neurons[neuronIndex].Bias -= nablaBiases[layerIndex][neuronIndex]; for (int weightIndex = 0; weightIndex < network.Layers[layerIndex].Neurons[neuronIndex].Weights.Length; weightIndex++) { network.Layers[layerIndex].Neurons[neuronIndex].Weights[weightIndex] -= nablaWeights[layerIndex][neuronIndex][weightIndex]; } } } currentIndex += _config.BatchSize; } while (currentIndex < data.Count);
:
"", ( , ) dE/dz
//last layer for (int j = 0; j < network.Layers[network.Layers.Length - 1].Neurons.Length; j++) { network.Layers[network.Layers.Length - 1].Neurons[j].dEdz = _config.ErrorFunction.CalculatePartialDerivaitveByV2Index(data[inBatchIndex].Output, realOutput, j) * network.Layers[network.Layers.Length - 1].Neurons[j].ActivationFunction. ComputeFirstDerivative(network.Layers[network.Layers.Length - 1].Neurons[j].LastNET); nablaBiases[network.Layers.Length - 1][j] += _config.LearningRate * network.Layers[network.Layers.Length - 1].Neurons[j].dEdz; for (int i = 0; i < network.Layers[network.Layers.Length - 1].Neurons[j].Weights.Length; i++) { nablaWeights[network.Layers.Length - 1][j][i] += _config.LearningRate*(network.Layers[network.Layers.Length - 1].Neurons[j].dEdz* (network.Layers.Length > 1 ? network.Layers[network.Layers.Length - 1 - 1].Neurons[i].LastState : data[inBatchIndex].Input[i]) + _config.RegularizationFactor * network.Layers[network.Layers.Length - 1].Neurons[j].Weights[i] / data.Count); } }
:
"", ( , ) dE/dz, ,
//hidden layers for (int hiddenLayerIndex = network.Layers.Length - 2; hiddenLayerIndex >= 0; hiddenLayerIndex--) { for (int j = 0; j < network.Layers[hiddenLayerIndex].Neurons.Length; j++) { network.Layers[hiddenLayerIndex].Neurons[j].dEdz = 0; for (int k = 0; k < network.Layers[hiddenLayerIndex + 1].Neurons.Length; k++) { network.Layers[hiddenLayerIndex].Neurons[j].dEdz += network.Layers[hiddenLayerIndex + 1].Neurons[k].Weights[j]* network.Layers[hiddenLayerIndex + 1].Neurons[k].dEdz; } network.Layers[hiddenLayerIndex].Neurons[j].dEdz *= network.Layers[hiddenLayerIndex].Neurons[j].ActivationFunction. ComputeFirstDerivative( network.Layers[hiddenLayerIndex].Neurons[j].LastNET ); nablaBiases[hiddenLayerIndex][j] += _config.LearningRate* network.Layers[hiddenLayerIndex].Neurons[j].dEdz; for (int i = 0; i < network.Layers[hiddenLayerIndex].Neurons[j].Weights.Length; i++) { nablaWeights[hiddenLayerIndex][j][i] += _config.LearningRate * ( network.Layers[hiddenLayerIndex].Neurons[j].dEdz * (hiddenLayerIndex > 0 ? network.Layers[hiddenLayerIndex - 1].Neurons[i].LastState : data[inBatchIndex].Input[i]) + _config.RegularizationFactor * network.Layers[hiddenLayerIndex].Neurons[j].Weights[i] / data.Count ); } } }
, ( ), :
//recalculating error on all data //real error currentError = 0; for (int i = 0; i < data.Count; i++) { double[] realOutput = network.ComputeOutput(data[i].Input); currentError += _config.ErrorFunction.Calculate(data[i].Output, realOutput); } currentError *= 1d/data.Count; //regularization term if (Math.Abs(_config.RegularizationFactor - 0d) > Double.Epsilon) { double reg = 0; for (int layerIndex = 0; layerIndex < network.Layers.Length; layerIndex++) { for (int neuronIndex = 0; neuronIndex < network.Layers[layerIndex].Neurons.Length; neuronIndex++) { for (int weightIndex = 0; weightIndex < network.Layers[layerIndex].Neurons[neuronIndex].Weights.Length; weightIndex++) { reg += network.Layers[layerIndex].Neurons[neuronIndex].Weights[weightIndex] * network.Layers[layerIndex].Neurons[neuronIndex].Weights[weightIndex]; } } } currentError += _config.RegularizationFactor * reg / (2 * data.Count); } epochNumber++; Logger.Instance.Log("Eposh #" + epochNumber.ToString() + " finished; current error is " + currentError.ToString() + "; it takes: " + (DateTime.Now - dtStart).Duration().ToString());
, :
} while (epochNumber < _config.MaxEpoches && currentError > _config.MinError && Math.Abs(currentError - lastError) > _config.MinErrorChange);
. , , , . . , , , , , .
:
:
.
, , , , . -)
internal class BackpropagationFCNLearningAlgorithm : ILearningStrategy, public void Train(IMultilayerNeuralNetwork network, IList<DataItem> data).
( ) :
if (_config.BatchSize < 1 || _config.BatchSize > data.Count) { _config.BatchSize = data.Count; } double currentError = Single.MaxValue; double lastError = 0; int epochNumber = 0; Logger.Instance.Log("Start learning...");
, , :
do { //... } while (epochNumber < _config.MaxEpoches && currentError > _config.MinError && Math.Abs(currentError - lastError) > _config.MinErrorChange);
, , . , batch , .
lastError = currentError; DateTime dtStart = DateTime.Now; //preparation for epoche int[] trainingIndices = new int[data.Count]; for (int i = 0; i < data.Count; i++) { trainingIndices[i] = i; } if (_config.BatchSize > 0) { trainingIndices = Shuffle(trainingIndices); }
, , , :
//process data set int currentIndex = 0; do { #region initialize accumulated error for batch, for weights and biases double[][][] nablaWeights = new double[network.Layers.Length][][]; double[][] nablaBiases = new double[network.Layers.Length][]; for (int i = 0; i < network.Layers.Length; i++) { nablaBiases[i] = new double[network.Layers[i].Neurons.Length]; nablaWeights[i] = new double[network.Layers[i].Neurons.Length][]; for (int j = 0; j < network.Layers[i].Neurons.Length; j++) { nablaBiases[i][j] = 0; nablaWeights[i][j] = new double[network.Layers[i].Neurons[j].Weights.Length]; for (int k = 0; k < network.Layers[i].Neurons[j].Weights.Length; k++) { nablaWeights[i][j][k] = 0; } } } #endregion //process one batch for (int inBatchIndex = currentIndex; inBatchIndex < currentIndex + _config.BatchSize && inBatchIndex < data.Count; inBatchIndex++) { //forward pass double[] realOutput = network.ComputeOutput(data[trainingIndices[inBatchIndex]].Input); //backward pass, error propagation //last layer //....................................... //hidden layers //....................................... } //update weights and bias for (int layerIndex = 0; layerIndex < network.Layers.Length; layerIndex++) { for (int neuronIndex = 0; neuronIndex < network.Layers[layerIndex].Neurons.Length; neuronIndex++) { network.Layers[layerIndex].Neurons[neuronIndex].Bias -= nablaBiases[layerIndex][neuronIndex]; for (int weightIndex = 0; weightIndex < network.Layers[layerIndex].Neurons[neuronIndex].Weights.Length; weightIndex++) { network.Layers[layerIndex].Neurons[neuronIndex].Weights[weightIndex] -= nablaWeights[layerIndex][neuronIndex][weightIndex]; } } } currentIndex += _config.BatchSize; } while (currentIndex < data.Count);
:
"", ( , ) dE/dz
//last layer for (int j = 0; j < network.Layers[network.Layers.Length - 1].Neurons.Length; j++) { network.Layers[network.Layers.Length - 1].Neurons[j].dEdz = _config.ErrorFunction.CalculatePartialDerivaitveByV2Index(data[inBatchIndex].Output, realOutput, j) * network.Layers[network.Layers.Length - 1].Neurons[j].ActivationFunction. ComputeFirstDerivative(network.Layers[network.Layers.Length - 1].Neurons[j].LastNET); nablaBiases[network.Layers.Length - 1][j] += _config.LearningRate * network.Layers[network.Layers.Length - 1].Neurons[j].dEdz; for (int i = 0; i < network.Layers[network.Layers.Length - 1].Neurons[j].Weights.Length; i++) { nablaWeights[network.Layers.Length - 1][j][i] += _config.LearningRate*(network.Layers[network.Layers.Length - 1].Neurons[j].dEdz* (network.Layers.Length > 1 ? network.Layers[network.Layers.Length - 1 - 1].Neurons[i].LastState : data[inBatchIndex].Input[i]) + _config.RegularizationFactor * network.Layers[network.Layers.Length - 1].Neurons[j].Weights[i] / data.Count); } }
:
"", ( , ) dE/dz, ,
//hidden layers for (int hiddenLayerIndex = network.Layers.Length - 2; hiddenLayerIndex >= 0; hiddenLayerIndex--) { for (int j = 0; j < network.Layers[hiddenLayerIndex].Neurons.Length; j++) { network.Layers[hiddenLayerIndex].Neurons[j].dEdz = 0; for (int k = 0; k < network.Layers[hiddenLayerIndex + 1].Neurons.Length; k++) { network.Layers[hiddenLayerIndex].Neurons[j].dEdz += network.Layers[hiddenLayerIndex + 1].Neurons[k].Weights[j]* network.Layers[hiddenLayerIndex + 1].Neurons[k].dEdz; } network.Layers[hiddenLayerIndex].Neurons[j].dEdz *= network.Layers[hiddenLayerIndex].Neurons[j].ActivationFunction. ComputeFirstDerivative( network.Layers[hiddenLayerIndex].Neurons[j].LastNET ); nablaBiases[hiddenLayerIndex][j] += _config.LearningRate* network.Layers[hiddenLayerIndex].Neurons[j].dEdz; for (int i = 0; i < network.Layers[hiddenLayerIndex].Neurons[j].Weights.Length; i++) { nablaWeights[hiddenLayerIndex][j][i] += _config.LearningRate * ( network.Layers[hiddenLayerIndex].Neurons[j].dEdz * (hiddenLayerIndex > 0 ? network.Layers[hiddenLayerIndex - 1].Neurons[i].LastState : data[inBatchIndex].Input[i]) + _config.RegularizationFactor * network.Layers[hiddenLayerIndex].Neurons[j].Weights[i] / data.Count ); } } }
, ( ), :
//recalculating error on all data //real error currentError = 0; for (int i = 0; i < data.Count; i++) { double[] realOutput = network.ComputeOutput(data[i].Input); currentError += _config.ErrorFunction.Calculate(data[i].Output, realOutput); } currentError *= 1d/data.Count; //regularization term if (Math.Abs(_config.RegularizationFactor - 0d) > Double.Epsilon) { double reg = 0; for (int layerIndex = 0; layerIndex < network.Layers.Length; layerIndex++) { for (int neuronIndex = 0; neuronIndex < network.Layers[layerIndex].Neurons.Length; neuronIndex++) { for (int weightIndex = 0; weightIndex < network.Layers[layerIndex].Neurons[neuronIndex].Weights.Length; weightIndex++) { reg += network.Layers[layerIndex].Neurons[neuronIndex].Weights[weightIndex] * network.Layers[layerIndex].Neurons[neuronIndex].Weights[weightIndex]; } } } currentError += _config.RegularizationFactor * reg / (2 * data.Count); } epochNumber++; Logger.Instance.Log("Eposh #" + epochNumber.ToString() + " finished; current error is " + currentError.ToString() + "; it takes: " + (DateTime.Now - dtStart).Duration().ToString());
, :
} while (epochNumber < _config.MaxEpoches && currentError > _config.MinError && Math.Abs(currentError - lastError) > _config.MinErrorChange);
. , , , . . , , , , , .
:

:
.
, , , , . -)
internal class BackpropagationFCNLearningAlgorithm : ILearningStrategy, public void Train(IMultilayerNeuralNetwork network, IList<DataItem> data).
( ) :
if (_config.BatchSize < 1 || _config.BatchSize > data.Count) { _config.BatchSize = data.Count; } double currentError = Single.MaxValue; double lastError = 0; int epochNumber = 0; Logger.Instance.Log("Start learning...");
, , :
do { //... } while (epochNumber < _config.MaxEpoches && currentError > _config.MinError && Math.Abs(currentError - lastError) > _config.MinErrorChange);
, , . , batch , .
lastError = currentError; DateTime dtStart = DateTime.Now; //preparation for epoche int[] trainingIndices = new int[data.Count]; for (int i = 0; i < data.Count; i++) { trainingIndices[i] = i; } if (_config.BatchSize > 0) { trainingIndices = Shuffle(trainingIndices); }
, , , :
//process data set int currentIndex = 0; do { #region initialize accumulated error for batch, for weights and biases double[][][] nablaWeights = new double[network.Layers.Length][][]; double[][] nablaBiases = new double[network.Layers.Length][]; for (int i = 0; i < network.Layers.Length; i++) { nablaBiases[i] = new double[network.Layers[i].Neurons.Length]; nablaWeights[i] = new double[network.Layers[i].Neurons.Length][]; for (int j = 0; j < network.Layers[i].Neurons.Length; j++) { nablaBiases[i][j] = 0; nablaWeights[i][j] = new double[network.Layers[i].Neurons[j].Weights.Length]; for (int k = 0; k < network.Layers[i].Neurons[j].Weights.Length; k++) { nablaWeights[i][j][k] = 0; } } } #endregion //process one batch for (int inBatchIndex = currentIndex; inBatchIndex < currentIndex + _config.BatchSize && inBatchIndex < data.Count; inBatchIndex++) { //forward pass double[] realOutput = network.ComputeOutput(data[trainingIndices[inBatchIndex]].Input); //backward pass, error propagation //last layer //....................................... //hidden layers //....................................... } //update weights and bias for (int layerIndex = 0; layerIndex < network.Layers.Length; layerIndex++) { for (int neuronIndex = 0; neuronIndex < network.Layers[layerIndex].Neurons.Length; neuronIndex++) { network.Layers[layerIndex].Neurons[neuronIndex].Bias -= nablaBiases[layerIndex][neuronIndex]; for (int weightIndex = 0; weightIndex < network.Layers[layerIndex].Neurons[neuronIndex].Weights.Length; weightIndex++) { network.Layers[layerIndex].Neurons[neuronIndex].Weights[weightIndex] -= nablaWeights[layerIndex][neuronIndex][weightIndex]; } } } currentIndex += _config.BatchSize; } while (currentIndex < data.Count);
:
"", ( , ) dE/dz
//last layer for (int j = 0; j < network.Layers[network.Layers.Length - 1].Neurons.Length; j++) { network.Layers[network.Layers.Length - 1].Neurons[j].dEdz = _config.ErrorFunction.CalculatePartialDerivaitveByV2Index(data[inBatchIndex].Output, realOutput, j) * network.Layers[network.Layers.Length - 1].Neurons[j].ActivationFunction. ComputeFirstDerivative(network.Layers[network.Layers.Length - 1].Neurons[j].LastNET); nablaBiases[network.Layers.Length - 1][j] += _config.LearningRate * network.Layers[network.Layers.Length - 1].Neurons[j].dEdz; for (int i = 0; i < network.Layers[network.Layers.Length - 1].Neurons[j].Weights.Length; i++) { nablaWeights[network.Layers.Length - 1][j][i] += _config.LearningRate*(network.Layers[network.Layers.Length - 1].Neurons[j].dEdz* (network.Layers.Length > 1 ? network.Layers[network.Layers.Length - 1 - 1].Neurons[i].LastState : data[inBatchIndex].Input[i]) + _config.RegularizationFactor * network.Layers[network.Layers.Length - 1].Neurons[j].Weights[i] / data.Count); } }
:
"", ( , ) dE/dz, ,
//hidden layers for (int hiddenLayerIndex = network.Layers.Length - 2; hiddenLayerIndex >= 0; hiddenLayerIndex--) { for (int j = 0; j < network.Layers[hiddenLayerIndex].Neurons.Length; j++) { network.Layers[hiddenLayerIndex].Neurons[j].dEdz = 0; for (int k = 0; k < network.Layers[hiddenLayerIndex + 1].Neurons.Length; k++) { network.Layers[hiddenLayerIndex].Neurons[j].dEdz += network.Layers[hiddenLayerIndex + 1].Neurons[k].Weights[j]* network.Layers[hiddenLayerIndex + 1].Neurons[k].dEdz; } network.Layers[hiddenLayerIndex].Neurons[j].dEdz *= network.Layers[hiddenLayerIndex].Neurons[j].ActivationFunction. ComputeFirstDerivative( network.Layers[hiddenLayerIndex].Neurons[j].LastNET ); nablaBiases[hiddenLayerIndex][j] += _config.LearningRate* network.Layers[hiddenLayerIndex].Neurons[j].dEdz; for (int i = 0; i < network.Layers[hiddenLayerIndex].Neurons[j].Weights.Length; i++) { nablaWeights[hiddenLayerIndex][j][i] += _config.LearningRate * ( network.Layers[hiddenLayerIndex].Neurons[j].dEdz * (hiddenLayerIndex > 0 ? network.Layers[hiddenLayerIndex - 1].Neurons[i].LastState : data[inBatchIndex].Input[i]) + _config.RegularizationFactor * network.Layers[hiddenLayerIndex].Neurons[j].Weights[i] / data.Count ); } } }
, ( ), :
//recalculating error on all data //real error currentError = 0; for (int i = 0; i < data.Count; i++) { double[] realOutput = network.ComputeOutput(data[i].Input); currentError += _config.ErrorFunction.Calculate(data[i].Output, realOutput); } currentError *= 1d/data.Count; //regularization term if (Math.Abs(_config.RegularizationFactor - 0d) > Double.Epsilon) { double reg = 0; for (int layerIndex = 0; layerIndex < network.Layers.Length; layerIndex++) { for (int neuronIndex = 0; neuronIndex < network.Layers[layerIndex].Neurons.Length; neuronIndex++) { for (int weightIndex = 0; weightIndex < network.Layers[layerIndex].Neurons[neuronIndex].Weights.Length; weightIndex++) { reg += network.Layers[layerIndex].Neurons[neuronIndex].Weights[weightIndex] * network.Layers[layerIndex].Neurons[neuronIndex].Weights[weightIndex]; } } } currentError += _config.RegularizationFactor * reg / (2 * data.Count); } epochNumber++; Logger.Instance.Log("Eposh #" + epochNumber.ToString() + " finished; current error is " + currentError.ToString() + "; it takes: " + (DateTime.Now - dtStart).Duration().ToString());
, :
} while (epochNumber < _config.MaxEpoches && currentError > _config.MinError && Math.Abs(currentError - lastError) > _config.MinErrorChange);
. , , , . . , , , , , .
:
:
.
, , , , . -)
internal class BackpropagationFCNLearningAlgorithm : ILearningStrategy, public void Train(IMultilayerNeuralNetwork network, IList<DataItem> data).
( ) :
if (_config.BatchSize < 1 || _config.BatchSize > data.Count) { _config.BatchSize = data.Count; } double currentError = Single.MaxValue; double lastError = 0; int epochNumber = 0; Logger.Instance.Log("Start learning...");
, , :
do { //... } while (epochNumber < _config.MaxEpoches && currentError > _config.MinError && Math.Abs(currentError - lastError) > _config.MinErrorChange);
, , . , batch , .
lastError = currentError; DateTime dtStart = DateTime.Now; //preparation for epoche int[] trainingIndices = new int[data.Count]; for (int i = 0; i < data.Count; i++) { trainingIndices[i] = i; } if (_config.BatchSize > 0) { trainingIndices = Shuffle(trainingIndices); }
, , , :
//process data set int currentIndex = 0; do { #region initialize accumulated error for batch, for weights and biases double[][][] nablaWeights = new double[network.Layers.Length][][]; double[][] nablaBiases = new double[network.Layers.Length][]; for (int i = 0; i < network.Layers.Length; i++) { nablaBiases[i] = new double[network.Layers[i].Neurons.Length]; nablaWeights[i] = new double[network.Layers[i].Neurons.Length][]; for (int j = 0; j < network.Layers[i].Neurons.Length; j++) { nablaBiases[i][j] = 0; nablaWeights[i][j] = new double[network.Layers[i].Neurons[j].Weights.Length]; for (int k = 0; k < network.Layers[i].Neurons[j].Weights.Length; k++) { nablaWeights[i][j][k] = 0; } } } #endregion //process one batch for (int inBatchIndex = currentIndex; inBatchIndex < currentIndex + _config.BatchSize && inBatchIndex < data.Count; inBatchIndex++) { //forward pass double[] realOutput = network.ComputeOutput(data[trainingIndices[inBatchIndex]].Input); //backward pass, error propagation //last layer //....................................... //hidden layers //....................................... } //update weights and bias for (int layerIndex = 0; layerIndex < network.Layers.Length; layerIndex++) { for (int neuronIndex = 0; neuronIndex < network.Layers[layerIndex].Neurons.Length; neuronIndex++) { network.Layers[layerIndex].Neurons[neuronIndex].Bias -= nablaBiases[layerIndex][neuronIndex]; for (int weightIndex = 0; weightIndex < network.Layers[layerIndex].Neurons[neuronIndex].Weights.Length; weightIndex++) { network.Layers[layerIndex].Neurons[neuronIndex].Weights[weightIndex] -= nablaWeights[layerIndex][neuronIndex][weightIndex]; } } } currentIndex += _config.BatchSize; } while (currentIndex < data.Count);
:
"", ( , ) dE/dz
//last layer for (int j = 0; j < network.Layers[network.Layers.Length - 1].Neurons.Length; j++) { network.Layers[network.Layers.Length - 1].Neurons[j].dEdz = _config.ErrorFunction.CalculatePartialDerivaitveByV2Index(data[inBatchIndex].Output, realOutput, j) * network.Layers[network.Layers.Length - 1].Neurons[j].ActivationFunction. ComputeFirstDerivative(network.Layers[network.Layers.Length - 1].Neurons[j].LastNET); nablaBiases[network.Layers.Length - 1][j] += _config.LearningRate * network.Layers[network.Layers.Length - 1].Neurons[j].dEdz; for (int i = 0; i < network.Layers[network.Layers.Length - 1].Neurons[j].Weights.Length; i++) { nablaWeights[network.Layers.Length - 1][j][i] += _config.LearningRate*(network.Layers[network.Layers.Length - 1].Neurons[j].dEdz* (network.Layers.Length > 1 ? network.Layers[network.Layers.Length - 1 - 1].Neurons[i].LastState : data[inBatchIndex].Input[i]) + _config.RegularizationFactor * network.Layers[network.Layers.Length - 1].Neurons[j].Weights[i] / data.Count); } }
:
"", ( , ) dE/dz, ,
//hidden layers for (int hiddenLayerIndex = network.Layers.Length - 2; hiddenLayerIndex >= 0; hiddenLayerIndex--) { for (int j = 0; j < network.Layers[hiddenLayerIndex].Neurons.Length; j++) { network.Layers[hiddenLayerIndex].Neurons[j].dEdz = 0; for (int k = 0; k < network.Layers[hiddenLayerIndex + 1].Neurons.Length; k++) { network.Layers[hiddenLayerIndex].Neurons[j].dEdz += network.Layers[hiddenLayerIndex + 1].Neurons[k].Weights[j]* network.Layers[hiddenLayerIndex + 1].Neurons[k].dEdz; } network.Layers[hiddenLayerIndex].Neurons[j].dEdz *= network.Layers[hiddenLayerIndex].Neurons[j].ActivationFunction. ComputeFirstDerivative( network.Layers[hiddenLayerIndex].Neurons[j].LastNET ); nablaBiases[hiddenLayerIndex][j] += _config.LearningRate* network.Layers[hiddenLayerIndex].Neurons[j].dEdz; for (int i = 0; i < network.Layers[hiddenLayerIndex].Neurons[j].Weights.Length; i++) { nablaWeights[hiddenLayerIndex][j][i] += _config.LearningRate * ( network.Layers[hiddenLayerIndex].Neurons[j].dEdz * (hiddenLayerIndex > 0 ? network.Layers[hiddenLayerIndex - 1].Neurons[i].LastState : data[inBatchIndex].Input[i]) + _config.RegularizationFactor * network.Layers[hiddenLayerIndex].Neurons[j].Weights[i] / data.Count ); } } }
, ( ), :
//recalculating error on all data //real error currentError = 0; for (int i = 0; i < data.Count; i++) { double[] realOutput = network.ComputeOutput(data[i].Input); currentError += _config.ErrorFunction.Calculate(data[i].Output, realOutput); } currentError *= 1d/data.Count; //regularization term if (Math.Abs(_config.RegularizationFactor - 0d) > Double.Epsilon) { double reg = 0; for (int layerIndex = 0; layerIndex < network.Layers.Length; layerIndex++) { for (int neuronIndex = 0; neuronIndex < network.Layers[layerIndex].Neurons.Length; neuronIndex++) { for (int weightIndex = 0; weightIndex < network.Layers[layerIndex].Neurons[neuronIndex].Weights.Length; weightIndex++) { reg += network.Layers[layerIndex].Neurons[neuronIndex].Weights[weightIndex] * network.Layers[layerIndex].Neurons[neuronIndex].Weights[weightIndex]; } } } currentError += _config.RegularizationFactor * reg / (2 * data.Count); } epochNumber++; Logger.Instance.Log("Eposh #" + epochNumber.ToString() + " finished; current error is " + currentError.ToString() + "; it takes: " + (DateTime.Now - dtStart).Duration().ToString());
, :
} while (epochNumber < _config.MaxEpoches && currentError > _config.MinError && Math.Abs(currentError - lastError) > _config.MinErrorChange);
. , , , . . , , , , , .
:

:
.
, , , , . -)
internal class BackpropagationFCNLearningAlgorithm : ILearningStrategy, public void Train(IMultilayerNeuralNetwork network, IList<DataItem> data).
( ) :
if (_config.BatchSize < 1 || _config.BatchSize > data.Count) { _config.BatchSize = data.Count; } double currentError = Single.MaxValue; double lastError = 0; int epochNumber = 0; Logger.Instance.Log("Start learning...");
, , :
do { //... } while (epochNumber < _config.MaxEpoches && currentError > _config.MinError && Math.Abs(currentError - lastError) > _config.MinErrorChange);
, , . , batch , .
lastError = currentError; DateTime dtStart = DateTime.Now; //preparation for epoche int[] trainingIndices = new int[data.Count]; for (int i = 0; i < data.Count; i++) { trainingIndices[i] = i; } if (_config.BatchSize > 0) { trainingIndices = Shuffle(trainingIndices); }
, , , :
//process data set int currentIndex = 0; do { #region initialize accumulated error for batch, for weights and biases double[][][] nablaWeights = new double[network.Layers.Length][][]; double[][] nablaBiases = new double[network.Layers.Length][]; for (int i = 0; i < network.Layers.Length; i++) { nablaBiases[i] = new double[network.Layers[i].Neurons.Length]; nablaWeights[i] = new double[network.Layers[i].Neurons.Length][]; for (int j = 0; j < network.Layers[i].Neurons.Length; j++) { nablaBiases[i][j] = 0; nablaWeights[i][j] = new double[network.Layers[i].Neurons[j].Weights.Length]; for (int k = 0; k < network.Layers[i].Neurons[j].Weights.Length; k++) { nablaWeights[i][j][k] = 0; } } } #endregion //process one batch for (int inBatchIndex = currentIndex; inBatchIndex < currentIndex + _config.BatchSize && inBatchIndex < data.Count; inBatchIndex++) { //forward pass double[] realOutput = network.ComputeOutput(data[trainingIndices[inBatchIndex]].Input); //backward pass, error propagation //last layer //....................................... //hidden layers //....................................... } //update weights and bias for (int layerIndex = 0; layerIndex < network.Layers.Length; layerIndex++) { for (int neuronIndex = 0; neuronIndex < network.Layers[layerIndex].Neurons.Length; neuronIndex++) { network.Layers[layerIndex].Neurons[neuronIndex].Bias -= nablaBiases[layerIndex][neuronIndex]; for (int weightIndex = 0; weightIndex < network.Layers[layerIndex].Neurons[neuronIndex].Weights.Length; weightIndex++) { network.Layers[layerIndex].Neurons[neuronIndex].Weights[weightIndex] -= nablaWeights[layerIndex][neuronIndex][weightIndex]; } } } currentIndex += _config.BatchSize; } while (currentIndex < data.Count);
:
"", ( , ) dE/dz
//last layer for (int j = 0; j < network.Layers[network.Layers.Length - 1].Neurons.Length; j++) { network.Layers[network.Layers.Length - 1].Neurons[j].dEdz = _config.ErrorFunction.CalculatePartialDerivaitveByV2Index(data[inBatchIndex].Output, realOutput, j) * network.Layers[network.Layers.Length - 1].Neurons[j].ActivationFunction. ComputeFirstDerivative(network.Layers[network.Layers.Length - 1].Neurons[j].LastNET); nablaBiases[network.Layers.Length - 1][j] += _config.LearningRate * network.Layers[network.Layers.Length - 1].Neurons[j].dEdz; for (int i = 0; i < network.Layers[network.Layers.Length - 1].Neurons[j].Weights.Length; i++) { nablaWeights[network.Layers.Length - 1][j][i] += _config.LearningRate*(network.Layers[network.Layers.Length - 1].Neurons[j].dEdz* (network.Layers.Length > 1 ? network.Layers[network.Layers.Length - 1 - 1].Neurons[i].LastState : data[inBatchIndex].Input[i]) + _config.RegularizationFactor * network.Layers[network.Layers.Length - 1].Neurons[j].Weights[i] / data.Count); } }
:
"", ( , ) dE/dz, ,
//hidden layers for (int hiddenLayerIndex = network.Layers.Length - 2; hiddenLayerIndex >= 0; hiddenLayerIndex--) { for (int j = 0; j < network.Layers[hiddenLayerIndex].Neurons.Length; j++) { network.Layers[hiddenLayerIndex].Neurons[j].dEdz = 0; for (int k = 0; k < network.Layers[hiddenLayerIndex + 1].Neurons.Length; k++) { network.Layers[hiddenLayerIndex].Neurons[j].dEdz += network.Layers[hiddenLayerIndex + 1].Neurons[k].Weights[j]* network.Layers[hiddenLayerIndex + 1].Neurons[k].dEdz; } network.Layers[hiddenLayerIndex].Neurons[j].dEdz *= network.Layers[hiddenLayerIndex].Neurons[j].ActivationFunction. ComputeFirstDerivative( network.Layers[hiddenLayerIndex].Neurons[j].LastNET ); nablaBiases[hiddenLayerIndex][j] += _config.LearningRate* network.Layers[hiddenLayerIndex].Neurons[j].dEdz; for (int i = 0; i < network.Layers[hiddenLayerIndex].Neurons[j].Weights.Length; i++) { nablaWeights[hiddenLayerIndex][j][i] += _config.LearningRate * ( network.Layers[hiddenLayerIndex].Neurons[j].dEdz * (hiddenLayerIndex > 0 ? network.Layers[hiddenLayerIndex - 1].Neurons[i].LastState : data[inBatchIndex].Input[i]) + _config.RegularizationFactor * network.Layers[hiddenLayerIndex].Neurons[j].Weights[i] / data.Count ); } } }
, ( ), :
//recalculating error on all data //real error currentError = 0; for (int i = 0; i < data.Count; i++) { double[] realOutput = network.ComputeOutput(data[i].Input); currentError += _config.ErrorFunction.Calculate(data[i].Output, realOutput); } currentError *= 1d/data.Count; //regularization term if (Math.Abs(_config.RegularizationFactor - 0d) > Double.Epsilon) { double reg = 0; for (int layerIndex = 0; layerIndex < network.Layers.Length; layerIndex++) { for (int neuronIndex = 0; neuronIndex < network.Layers[layerIndex].Neurons.Length; neuronIndex++) { for (int weightIndex = 0; weightIndex < network.Layers[layerIndex].Neurons[neuronIndex].Weights.Length; weightIndex++) { reg += network.Layers[layerIndex].Neurons[neuronIndex].Weights[weightIndex] * network.Layers[layerIndex].Neurons[neuronIndex].Weights[weightIndex]; } } } currentError += _config.RegularizationFactor * reg / (2 * data.Count); } epochNumber++; Logger.Instance.Log("Eposh #" + epochNumber.ToString() + " finished; current error is " + currentError.ToString() + "; it takes: " + (DateTime.Now - dtStart).Duration().ToString());
, :
} while (epochNumber < _config.MaxEpoches && currentError > _config.MinError && Math.Abs(currentError - lastError) > _config.MinErrorChange);
. , , , . . , , , , , .
:

:
.
, , , , . -)
internal class BackpropagationFCNLearningAlgorithm : ILearningStrategy, public void Train(IMultilayerNeuralNetwork network, IList<DataItem> data).
( ) :
if (_config.BatchSize < 1 || _config.BatchSize > data.Count) { _config.BatchSize = data.Count; } double currentError = Single.MaxValue; double lastError = 0; int epochNumber = 0; Logger.Instance.Log("Start learning...");
, , :
do { //... } while (epochNumber < _config.MaxEpoches && currentError > _config.MinError && Math.Abs(currentError - lastError) > _config.MinErrorChange);
, , . , batch , .
lastError = currentError; DateTime dtStart = DateTime.Now; //preparation for epoche int[] trainingIndices = new int[data.Count]; for (int i = 0; i < data.Count; i++) { trainingIndices[i] = i; } if (_config.BatchSize > 0) { trainingIndices = Shuffle(trainingIndices); }
, , , :
//process data set int currentIndex = 0; do { #region initialize accumulated error for batch, for weights and biases double[][][] nablaWeights = new double[network.Layers.Length][][]; double[][] nablaBiases = new double[network.Layers.Length][]; for (int i = 0; i < network.Layers.Length; i++) { nablaBiases[i] = new double[network.Layers[i].Neurons.Length]; nablaWeights[i] = new double[network.Layers[i].Neurons.Length][]; for (int j = 0; j < network.Layers[i].Neurons.Length; j++) { nablaBiases[i][j] = 0; nablaWeights[i][j] = new double[network.Layers[i].Neurons[j].Weights.Length]; for (int k = 0; k < network.Layers[i].Neurons[j].Weights.Length; k++) { nablaWeights[i][j][k] = 0; } } } #endregion //process one batch for (int inBatchIndex = currentIndex; inBatchIndex < currentIndex + _config.BatchSize && inBatchIndex < data.Count; inBatchIndex++) { //forward pass double[] realOutput = network.ComputeOutput(data[trainingIndices[inBatchIndex]].Input); //backward pass, error propagation //last layer //....................................... //hidden layers //....................................... } //update weights and bias for (int layerIndex = 0; layerIndex < network.Layers.Length; layerIndex++) { for (int neuronIndex = 0; neuronIndex < network.Layers[layerIndex].Neurons.Length; neuronIndex++) { network.Layers[layerIndex].Neurons[neuronIndex].Bias -= nablaBiases[layerIndex][neuronIndex]; for (int weightIndex = 0; weightIndex < network.Layers[layerIndex].Neurons[neuronIndex].Weights.Length; weightIndex++) { network.Layers[layerIndex].Neurons[neuronIndex].Weights[weightIndex] -= nablaWeights[layerIndex][neuronIndex][weightIndex]; } } } currentIndex += _config.BatchSize; } while (currentIndex < data.Count);
:
"", ( , ) dE/dz
//last layer for (int j = 0; j < network.Layers[network.Layers.Length - 1].Neurons.Length; j++) { network.Layers[network.Layers.Length - 1].Neurons[j].dEdz = _config.ErrorFunction.CalculatePartialDerivaitveByV2Index(data[inBatchIndex].Output, realOutput, j) * network.Layers[network.Layers.Length - 1].Neurons[j].ActivationFunction. ComputeFirstDerivative(network.Layers[network.Layers.Length - 1].Neurons[j].LastNET); nablaBiases[network.Layers.Length - 1][j] += _config.LearningRate * network.Layers[network.Layers.Length - 1].Neurons[j].dEdz; for (int i = 0; i < network.Layers[network.Layers.Length - 1].Neurons[j].Weights.Length; i++) { nablaWeights[network.Layers.Length - 1][j][i] += _config.LearningRate*(network.Layers[network.Layers.Length - 1].Neurons[j].dEdz* (network.Layers.Length > 1 ? network.Layers[network.Layers.Length - 1 - 1].Neurons[i].LastState : data[inBatchIndex].Input[i]) + _config.RegularizationFactor * network.Layers[network.Layers.Length - 1].Neurons[j].Weights[i] / data.Count); } }
:
"", ( , ) dE/dz, ,
//hidden layers for (int hiddenLayerIndex = network.Layers.Length - 2; hiddenLayerIndex >= 0; hiddenLayerIndex--) { for (int j = 0; j < network.Layers[hiddenLayerIndex].Neurons.Length; j++) { network.Layers[hiddenLayerIndex].Neurons[j].dEdz = 0; for (int k = 0; k < network.Layers[hiddenLayerIndex + 1].Neurons.Length; k++) { network.Layers[hiddenLayerIndex].Neurons[j].dEdz += network.Layers[hiddenLayerIndex + 1].Neurons[k].Weights[j]* network.Layers[hiddenLayerIndex + 1].Neurons[k].dEdz; } network.Layers[hiddenLayerIndex].Neurons[j].dEdz *= network.Layers[hiddenLayerIndex].Neurons[j].ActivationFunction. ComputeFirstDerivative( network.Layers[hiddenLayerIndex].Neurons[j].LastNET ); nablaBiases[hiddenLayerIndex][j] += _config.LearningRate* network.Layers[hiddenLayerIndex].Neurons[j].dEdz; for (int i = 0; i < network.Layers[hiddenLayerIndex].Neurons[j].Weights.Length; i++) { nablaWeights[hiddenLayerIndex][j][i] += _config.LearningRate * ( network.Layers[hiddenLayerIndex].Neurons[j].dEdz * (hiddenLayerIndex > 0 ? network.Layers[hiddenLayerIndex - 1].Neurons[i].LastState : data[inBatchIndex].Input[i]) + _config.RegularizationFactor * network.Layers[hiddenLayerIndex].Neurons[j].Weights[i] / data.Count ); } } }
, ( ), :
//recalculating error on all data //real error currentError = 0; for (int i = 0; i < data.Count; i++) { double[] realOutput = network.ComputeOutput(data[i].Input); currentError += _config.ErrorFunction.Calculate(data[i].Output, realOutput); } currentError *= 1d/data.Count; //regularization term if (Math.Abs(_config.RegularizationFactor - 0d) > Double.Epsilon) { double reg = 0; for (int layerIndex = 0; layerIndex < network.Layers.Length; layerIndex++) { for (int neuronIndex = 0; neuronIndex < network.Layers[layerIndex].Neurons.Length; neuronIndex++) { for (int weightIndex = 0; weightIndex < network.Layers[layerIndex].Neurons[neuronIndex].Weights.Length; weightIndex++) { reg += network.Layers[layerIndex].Neurons[neuronIndex].Weights[weightIndex] * network.Layers[layerIndex].Neurons[neuronIndex].Weights[weightIndex]; } } } currentError += _config.RegularizationFactor * reg / (2 * data.Count); } epochNumber++; Logger.Instance.Log("Eposh #" + epochNumber.ToString() + " finished; current error is " + currentError.ToString() + "; it takes: " + (DateTime.Now - dtStart).Duration().ToString());
, :
} while (epochNumber < _config.MaxEpoches && currentError > _config.MinError && Math.Abs(currentError - lastError) > _config.MinErrorChange);
. , , , . . , , , , , .
:

:
.
, , , , . -)