ããã«ã¡ã¯ãHabrïŒ æè¿ãæ©æ¢°åŠç¿ãšããŒã¿ãµã€ãšã³ã¹å
šäœããŸããŸãäžè¬çã«ãªã£ãŠããŸãã æ°ããã©ã€ãã©ãªã¯åžžã«ç»å ŽããŠãããæ©æ¢°åŠç¿ã¢ãã«ã®ãã¬ãŒãã³ã°ã«å¿
èŠãªã³ãŒãã¯ãããããã§ãã ãã®ãããªç¶æ³ã§ã¯ãæ©æ¢°åŠç¿ã¯ããèªäœãç®çã§ã¯ãªããåé¡ã解決ããããã®ããŒã«ã§ããããšãå¿ããããšãã§ããŸãã äœæ¥ã¢ãã«ãäœæããã ãã§ã¯äžååã§ããåæã®çµæã宿§çã«æç€ºããããäœæ¥è£œåãäœæããããšãåæ§ã«éèŠã§ãã

ãŠãŒã¶ãŒãæããæ°åã§åèšç·Žãããã¢ãã«ã䜿çšããŠãæ°åã®ææžãèªèã®ããã®
ãããžã§ã¯ããäœæããæ¹æ³ã«ã€ããŠã話ããããšæããŸãã 2ã€ã®ã¢ãã«ã䜿çšãããŸããçŽç²ãªnumpyã®åçŽãªãã¥ãŒã©ã«ãããã¯ãŒã¯ïŒFNNïŒãšTensorflowã®ç³ã¿èŸŒã¿ãããã¯ãŒã¯ïŒCNNïŒã§ãã æ¬¡ã®ããšãæåããè¡ãæ¹æ³ãåŠç¿ã§ããŸãã
- FlaskãšBootstrapã䜿çšããŠç°¡åãªãµã€ããäœæããŸãã
- Herokuãã©ãããã©ãŒã ã«é
眮ããŸãã
- Amazon s3ã¯ã©ãŠãã䜿çšããŠããŒã¿ã®ä¿åãšèªã¿èŸŒã¿ãå®è£
ãã
- ç¬èªã®ããŒã¿ã»ãããæ§ç¯ããŸãã
- æ©æ¢°åŠç¿ã¢ãã«ïŒFNNããã³CNNïŒããã¬ãŒãã³ã°ããŸãã
- ãããã®ã¢ãã«ãåãã¬ãŒãã³ã°ã§ããããã«ããŸãã
- æãããç»åãèªèã§ãããµã€ããäœããŸãã
ãããžã§ã¯ããå®å
šã«çè§£ããã«ã¯ãç»åèªèã§ãã£ãŒãã©ãŒãã³ã°ãã©ã®ããã«æ©èœããããçè§£ããFlaskã®åºæ¬çãªç¥èãšãHTMLãJSãCSSã®å°ãã®ç¥èãå¿
èŠã§ãã
ç§ã«ã€ããŠå°ã
ã¢ã¹ã¯ã¯å·ç«å€§åŠçµæžåŠéšã忥åŸãERPã·ã¹ãã ã®å®è£
ãšéçºã®åéã§ITã³ã³ãµã«ãã£ã³ã°ã«4幎éåŸäºããŸããã åãããšã¯éåžžã«é¢çœãã£ãã§ããé·å¹Žã«ããã£ãŠå€ãã®æçšãªããšãåŠã³ãŸãããããããŠãããç§ã®ãã®ã§ã¯ãªãããšãçè§£ããããã«ãªããŸããã ããèããŠãããæŽ»ååéãå€ããŠæ©æ¢°åŠç¿ã®æ¹åã«åãæ¿ããããšã«ããŸããïŒèªå€§åºåã§ã¯ãªããæ¬åœã«èå³ãæã£ãããã§ãïŒã ãããè¡ãã«ã¯ãçµäºããŠçŽ6ãæéåããŠãããã°ã©ãã³ã°ãæ©æ¢°åŠç¿ãªã©ã®å¿
èŠãªããšãåå¥ã«å匷ããŸããã ãã®åŸãå°é£ã䌎ããŸãããããã§ããã®æ¹åã§äœæ¥ãèŠã€ãããŸããã æãªãšãã«ã¹ãã«ãéçºããæŽ»æ°ã¥ããããšããŠããŸãã
ã¢ã€ãã¢ã®èµ·æº
æ°ãæåãç§ã¯Courseraã§YandexãšMIPTã®å°éåãå®äºããŸããã 圌女ã¯Slackã«èªåã®ããŒã ãæã¡ã4æã«ããã§ã¹ã¿ã³ãã©ãŒãã®cs231nã³ãŒã¹ã®ããã«ã°ã«ãŒããç·šæããŸããã ãããã©ã®ããã«èµ·ãã£ããã¯å¥ã®è©±ã§ããããã€ã³ãã«è¿ãã§ãã ã³ãŒã¹ã®éèŠãªéšåã¯ç¬ç«ãããããžã§ã¯ãïŒåŠçã®æçžŸã®40ïŒ
ïŒã§ãããããã«ããè¡åã®å®å
šãªèªç±ãæäŸãããŸãã çå£ã«äœããããŠãããã«ã€ããŠèšäºãæžãããšã®æå³ãããããŸããã§ããããããã§ãç§ã®éã¯ç§ã«å°å³ããã£ãŠã³ãŒã¹ãçµããããã«é Œã¿ãŸããã ãã®é ãTensorflowã§æ°åãš2ã€ã®ã°ãªãããæç»ã§ãã
Webãµã€ãã«åºäŒããå³åº§ã«ãããèªèããŠçµæã衚瀺ããŸããã åæ§ã®ããšãè¡ãããšã«ããŸããããããŒã¿ãšã¢ãã«ã䜿çšããŸããã
æ¬äœ
ããã§ã¯ããããžã§ã¯ããå®è£
ããããã«äœãã©ã®ããã«è¡ã£ããã«ã€ããŠèª¬æããŸãã 説æã¯ãè¡ãããããšãç¹°ãè¿ãããšãã§ããã»ã©ååã«è©³çްã«ãªããŸãããåºæ¬çãªããšãç°¡åã«èª¬æãããã¹ãããããŸãã
ãããžã§ã¯ãèšç»
倧ããªäœãã«çæããåã«ãäœããèšç»ãã䟡å€ããããŸãã ãã®éçšã§ãæ°ãã詳现ãæããã«ãªããèšç»ãä¿®æ£ããå¿
èŠããããŸãããããã€ãã®æåã®ããžã§ã³ãä¿®æ£ããå¿
èŠããããŸãã
- æ©æ¢°åŠç¿ãããžã§ã¯ãã®åºæ¬çãªåé¡ã®1ã€ã¯ãã©ã®ããŒã¿ã䜿çšããã©ãã§ååŸããããšããåé¡ã§ãã MNISTããŒã¿ã»ããã¯ãæ°åèªèã®åé¡ã§ç©æ¥µçã«äœ¿çšãããŠããããã䜿çšããããããŸããã§ããã ã€ã³ã¿ãŒãããã§ã¯ãMNISTã§ã¢ãã«ããã¬ãŒãã³ã°ãããåæ§ã®ãããžã§ã¯ãã®äŸããããŸãïŒããšãã°ã åè¿°ã® ïŒããäœãæ°ããããšããããã£ãã®ã§ãã ãããŠæåŸã«ãMNISTã®æ°åã®å€ãã¯çŸå®ããããé¢ããŠããããã«æããŸãã-ããŒã¿ã»ãããèŠããšãçŸå®ã«ã¯æ³åãã«ããå€ãã®ãªãã·ã§ã³ã«åºããããŸããïŒãã®äººãæ¬åœã«ã²ã©ãææžãæåãæã£ãŠããå Žåãé€ãïŒã ããã«ããã©ãŠã¶ã§ããŠã¹ã䜿çšããŠæ°åãæãããšã¯ããã³ã§æ°åãæžãããšãšã¯å€å°ç°ãªããŸãã ãã®çµæãç§ã¯èªåã®ããŒã¿ã»ããããŸãšããããšã«ããŸããã
- 次ã®ïŒãŸãã¯ãåæã«ïŒã¹ãããã¯ãããŒã¿ãåéããããã®Webãµã€ããäœæããããšã§ãã åœæãç§ã¯Flaskã®åºæ¬çãªç¥èã ãã§ãªããHTMLãJSãCSSã«ã€ããŠãç¥ã£ãŠããŸããã ãããã£ãŠãFlaskã«ãµã€ããäœæããããšã«ããŸãããHerokuããã¹ãã£ã³ã°ãšããŠéžæãããŸãããå°ããªãµã€ãããã°ããç°¡åã«ãã¹ãã§ããããã§ãã
- 次ã«ãã¢ãã«èªäœãäœæããå¿
èŠããããŸãããããã«ãããäž»ãªäœæ¥ãè¡ãããŸãã cs231nã®åŸãç»åèªèçšã®ãã¥ãŒã©ã«ãããã¯ãŒã¯ã¢ãŒããã¯ãã£ãäœæããã®ã«ååãªçµéšããã£ãããããã®æé ã¯æãç°¡åã«æããŸããã 以åã¯ãããã€ãã®ã¢ãã«ãäœæãããã£ãã®ã§ãããåŸã«FNNãšCNNã®2ã€ã«çŠç¹ãåãããããšã«ããŸããã ããã«ããããã®ã¢ãã«ããã¬ãŒãã³ã°ã§ããããã«ããå¿
èŠããããŸããããã®ããŒãã«ã€ããŠã¯ãã§ã«ããã€ãã®ã¢ã€ãã¢ããããŸããã
- ã¢ãã«ãæºåããåŸããµã€ããé©åãªå€èгã«ããäœããã®åœ¢ã§äºæž¬ã衚瀺ããçãã®æ£ãããè©äŸ¡ããæ©èœãå°ã説æãããã®ä»å€ãã®å°ãããŠããŸããªãããšãè¡ãæ©äŒãäžããŸãã èšç»ã®æ®µéã§ãç§ã¯ããã«ã€ããŠèããããšã«å€ãã®æéãè²»ããããåã«ãªã¹ããäœæããŸããã
ããŒã¿åé
ãããžã§ã¯ãã«è²»ãããæéã®ã»ãŒååãããŒã¿åéã«è²»ããããŸããã äºå®ãç§ã¯äœãããªããã°ãªããªããã«ã€ããŠã»ãšãã©ç¥ããªãã£ãã®ã§ã詊è¡é¯èª€ãç¹°ãè¿ãå¿
èŠããããŸããã åœç¶ãè€éãªã®ã¯å³é¢èªäœã§ã¯ãªããæ°åãæç»ããŠã©ããã«ä¿åãããµã€ãã®äœæã§ãã ãããè¡ãã«ã¯ãFlaskãããããçè§£ããJavascriptãæãäžããAmazon S3ã¯ã©ãŠããçè§£ããHerokuã«ã€ããŠåŠã¶å¿
èŠããããŸããã ç§ã¯ãããžã§ã¯ãã®æåã«æã£ãŠããã®ãšåãã¬ãã«ã®ç¥èãæã£ãŠããããç¹°ãè¿ãããšãã§ããããã«ãããããã¹ãŠãåå詳现ã«èª¬æããŸãã
以åãç§ã¯ãã®å³ãæããŸããïŒ
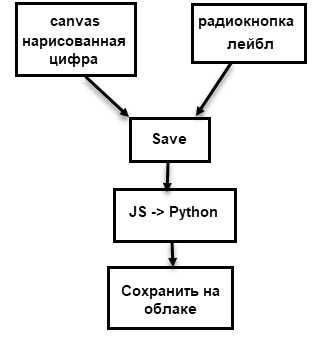
ããŒã¿åéèªäœã¯æ°æ¥ããŸãããŸãã¯æ°æéã®çŽç²ãªæéãèŠããŸããã ç§ã¯1000æ¡ãããããçŽ100æ¡ïŒæ£ç¢ºã§ã¯ãªãïŒãæç»ããç°ãªãã¹ã¿ã€ã«ã§æç»ããããšããŸãããããã¡ãããå¯èœãªãã¹ãŠã®ææžããªãã·ã§ã³ãã«ããŒããããšã¯ã§ããŸããã§ããããããã¯ç®æšã§ã¯ãããŸããã§ããã
ãµã€ãã®æåã®ããŒãžã§ã³ã®äœæïŒããŒã¿åéçšïŒ
ãµã€ãã®æåã®ããŒãžã§ã³ã¯æ¬¡ã®ããã«ãªããŸããã

æãåºæ¬çãªæ©èœãããããŸããã§ããã
- æç»çšã®ãã£ã³ãã¹ã
- ã©ãã«ãéžæããããã®ã©ãžãªãã¿ã³ã
- ç»åãä¿åãããã£ã³ãã¹ãã¯ãªãŒãã³ã°ããããã®ãã¿ã³ã
- ä¿åãæ£åžžã«æžã蟌ãŸãããã£ãŒã«ãã
- Amazonã¯ã©ãŠãã«åçãä¿åããŸãã
ããã§ãããã«ã€ããŠãã£ãšè©³ãã説æããŸãããã ç¹ã«ãã®èšäºã§ã¯ãæå°éã®æ©èœãåããããŒãžã§ã³ã®ãµã€ããäœæããŸããããã®äŸã§ã¯ãäžèšã®æ¹æ³ã説æããŸãã
泚ïŒãµã€ããé·ãé誰ãã«ãã£ãŠéãããŠããªãå Žåããµã€ãã®èµ·åã«ã¯æå€§20ã30ç§ããããŸããããã¯ãã¹ãã£ã³ã°ã®ç¡æããŒãžã§ã³ã®ã³ã¹ãã§ãã ãã®ç¶æ³ã¯ããµã€ãã®ãã«ããŒãžã§ã³ã«é¢é£ããŠããŸãã
ãã©ã¹ã³ã«ã€ããŠç°¡åã«
Flaskã¯ãWebãµã€ããäœæããããã®Pythonãã¬ãŒã ã¯ãŒã¯ã§ãã å
¬åŒãŠã§ããµã€ãã«ã¯çŽ æŽããã
玹ä»ããããŸãã Flaskã䜿çšããŠæ
å ±ãéåä¿¡ããããŸããŸãªæ¹æ³ãããããããã®ãããžã§ã¯ãã§ã¯AJAXã䜿çšããŸããã AJAXã¯ããã©ãŠã¶ãšWebãµãŒããŒéã§ããŒã¿ããããã¯ã°ã©ãŠã³ããã§äº€æããæ©èœãæäŸããŸããããã«ãããããŒã¿ã転éãããã³ã«ããŒãžããªããŒãããå¿
èŠããªããªããŸãã
ãããžã§ã¯ãæ§é

ãããžã§ã¯ãã§äœ¿çšããããã¹ãŠã®ãã¡ã€ã«ã¯ã2ã€ã®çãããªãã°ã«ãŒãã«åããããšãã§ããŸããã¢ããªã±ãŒã·ã§ã³ãHerokuã§åäœããããã«å¿
èŠãªéšåã¯å°ãããæ®ãã¯ãã¹ãŠãµã€ãã®äœæ¥ã«çŽæ¥é¢äžããŸãã
HTMLãšJS
HTMLãã¡ã€ã«ã¯ãã³ãã¬ãŒããã©ã«ããŒã«ä¿åããå¿
èŠããããŸãããã®æ®µéã§ã¯ããã³ãã¬ãŒããã©ã«ããŒãããã°ååã§ãã ããã¥ã¡ã³ãããããŒãšbodyã¿ã°ã®æåŸã«ã¯ãjsãã¡ã€ã«ãšcssãã¡ã€ã«ãžã®ãªã³ã¯ããããŸãã ãããã®ãã¡ã€ã«èªäœã¯ããéçããã©ã«ããŒã«ãªããã°ãªããŸããã
HTMLãã¡ã€ã«<!doctype html> <html> <head> <meta charset="utf-8" /> <title>Handwritten digit recognition</title> <link rel="stylesheet" type="text/css" href="static/bootstrap.min.css"> <link rel="stylesheet" type="text/css" href="static/style.css"> </head> <body> <div class="container"> <div> . <canvas id="the_stage" width="200" height="200">fsf</canvas> <div> <button type="button" class="btn btn-default butt" onclick="clearCanvas()"><strong>clear</strong></button> <button type="button" class="btn btn-default butt" id="save" onclick="saveImg()"><strong>save</strong></button> </div> <div> Please select one of the following <input type="radio" name="action" value="0" id="digit">0 <input type="radio" name="action" value="1" id="digit">1 <input type="radio" name="action" value="2" id="digit">2 <input type="radio" name="action" value="3" id="digit">3 <input type="radio" name="action" value="4" id="digit">4 <input type="radio" name="action" value="5" id="digit">5 <input type="radio" name="action" value="6" id="digit">6 <input type="radio" name="action" value="7" id="digit">7 <input type="radio" name="action" value="8" id="digit">8 <input type="radio" name="action" value="9" id="digit">9 </div> </div> <div class="col-md-6 column"> <h3>result:</h3> <h2 id="rec_result"></h2> </div> </div> <script src="static/jquery.min.js"></script> <script src="static/bootstrap.min.js"></script> <script src="static/draw.js"></script> </body></html>
ãã£ã³ãã¹ã§ã®æç»æ¹æ³ãšæç»ã®ä¿åæ¹æ³ã«ã€ããŠè©³ãã説æããŸãã
Canvasã¯2次å
ã®HTML5æç»èŠçŽ ã§ãã ç»åã¯ã¹ã¯ãªããã䜿çšããŠæç»ããããšãããŠãŒã¶ãŒãããŠã¹ã䜿çšããŠïŒãŸãã¯ã¿ããã¹ã¯ãªãŒã³ã«è§ŠããŠïŒæç»ããããšãã§ããŸãã
Canvasã¯ãHTMLã§æ¬¡ã®ããã«å®çŸ©ãããŠããŸãã
<canvas id="the_stage" width="200" height="200"> </canvas>
ãã以åã¯ããã®HTMLèŠçŽ ã«æ
£ããŠããªãã£ããããæç»ãå¯èœã«ããæåã®è©Šã¿ã¯å€±æããŸããã ãã°ããããŠãå®çšçãªäŸãèŠã€ããŠåããŸããïŒãªã³ã¯ã¯draw.jsãã¡ã€ã«ã«ãããŸãïŒã
æç»çšã³ãŒã var drawing = false; var context; var offset_left = 0; var offset_top = 0; function start_canvas () { var canvas = document.getElementById ("the_stage"); context = canvas.getContext ("2d"); canvas.onmousedown = function (event) {mousedown(event)}; canvas.onmousemove = function (event) {mousemove(event)}; canvas.onmouseup = function (event) {mouseup(event)}; for (var o = canvas; o ; o = o.offsetParent) { offset_left += (o.offsetLeft - o.scrollLeft); offset_top += (o.offsetTop - o.scrollTop); } draw(); } function getPosition(evt) { evt = (evt) ? evt : ((event) ? event : null); var left = 0; var top = 0; var canvas = document.getElementById("the_stage"); if (evt.pageX) { left = evt.pageX; top = evt.pageY; } else if (document.documentElement.scrollLeft) { left = evt.clientX + document.documentElement.scrollLeft; top = evt.clientY + document.documentElement.scrollTop; } else { left = evt.clientX + document.body.scrollLeft; top = evt.clientY + document.body.scrollTop; } left -= offset_left; top -= offset_top; return {x : left, y : top}; } function mousedown(event) { drawing = true; var location = getPosition(event); context.lineWidth = 8.0; context.strokeStyle="#000000"; context.beginPath(); context.moveTo(location.x,location.y); } function mousemove(event) { if (!drawing) return; var location = getPosition(event); context.lineTo(location.x,location.y); context.stroke(); } function mouseup(event) { if (!drawing) return; mousemove(event); context.closePath(); drawing = false; } . . . onload = start_canvas;
æç»ã®ä»çµã¿ããŒãžãããŒãããããšãstart_canvas颿°ãèµ·åããŸãã æåã®2è¡ã¯ãç¹å®ã®IDïŒãã¹ããŒãžãïŒãæã€èŠçŽ ãšããŠãã£ã³ãã¹ãèŠã€ãã2次å
ç»åãšããŠå®çŸ©ããŸãã ãã£ã³ãã¹ã«æç»ããå Žåãonmousedownãonmousemoveãonmouseupã®3ã€ã®ã€ãã³ãããããŸãã ã¿ããã«ã€ããŠãåæ§ã®ã€ãã³ãããããŸãããããã«ã€ããŠã¯åŸã§è©³ãã説æããŸãã
onmousedown-ãã£ã³ãã¹ãã¯ãªãã¯ãããšçºçããŸãã ãã®æç¹ã§ãç·ã®å¹
ãšè²ãèšå®ãããæç»ã®éå§ç¹ã決å®ãããŸãã ã€ãŸããã«ãŒãœã«ã®äœçœ®ã決å®ããããšã¯ç°¡åã«èãããŸãããå®éã«ã¯å®å
šã«ç°¡åã§ã¯ãããŸããã getPositionïŒïŒé¢æ°ã¯ããã€ã³ããèŠã€ããããã«äœ¿çšãããŸã-ããŒãžäžã®ã«ãŒãœã«ã®åº§æšãèŠã€ããããŒãžäžã®ãã£ã³ãã¹ã®çžå¯Ÿäœçœ®ãèæ
®ããããŒãžãã¹ã¯ããŒã«ã§ããããšãèæ
®ããŠããã£ã³ãã¹äžã®ãã€ã³ãã®åº§æšã決å®ããŸãã ãã€ã³ããèŠã€ããåŸã context.beginPathïŒïŒã¯æç»ãã¹ãéå§ãã context.moveToïŒlocation.xãlocation.yïŒã¯ãã¯ãªãã¯æã«æ±ºå®ããããã€ã³ãã«ãã®ãã¹ããç§»åãããŸãã
onmousemove-å·Šãã¿ã³ãæŒãããŸãŸããŠã¹ã®åãã远跡ããŸãã æåã«ãããŒãæŒãããïŒã€ãŸããdrawing = trueïŒããšã確èªããŸãããããã§ãªãå Žåãæç»ã¯å®è¡ãããŸããã context.lineToïŒïŒã¯ããŠã¹ã®ãã¹ã«æ²¿ã£ãŠç·ãäœæãã context.strokeïŒïŒã¯ãããçŽæ¥æç»ããŸãã
onmouseup-ããŠã¹ã®å·Šãã¿ã³ãé¢ããããšçºçããŸãã context.closePathïŒïŒã¯ãç·ã®æç»ãå®äºããŸãã
ã€ã³ã¿ãŒãã§ãŒã¹ã«ã¯ããã«4ã€ã®èŠçŽ ããããŸãã
- çŸåšã®ã¹ããŒã¿ã¹ã®ããã£ãŒã«ããã JSã¯idïŒrec_resultïŒã§ã¢ã¯ã»ã¹ããçŸåšã®ã¹ããŒã¿ã¹ã衚瀺ããŸãã ã¹ããŒã¿ã¹ã¯ç©ºã§ããããç»åãä¿åãããŠããããšã瀺ãããä¿åãããç»åã®ååã瀺ããŸãã
<div class="col-md-6 column"> <h3>result:</h3> <h2 id="rec_result"></h2> </div>
function draw() { context.fillStyle = '#ffffff'; context.fillRect(0, 0, 200, 200); } function clearCanvas() { context.clearRect (0, 0, 200, 200); draw(); document.getElementById("rec_result").innerHTML = ""; }
ãã£ã³ãã¹ã®å
容ãã¯ãªã¢ãããçœã§å¡ãã€ã¶ãããŸãã ãŸããã¹ããŒã¿ã¹ã¯ç©ºã«ãªããŸãã
- æåŸã«ãæãããç»åãä¿åãããã¿ã³ã 次ã®JavaScript颿°ãåŒã³åºããŸãã
function saveImg() { document.getElementById("rec_result").innerHTML = "connecting..."; var canvas = document.getElementById("the_stage"); var dataURL = canvas.toDataURL('image/jpg'); var dig = document.querySelector('input[name="action"]:checked').value; $.ajax({ type: "POST", url: "/hook", data:{ imageBase64: dataURL, digit: dig } }).done(function(response) { console.log(response) document.getElementById("rec_result").innerHTML = response }); }
ãã¿ã³ãã¯ãªãã¯ããçŽåŸã«ãã¹ããŒã¿ã¹ãã£ãŒã«ãã«ãæ¥ç¶äž...ãã®å€ã衚瀺ãããŸãã ãã®åŸãç»åã¯base64ãšã³ã³ãŒãæ¹åŒã䜿çšããŠããã¹ãæååã«å€æãããŸãã çµæã¯æ¬¡ã®ãšããã§ãïŒ
data:image/png;base64,%string%
ããã¡ã€ã«ã¿ã€ãïŒimageïŒãæ¡åŒµåïŒpngïŒãbase64ãšã³ã³ãŒãã£ã³ã°ãããã³æååèªäœã確èªã§ããŸãã ããã§ãã³ãŒãã®ééãã«é
ãããããšã«æ°ä»ããŸããã
canvas.toDataURL()
åŒæ°ãšããŠãimage / jpegãã䜿çšããå¿
èŠããããŸããããã¿ã€ããã¹ãè¡ã£ãçµæãç»åã¯å®éã«ã¯pngã§ããã
次ã«ãã¢ã¯ãã£ãã©ãžãªãã¿ã³ã®å€ïŒåå= 'action'ã§
checked
ïŒãååŸãã
dig
倿°ã«ä¿åããŸãã
æåŸã«ãAJAXèŠæ±ã¯ãšã³ã³ãŒããããç»åãšã©ãã«ãpythonã«éä¿¡ããå¿çãåãåããŸãã ãã®èšèšãæ©èœãããããã«å€ãã®æéãè²»ãããŸãããåè¡ã§äœãèµ·ãããã説æããããšããŸãã
ãŸãããªã¯ãšã¹ãã®ã¿ã€ãã瀺ãããŸã-ãã®å ŽåããPOSTããã€ãŸããJSããã®ããŒã¿ãpythonã¹ã¯ãªããã«è»¢éãããŸãã
ã/ããã¯ãã¯ãããŒã¿ã転éãããå Žæã§ãã Flaskã䜿çšããŠãããããå¿
èŠãªãã³ã¬ãŒã¿ãŒã§URLãšããŠã/ hookããæå®ã§ããŸããããã¯ãPOSTèŠæ±ããã®URLã«éä¿¡ããããšãã«äœ¿çšãããã®ã¯ãã®ãã³ã¬ãŒã¿ãŒã®é¢æ°ã§ããããšãæå³ããŸãã 詳现ã«ã€ããŠã¯ã以äžã®ãã©ã¹ã³ã®ã»ã¯ã·ã§ã³ãåç
§ããŠãã ããã
data
ã¯ããªã¯ãšã¹ãã§éä¿¡ãããããŒã¿ã§ãã ããŒã¿ã®éä¿¡ã«ã¯å€ãã®ãªãã·ã§ã³ããããŸãããã®å Žåãå€ãšããã®å€ãååŸã§ããååãèšå®ããŸãã
æåŸã«ã
done()
ã¯ãªã¯ãšã¹ããæåãããšãã«èµ·ããããšã§ãã ç§ã®AJAXãªã¯ãšã¹ãã¯ç¹å®ã®åçïŒããæ£ç¢ºã«ã¯ãä¿åãããç»åã®ååãå«ãããã¹ãïŒãè¿ããŸãããã®åçã¯ãæåã«ã³ã³ãœãŒã«ã«è¡šç€ºããïŒãããã°çšïŒã次ã«ã¹ããŒã¿ã¹ãã£ãŒã«ãã«è¡šç€ºãããŸãã
æ°ãæ£ããããã«ããããžã§ã¯ãã®äœæ¥ã®éå§æã«çããå°ããªåé¡ã«ã€ããŠã話ããããšæããŸãã äœããã®çç±ã§ããã®ç»ååŠçã®åºæ¬çãªãµã€ãã®èªã¿èŸŒã¿ã«ã¯çŽ1åããããŸããã æ°è¡ã ããæ®ããŠæå°åããããšããŸããããäœãå©ãã«ã¯ãªããŸããã§ããã ãã®çµæãã³ã³ãœãŒã«ã®ãããã§ããã¬ãŒãã®åå ãç¹å®ããããšãã§ããŸãã-ã¢ã³ããŠã€ã«ã¹ã

Kasperskyãèªåã®ãµã€ãã«ã¹ã¯ãªãããæ¿å
¥ãããããããŠã³ããŒãã«æéãããããŸããã ãªãã·ã§ã³ãç¡å¹ã«ããåŸããµã€ãã¯ããã«ããŒããå§ããŸããã
ãã©ã¹ã³ãšç»åä¿å
ããã§ã¯ãAJAXãªã¯ãšã¹ãããã®ããŒã¿ãã©ã®ããã«pythonã«éãããç»åãã©ã®ããã«ä¿åãããããèŠãŠãããŸãããã
Flaskã®æäœã«é¢ããã¬ã€ããèšäºã¯å€æ°ãããŸãããã®ãããåºæ¬çãªããšãç°¡åã«èª¬æããã³ãŒããæ©èœããªãè¡ã«ç¹ã«æ³šæãæããŸãããã¡ããããµã€ãã®åºæ¬ã§ããæ®ãã®ã³ãŒãã«ã€ããŠã説æããŸãã
main.py __author__ = 'Artgor' from functions import Model from flask import Flask, render_template, request from flask_cors import CORS, cross_origin import base64 import os app = Flask(__name__) model = Model() CORS(app, headers=['Content-Type']) @app.route("/", methods=["POST", "GET", 'OPTIONS']) def index_page(): return render_template('index.html') @app.route('/hook', methods = ["GET", "POST", 'OPTIONS']) def get_image(): if request.method == 'POST': image_b64 = request.values['imageBase64'] drawn_digit = request.values['digit'] image_encoded = image_b64.split(',')[1] image = base64.decodebytes(image_encoded.encode('utf-8')) save = model.save_image(drawn_digit, image) print('Done') return save if __name__ == '__main__': port = int(os.environ.get("PORT", 5000)) app.run(host='0.0.0.0', port=port, debug=False)
functions.py __author__ = 'Artgor' from codecs import open import os import uuid import boto3 from boto.s3.key import Key from boto.s3.connection import S3Connection class Model(object): def __init__(self): self.nothing = 0 def save_image(self, drawn_digit, image): filename = 'digit' + str(drawn_digit) + '__' + str(uuid.uuid1()) + '.jpg' with open('tmp/' + filename, 'wb') as f: f.write(image) print('Image written') REGION_HOST = 's3-external-1.amazonaws.com' conn = S3Connection(os.environ['AWS_ACCESS_KEY_ID'], os.environ['AWS_SECRET_ACCESS_KEY'], host=REGION_HOST) bucket = conn.get_bucket('testddr') k = Key(bucket) fn = 'tmp/' + filename k.key = filename k.set_contents_from_filename(fn) print('Done') return ('Image saved successfully with the name {0}'.format(filename))
ã³ãŒãã®ä»çµã¿æåã®ã¹ãããã¯ãããã©ã«ãå€app = Flask(__name__)
Flaskã¯ã©ã¹ã®ã€ã³ã¹ã¿ã³ã¹ãäœæããããšã§ãã ãããã¢ããªã±ãŒã·ã§ã³ã®åºç€ã«ãªããŸãã
次ã«ã2çªç®ã®ã¹ã¯ãªããã䜿çšããã¯ã©ã¹ã®ã€ã³ã¹ã¿ã³ã¹ãäœæããŸãã 颿°ã1ã€ãããªãå Žåãã¯ã©ã¹ã䜿çšããã«å®è¡ããããšãã1ã€ã®ã¹ã¯ãªããã§ãã¹ãŠã®ã³ãŒããä¿æããããšãã§ããŸãã ããããã¡ãœããã®æ°ãå¢ããããšã¯ããã£ãŠããã®ã§ãããã«ãã®ãªãã·ã§ã³ã䜿çšããããšã«ããŸããã
CORSïŒã¯ãã¹ãªãªãžã³ãªãœãŒã¹å
±æïŒã¯ãWebããŒãžã«å¥ã®ãã¡ã€ã³ã®ãªãœãŒã¹ãžã®ã¢ã¯ã»ã¹ãæäŸããæè¡ã§ãã ãã®ã¢ããªã±ãŒã·ã§ã³ã§ã¯ãããã䜿çšããŠAmazonã¯ã©ãŠãã«ç»åãä¿åããŸãã ç§ã¯é·ãéãã®èšå®ãæå¹ã«ããæ¹æ³ãæ¢ããŠããŸãããããããç°¡åã«ããæ¹æ³ãæ¢ããŠããŸããã ãã®çµæã CORS(app, headers=['Content-Type'])
1è¡ã§å®è£
ããŸããã
次ã«ã route()
ãã³ã¬ãŒã¿ã䜿çšãããŸã-ã©ã®é¢æ°ãã©ã®URLã«å¯ŸããŠå®è¡ãããããæ±ºå®ããŸãã ã¡ã€ã³ã¹ã¯ãªããã«ã¯ã颿°ãæã€2ã€ã®ãã³ã¬ãŒã¿ããããŸãã æåã®ããŒãžã¯ã¡ã€ã³ããŒãžã«äœ¿çšããïŒã¢ãã¬ã¹ã¯"/"
ïŒã "index.html"ã衚瀺ããŸãã 2çªç®ã®ãã³ã¬ãŒã¿ãŒã«ã¯ã¢ãã¬ã¹ã "/ hook"ãããããŸããããã¯ãJSããã®ããŒã¿ãããã«è»¢éãããããšãæå³ããŸãïŒåãã¢ãã¬ã¹ãããã«æå®ãããããšãæãåºããŸãïŒã
ã©ã¡ãã®ãã³ã¬ãŒã¿ãŒã«ããã¡ãœãããã©ã¡ãŒã¿ãŒã®å€ãšããŠãPOSTãããGETãããOPTIONSãã®ãªã¹ããããããšã«æ³šæããŠãã ããã POSTãšGETã¯ããŒã¿ã®éåä¿¡ã«äœ¿çšããïŒäž¡æ¹ãšã念ã®ããïŒãCORSãªã©ã®ãã©ã¡ãŒã¿ãŒãæž¡ãã«ã¯OPTIONSãå¿
èŠã§ãã çè«çã«ã¯ãFlaskããŒãžã§ã³0.6ããOPTIONSãããã©ã«ãã§äœ¿çšããå¿
èŠããããŸãããæç€ºçãªæç€ºããªããšãã³ãŒããåäœãããããšãã§ããŸããã§ããã
次ã«ãç»åãååŸããæ©èœã«ã€ããŠèª¬æããŸãã JSããPythonãžã®ããªã¯ãšã¹ããããããŸãã ããã¯ããã©ãŒã ããã®ããŒã¿ãAJAXããååŸããããŒã¿ãªã©ã§ãã ç§ã®å Žåãããã¯ããŒãšå€ãJSã§æå®ãããèŸæžã§ãã ç»åã®ã©ãã«ã®æœåºã¯ç°¡åã§ãããŒããšã«èŸæžã®å€ãååŸããå¿
èŠããããŸãããç»åãå«ãæååãåŠçããå¿
èŠããããŸã-ç»åèªäœã«é¢é£ããéšåãååŸãïŒèª¬æãç Žæ£ïŒããã³ãŒãããŸãã
ãã®åŸã2çªç®ã®ã¹ã¯ãªããã®é¢æ°ãåŒã³åºãããç»åãä¿åãããŸãã ä¿åãããç»åã®ååãå«ãæååãè¿ããŸãããã®åŸãJSã«æ»ãããã©ãŠã¶ããŒãžã«è¡šç€ºãããŸãã
ã³ãŒãã®æåŸã®éšåã¯ãã¢ããªã±ãŒã·ã§ã³ãherokuã§åäœããããã«å¿
èŠã§ãïŒããã¥ã¡ã³ãããååŸïŒã Herokuã«ã¢ããªã±ãŒã·ã§ã³ãé
眮ããæ¹æ³ã«ã€ããŠã¯ã察å¿ããã»ã¯ã·ã§ã³ã§è©³ãã説æããŸãã
æåŸã«ãç»åãã©ã®ããã«ä¿åãããããèŠãŠã¿ãŸãããã çŸåšãç»åã¯å€æ°ã«ä¿åãããŠããŸãããç»åãAmazonã¯ã©ãŠãã«ä¿åããã«ã¯ãã¡ã€ã«ãå¿
èŠãªã®ã§ãç»åãä¿åããå¿
èŠããããŸãã ç»åã®ååã«ã¯ãã©ãã«ãšãuuidã䜿çšããŠçæãããäžæã®IDãå«ãŸããŸãã ãã®åŸãç»åã¯äžæçãªtmpãã©ã«ããŒã«ä¿åãããã¯ã©ãŠãã«ã¢ããããŒããããŸãã tmpãã©ã«ããŒå
ã®ãã¡ã€ã«ã¯äžæçãªãã®ã§ãããšããã«èšã£ãŠããå¿
èŠããããŸããherokuã¯ã»ãã·ã§ã³äžã«æ°ãã/倿Žããããã¡ã€ã«ãä¿åããçµäºæã«ãã¡ã€ã«ãåé€ãããã倿ŽãããŒã«ããã¯ããŸãã ããã«ããããã©ã«ããŒãã¯ãªã¢ããå¿
èŠæ§ã«ã€ããŠèããå¿
èŠããªããªããäžè¬çã«äŸ¿å©ã§ãããã¯ã©ãŠãã䜿çšããå¿
èŠãããã®ã¯ãŸãã«ãã®ããã§ãã
ããã§ãAmazonã¯ã©ãŠãã®ä»çµã¿ãšãããã䜿ã£ãŠäœãã§ãããã«ã€ããŠè©±ãããšãã§ããŸãã
Amazon S3ã®çµ±å
Pythonã«ã¯ãbotoãšboto3ã®2ã€ã®Amazonã¯ã©ãŠãã©ã€ãã©ãªããããŸãã 2çªç®ã®æ¹æ³ã¯ããæ°ãããããé©åã«ãµããŒããããŠããŸããããããŸã§ã®ãšãããæåã®æ¹æ³ã䜿çšããæ¹ã䟿å©ãªå ŽåããããŸãã
Amazonã§ãã±ãããæ§ç¯ããã³æ§æããã¢ã«ãŠã³ããç»é²ããŠãåé¡ã¯ãªããšæããŸãã ã©ã€ãã©ãªã䜿çšããŠã¢ã¯ã»ã¹ããããã®ããŒïŒã¢ã¯ã»ã¹ããŒIDãšã·ãŒã¯ã¬ããã¢ã¯ã»ã¹ããŒïŒãçæããããšãå¿ããªãããšãéèŠã§ãã ã¯ã©ãŠãèªäœã§ããã¡ã€ã«ãä¿åãããã±ãããäœæã§ããŸãã ãã·ã¢èªã§ã¯ããã±ãã¯ãã±ãã§ãããç§ã«ã¯å¥åŠã«èãããã®ã§ãå
ã®ååã䜿çšããããšã奜ã¿ãŸãã
ãããŠä»ããã¥ã¢ã³ã¹ã¯ãªããªã£ãŠããŸãã

äœæãããšãã¯ãååãæå®ããå¿
èŠããããããã§ã¯æ³šæããå¿
èŠããããŸãã æåã¯ããã€ãã³ã§ã€ãªãããååã䜿çšããŸããããã¯ã©ãŠãã«æ¥ç¶ã§ããŸããã§ããã ããã€ãã®åã
ã®ãã£ã©ã¯ã¿ãŒããã®åé¡ãåŒãèµ·ããããšã倿ããŸããã åé¿çã¯ãããŸããããã¹ãŠã®äººã«åœ¹ç«ã€ããã§ã¯ãããŸããã ãã®çµæãã¢ã³ããŒã¹ã³ã¢ïŒdigit_draw_recognizeïŒã§ååã®å€åœ¢ã䜿çšãå§ããŸããããåé¡ã¯ãããŸããã§ããã
次ã«ãå°åãæå®ããå¿
èŠããããŸãã ã»ãšãã©ãã¹ãŠãéžæã§ããŸããããã®
ãã¬ãŒãã«ã¬ã€ããããå¿
èŠããããŸãã
ãŸããéžæãããªãŒãžã§ã³ã«ãšã³ããã€ã³ããå¿
èŠã§ããæ¬¡ã«ã䟿å©ãªãã®ã䜿çšã§ããããã«ã2ããã³4ããŒãžã§ã³ã®çœ²åããµããŒããããªãŒãžã§ã³ã䜿çšããæ¹ãç°¡åã§ãã ç±³åœæ±éšïŒããŒãžãã¢åéšïŒãéžã³ãŸããã
ãã±ããäœææã®ä»ã®ãã©ã¡ãŒã¿ãŒã¯å€æŽã§ããŸããã
ãã1ã€ã®éèŠãªãã€ã³ãã¯ãCORSã®æ§æã§ãã
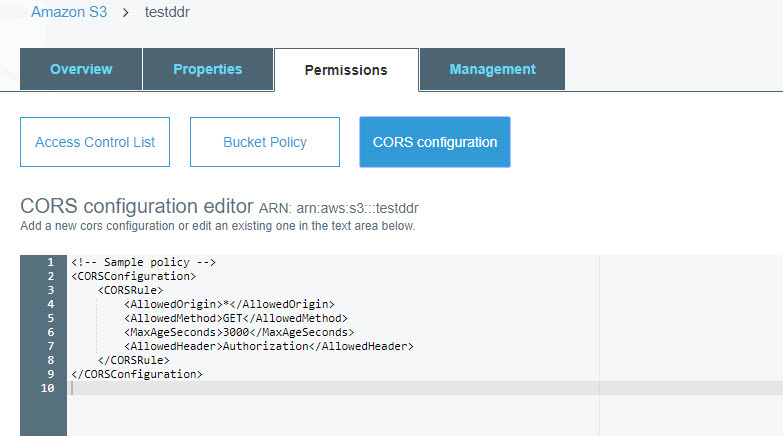
次ã®ã³ãŒããæ§æã«å
¥åããå¿
èŠããããŸãã
<CORSConfiguration> <CORSRule> <AllowedOrigin>*</AllowedOrigin> <AllowedMethod>GET</AllowedMethod> <MaxAgeSeconds>3000</MaxAgeSeconds> <AllowedHeader>Authorization</AllowedHeader> </CORSRule> </CORSConfiguration>
ãã®èšå®ã®è©³çްã«ã€ããŠã¯ãã¡ãã
ã芧ãã ããããœãã/ã¿ããªãã©ã¡ãŒã¿ãŒãèšå®ã§ããŸãã æåã¯ãïŒGETã ãã§ãªãïŒããå€ãã®ã¡ãœãããæå®ããå¿
èŠããããšæããŸãããããã¹ãŠãã®ããã«åäœããŸããã
ããŠãå®éã«ã³ãŒãã«ã€ããŠè©±ãã ããã§ã¯ããã¡ã€ã«ãAmazonã«ä¿åããæ¹æ³ã®ã¿ã«çŠç¹ãåœãŠãŸãã ã¯ã©ãŠãããã®ããŒã¿ã®åä¿¡ã«ã€ããŠã¯ãå°ãåŸã§èª¬æããŸãã
REGION_HOST = 's3-external-1.amazonaws.com' conn = S3Connection(os.environ['AWS_ACCESS_KEY_ID'], os.environ['AWS_SECRET_ACCESS_KEY'], host=REGION_HOST) bucket = conn.get_bucket('testddr') k = Key(bucket) fn = 'tmp/' + filename k.key = filename k.set_contents_from_filename(fn)
æåã®ã¹ãããã¯ãã¯ã©ãŠãæ¥ç¶ãäœæããããšã§ãã ãããè¡ãã«ã¯ãã¢ã¯ã»ã¹ããŒIDãã·ãŒã¯ã¬ããã¢ã¯ã»ã¹ããŒãããã³ãªãŒãžã§ã³ãã¹ããæå®ããŸãã ããŒã¯ãã§ã«çæãããŠããŸãããæç€ºçã«æå®ããããšã¯å±éºã§ãã ããã¯ãã³ãŒããããŒã«ã«ã§ãã¹ããããšãã«ã®ã¿å®è¡ã§ããŸãã å
¬ç¶ãšæå®ãããããŒã䜿çšããŠgithubã³ãŒãã«æ°åã³ãããããŸãã-ãããã¯æ°åéçãŸããŸããã ç§ã®ééããç¹°ãè¿ããªãã§ãã ããã Herokuã«ã¯ãããŒãä¿åããååã§ã¢ã¯ã»ã¹ããæ©èœããããŸãã詳现ã«ã€ããŠã¯ã以äžãã芧ãã ããã ãªãŒãžã§ã³ãã¹ãã¯ããªãŒãžã§ã³ã©ãã«ã®ãšã³ããã€ã³ãå€ã§ãã
ãã®åŸããã±ããã«æ¥ç¶ããå¿
èŠããããŸã-ããã§ã¯ãååãçŽæ¥æå®ããããšã¯å®å
šã«åãå
¥ããããŸãã
Key
ããã¹ã±ããå
ã®ãªããžã§ã¯ããæäœããããã«äœ¿çšãããŸãã ãªããžã§ã¯ããä¿åããã«ã¯ããã¡ã€ã«åïŒk.keyïŒãæå®ããä¿åãããã¡ã€ã«ãžã®ãã¹ã§
k.set_contents_from_filename()
ãåŒã³åºãå¿
èŠããããŸãã
ããã¯
Herokuã§ãµã€ãããã¹ãããæ¹æ³ã«ã€ããŠè©±ããŸãã Herokuã¯ãWebã¢ããªã±ãŒã·ã§ã³ãè¿
éãã€äŸ¿å©ã«å±éã§ããå€èšèªã¯ã©ãŠããã©ãããã©ãŒã ã§ãã Postgresãšå€ãã®è峿·±ããã®ã䜿çšããããšãå¯èœã§ãã äžè¬çã«ãHerokuãªãœãŒã¹ã䜿çšããŠç»åãä¿åã§ããŸãããç°ãªãçš®é¡ã®ããŒã¿ãä¿åããå¿
èŠããããããå¥ã®ã¯ã©ãŠãã䜿çšããæ¹ãç°¡åã§ããã
Herokuã«ã¯ããã€ãã®äŸ¡æ Œãã©ã³ããããŸãããç§ã®ã¢ããªã±ãŒã·ã§ã³ïŒãã®å°ããªãã©ã³ã§ã¯ãªããæ¬æ Œçãªãã©ã³ãå«ãïŒã«ã¯ãç¡æã®ãã©ã³ã§ååã§ãã å¯äžã®ãã€ãã¹ç¹ã¯ã30åéã®ã¢ã¯ãã£ããã£ã®åŸãã¢ããªã±ãŒã·ã§ã³ããã¹ãªãŒããç¶æ
ã«ãªããæ¬¡ã«èµ·åãããšãã«ããŠã§ã€ã¯ã¢ãããã«30ç§ãè²»ããããšãã§ããããšã§ãã
ãããã¯ãŒã¯äžã®Herokuã«ã¢ããªã±ãŒã·ã§ã³ããããã€ããããã®ã¬ã€ãã¯å€æ°ãããŸãïŒ
å
¬åŒãªã³ã¯ãžã®ãªã³ã¯ã¯ãã¡ãïŒãããããã®ã»ãšãã©ã¯ã³ã³ãœãŒã«ã䜿çšããŠãããã€ã³ã¿ãŒãã§ã€ã¹ã䜿çšããããšã奜ã¿ãŸãã ããã«ãç§ã«ãšã£ãŠã¯ãã£ãšã·ã³ãã«ã§äŸ¿å©ãªããã§ãã
Herokuã§ã®ã¢ããªãã¹ãã£ã³ã°ã ãããã€ã³ãã«ã ã¢ããªã±ãŒã·ã§ã³ãæºåããã«ã¯ãããã€ãã®ãã¡ã€ã«ãäœæããå¿
èŠããããŸãã
- ããŒãžã§ã³ç®¡çã·ã¹ãã .gitãå¿
èŠã§ãã éåžžãgithubãªããžããªãäœæãããšèªåçã«äœæãããŸãããå®éã«äœæãããŠããããšã確èªããå¿
èŠããããŸãã
runtime.txt
ãã®ãã¡ã€ã«ã§ã¯ãããã°ã©ãã³ã°èšèªã®å¿
èŠãªããŒãžã§ã³ã瀺ãå¿
èŠããããŸããç§ã®å Žåã¯python-3.6.1ãProcfile
ã¯ãæ¡åŒµåã®ãªããã¡ã€ã«ã§ãã herokuã§å®è¡ããã³ãã³ãã瀺ããŸãã ããã«ãããéå§ããweb
ããã»ã¹ã®ã¿ã€ãïŒç§ã®ã¹ã¯ãªããïŒãšã¢ãã¬ã¹ã決ãŸããŸãã
web: python main.py runserver 0.0.0.0:5000
requirements.txt
-Herokuã«ã€ã³ã¹ããŒã«ãããã©ã€ãã©ãªã®ãªã¹ãã å¿
èŠãªããŒãžã§ã³ã瀺ãããšããå§ãããŸãã- æåŸã®-å°ãªããšã1ã€ã®ãã¡ã€ã«ããtmpããã©ã«ããŒã«ããå¿
èŠããããŸããããã§ãªãå Žåãã¢ããªã±ãŒã·ã§ã³æäœäžã«ãã®ãã©ã«ããŒã«ãã¡ã€ã«ãä¿åããéã«åé¡ãçºçããå¯èœæ§ããããŸãã
ããã§ãã¢ããªã±ãŒã·ã§ã³ã®äœæããã»ã¹ãéå§ã§ããŸãã ãã®æç¹ã§ãherokuã®ã¢ã«ãŠã³ããšæºåæžã¿ã®githubãªããžããªãå¿
èŠã§ãã
ã¢ããªã±ãŒã·ã§ã³ã®ãªã¹ããå«ã
ããŒãžãããæ°ãããã®ãäœæãããŸãã

ååãšåœã瀺ããŸãã

[ãããã€]ã¿ãã§ãGithubãžã®æ¥ç¶ãéžæããŸãã
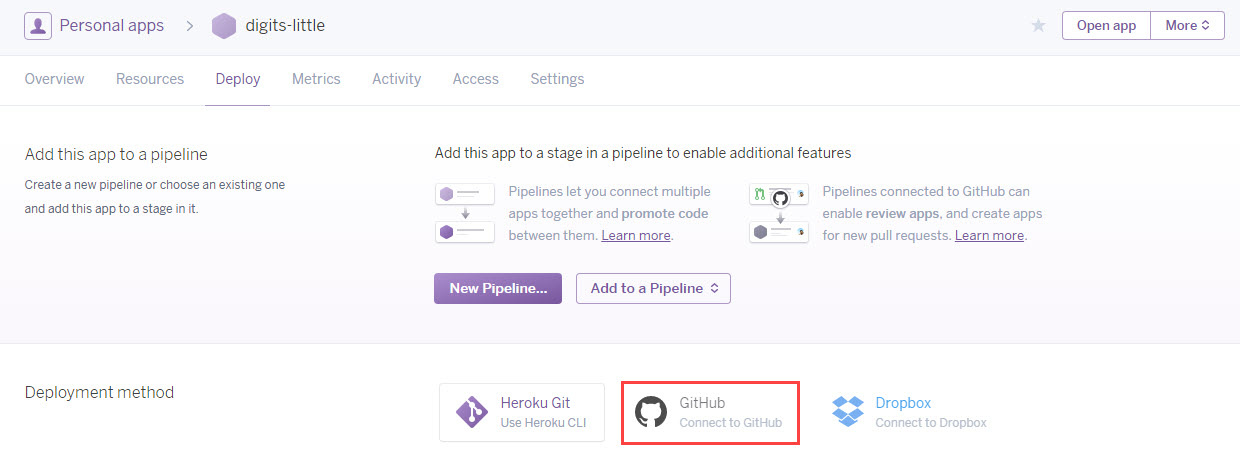
ãªããžããªãæ¥ç¶ããŠéžæããŸãã
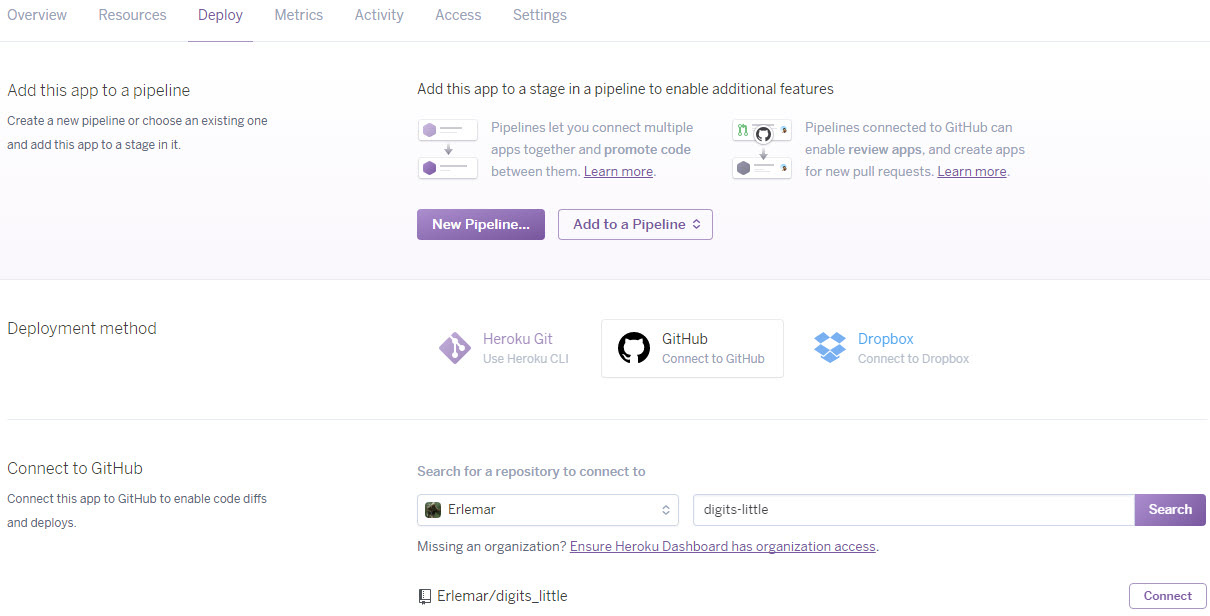
ãªããžããªãæŽæ°ããããã³ã«Herokuã§ã¢ããªã±ãŒã·ã§ã³ã®èªåæŽæ°ãæå¹ã«ããããšã¯çã«ããªã£ãŠããŸãã ãããŠãå±éãéå§ã§ããŸãã
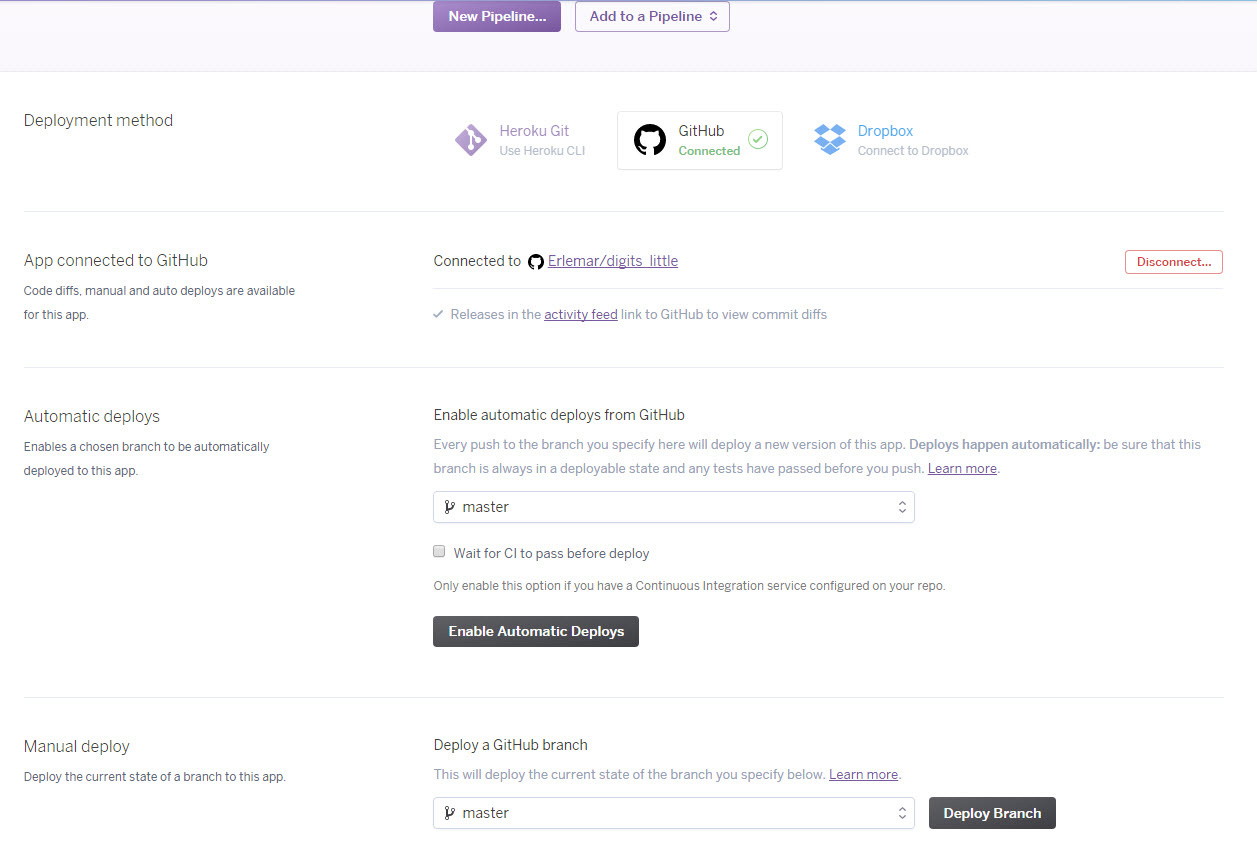
ãã°ãèŠãŠããã¹ãŠãããŸããã£ãããšãé¡ã£ãŠããŸãã

ãããããã¹ãŠããŸã æºåãã§ããŠããããã§ã¯ãããŸãããèšå®ã¿ãã§ã¢ããªã±ãŒã·ã§ã³ãæåã«èµ·åããåã«ã倿°-Amazonã¯ã©ãŠãã®ããŒãèšå®ããå¿
èŠããããŸãã

ããã§ãã¢ããªã±ãŒã·ã§ã³ãå®è¡ããŠæäœã§ããããã«ãªããŸããã ããã§ãããŒã¿åéãµã€ãã®èª¬æã¯å®äºã§ãã ããã«ãããŒã¿åŠçãšãããžã§ã¯ãã®æ¬¡ã®æ®µéã«çŠç¹ãåœãŠãŸãã
ã¢ãã«ã®ç»ååŠç
æç»ãããç»åãå
ã®åœ¢åŒã§ç»åãšããŠä¿åããŸããããã«ãããåžžã«ãããã衚瀺ããŠãããŸããŸãªåŠçãªãã·ã§ã³ã詊ãããšãã§ããŸãã
以äžã«äŸã瀺ããŸãã
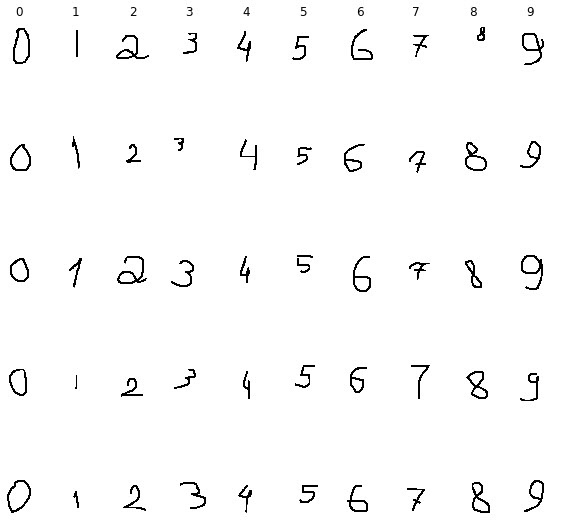
ç§ã®ãããžã§ã¯ãã®ç»ååŠçã®ã¢ã€ãã¢ã¯æ¬¡ã®ãšããã§ãïŒmnistã«äŒŒãŠããŸãïŒïŒæãããæ°åã¯ã20x20ã®æ£æ¹åœ¢ã«åãŸãããã«ã¹ã±ãŒãªã³ã°ãããæ¯çãä¿ããã28x28ã®å€§ããã®çœãæ£æ¹åœ¢ã®äžå€®ã«é
眮ãããŸãã ãããè¡ãã«ã¯ãæ¬¡ã®æé ãå¿
èŠã§ãã
- ç»åã®å¢çç·ãèŠã€ããïŒå¢çç·ã¯é·æ¹åœ¢ã®åœ¢ãããŠããŸãïŒ;
- å¢çç©åœ¢ã®é«ããšå¹
ãèŠã€ããŸãã
- 倧ããåŽã20ã«çããããå°ããåŽãã¹ã±ãŒãªã³ã°ããŠæ¯çãç¶æããŸãã
- 28x28ã®æ£æ¹åœ¢ã«ã¹ã±ãŒãªã³ã°ãããå³åœ¢ãæç»ããããã®éå§ç¹ãèŠã€ããŠæç»ããŸãã
- ããŒã¿ãæäœããŠæ£èŠåã§ããããã«ãnp.arrayã«å€æããŸãã
ç»ååŠç # img = Image.open('tmp/' + filename) # bbox = Image.eval(img, lambda px: 255-px).getbbox() if bbox == None: return None # widthlen = bbox[2] - bbox[0] heightlen = bbox[3] - bbox[1] # if heightlen > widthlen: widthlen = int(20.0 * widthlen / heightlen) heightlen = 20 else: heightlen = int(20.0 * widthlen / heightlen) widthlen = 20 # hstart = int((28 - heightlen) / 2) wstart = int((28 - widthlen) / 2) # img_temp = img.crop(bbox).resize((widthlen, heightlen), Image.NEAREST) # new_img = Image.new('L', (28,28), 255) new_img.paste(img_temp, (wstart, hstart)) # np.array imgdata = list(new_img.getdata()) img_array = np.array([(255.0 - x) / 255.0 for x in imgdata])
ç»åå¢åŒ·
æããã«ã1000åã®ç»åã¯ãã¥ãŒã©ã«ãããã¯ãŒã¯ã®ãã¬ãŒãã³ã°ã«ã¯äžååã§ããããããã®äžéšã¯ã¢ãã«ã®æ€èšŒã«äœ¿çšããå¿
èŠããããŸãã ãã®åé¡ã®è§£æ±ºçã¯ãããŒã¿ã®å¢åŒ·ã§ããããŒã¿ã»ããã®ãµã€ãºãå¢ããã®ã«åœ¹ç«ã€è¿œå ã®ç»åãäœæããŸãã , , . .
, . 2020 . 4 â 4 .
. , â 30 ; 5 , 12 . , 12 6.
# image = Image.open('tmp/' + filename) # ims_add = [] labs_add = [] # , angles = np.arange(-30, 30, 5) # bbox = Image.eval(image, lambda px: 255-px).getbbox() # widthlen = bbox[2] - bbox[0] heightlen = bbox[3] - bbox[1] # if heightlen > widthlen: widthlen = int(20.0 * widthlen/heightlen) heightlen = 20 else: heightlen = int(20.0 * widthlen/heightlen) widthlen = 20 # hstart = int((28 - heightlen) / 2) wstart = int((28 - widthlen) / 2) # 4 for i in [min(widthlen, heightlen), max(widthlen, heightlen)]: for j in [min(widthlen, heightlen), max(widthlen, heightlen)]: # resized_img = image.crop(bbox).resize((i, j), Image.NEAREST) # resized_image = Image.new('L', (28,28), 255) resized_image.paste(resized_img, (wstart, hstart)) # 6 12. angles_ = random.sample(set(angles), 6) for angle in angles_: # transformed_image = transform.rotate(np.array(resized_image), angle, cval=255, preserve_range=True).astype(np.uint8) labs_add.append(int(label)) # img_temp = Image.fromarray(np.uint8(transformed_image)) # np.array imgdata = list(img_temp.getdata()) normalized_img = [(255.0 - x) / 255.0 for x in imgdata] ims_add.append(normalized_img)
:
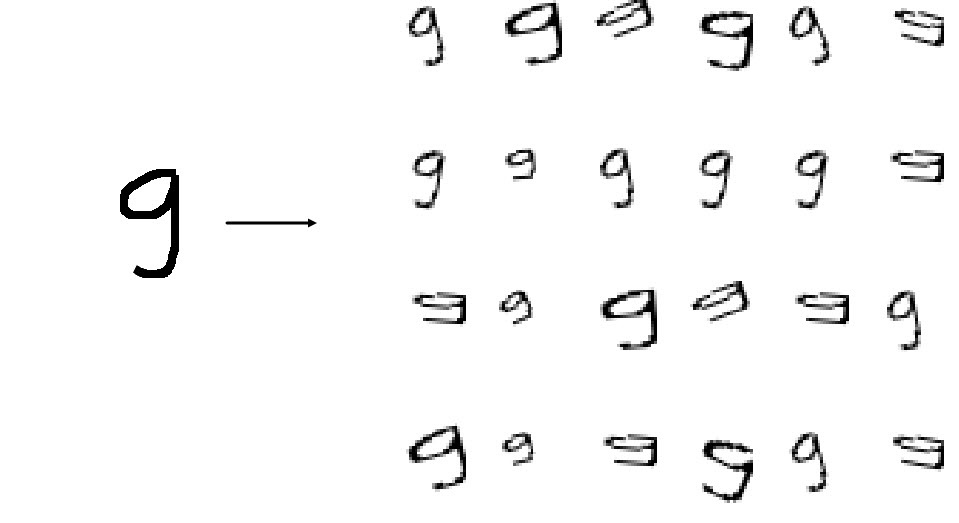
800 * 24 = 19200 200 . .
FNN
feed forward neural net. , cs231n, .
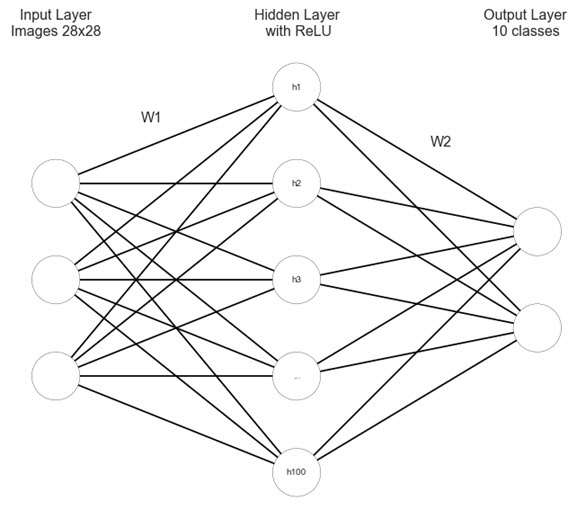
import matplotlib.pyplot as plt #https://gist.github.com/anbrjohn/7116fa0b59248375cd0c0371d6107a59 def draw_neural_net(ax, left, right, bottom, top, layer_sizes, layer_text=None): ''' :parameters: - ax : matplotlib.axes.AxesSubplot The axes on which to plot the cartoon (get eg by plt.gca()) - left : float The center of the leftmost node(s) will be placed here - right : float The center of the rightmost node(s) will be placed here - bottom : float The center of the bottommost node(s) will be placed here - top : float The center of the topmost node(s) will be placed here - layer_sizes : list of int List of layer sizes, including input and output dimensionality - layer_text : list of str List of node annotations in top-down left-right order ''' n_layers = len(layer_sizes) v_spacing = (top - bottom)/float(max(layer_sizes)) h_spacing = (right - left)/float(len(layer_sizes) - 1) ax.axis('off') # Nodes for n, layer_size in enumerate(layer_sizes): layer_top = v_spacing*(layer_size - 1)/2. + (top + bottom)/2. for m in range(layer_size): x = n*h_spacing + left y = layer_top - m*v_spacing circle = plt.Circle((x,y), v_spacing/4., color='w', ec='k', zorder=4) ax.add_artist(circle) # Node annotations if layer_text: text = layer_text.pop(0) plt.annotate(text, xy=(x, y), zorder=5, ha='center', va='center') # Edges for n, (layer_size_a, layer_size_b) in enumerate(zip(layer_sizes[:-1], layer_sizes[1:])): layer_top_a = v_spacing*(layer_size_a - 1)/2. + (top + bottom)/2. layer_top_b = v_spacing*(layer_size_b - 1)/2. + (top + bottom)/2. for m in range(layer_size_a): for o in range(layer_size_b): line = plt.Line2D([n*h_spacing + left, (n + 1)*h_spacing + left], [layer_top_a - m*v_spacing, layer_top_b - o*v_spacing], c='k') ax.add_artist(line) node_text = ['','','','h1','h2','h3','...', 'h100'] fig = plt.figure(figsize=(8, 8)) ax = fig.gca() draw_neural_net(ax, .1, .9, .1, .9, [3, 5, 2], node_text) plt.text(0.1, 0.95, "Input Layer\nImages 28x28", ha='center', va='top', fontsize=16) plt.text(0.26, 0.78, "W1", ha='center', va='top', fontsize=16) plt.text(0.5, 0.95, "Hidden Layer\n with ReLU", ha='center', va='top', fontsize=16) plt.text(0.74, 0.74, "W2", ha='center', va='top', fontsize=16) plt.text(0.88, 0.95, "Output Layer\n10 classes", ha='center', va='top', fontsize=16) plt.show()
FNN import numpy as np class TwoLayerNet(object): def __init__(self, input_size, hidden_size, output_size, std): self.params = {} self.params['W1'] = (((2 / input_size) ** 0.5) * np.random.randn(input_size, hidden_size)) self.params['b1'] = np.zeros(hidden_size) self.params['W2'] = (((2 / hidden_size) ** 0.5) * np.random.randn(hidden_size, output_size)) self.params['b2'] = np.zeros(output_size) def loss(self, X, y=None, reg=0.0): # Unpack variables from the params dictionary W1, b1 = self.params['W1'], self.params['b1'] W2, b2 = self.params['W2'], self.params['b2'] N, D = X.shape # Compute the forward pass l1 = X.dot(W1) + b1 l1[l1 < 0] = 0 l2 = l1.dot(W2) + b2 exp_scores = np.exp(l2) probs = exp_scores / np.sum(exp_scores, axis=1, keepdims=True) scores = l2 # Compute the loss W1_r = 0.5 * reg * np.sum(W1 * W1) W2_r = 0.5 * reg * np.sum(W2 * W2) loss = -np.sum(np.log(probs[range(y.shape[0]), y]))/N + W1_r + W2_r # Backward pass: compute gradients grads = {} probs[range(X.shape[0]),y] -= 1 dW2 = np.dot(l1.T, probs) dW2 /= X.shape[0] dW2 += reg * W2 grads['W2'] = dW2 grads['b2'] = np.sum(probs, axis=0, keepdims=True) / X.shape[0] delta = probs.dot(W2.T) delta = delta * (l1 > 0) grads['W1'] = np.dot(XT, delta)/ X.shape[0] + reg * W1 grads['b1'] = np.sum(delta, axis=0, keepdims=True) / X.shape[0] return loss, grads def train(self, X, y, X_val, y_val, learning_rate=1e-3, learning_rate_decay=0.95, reg=5e-6, num_iters=100, batch_size=24, verbose=False): num_train = X.shape[0] iterations_per_epoch = max(num_train / batch_size, 1) # Use SGD to optimize the parameters in self.model loss_history = [] train_acc_history = [] val_acc_history = [] # Training cycle for it in range(num_iters): # Mini-batch selection indexes = np.random.choice(X.shape[0], batch_size, replace=True) X_batch = X[indexes] y_batch = y[indexes] # Compute loss and gradients using the current minibatch loss, grads = self.loss(X_batch, y=y_batch, reg=reg) loss_history.append(loss) # Update weights self.params['W1'] -= learning_rate * grads['W1'] self.params['b1'] -= learning_rate * grads['b1'][0] self.params['W2'] -= learning_rate * grads['W2'] self.params['b2'] -= learning_rate * grads['b2'][0] if verbose and it % 100 == 0: print('iteration %d / %d: loss %f' % (it, num_iters, loss)) # Every epoch, check accuracy and decay learning rate. if it % iterations_per_epoch == 0: # Check accuracy train_acc = (self.predict(X_batch)==y_batch).mean() val_acc = (self.predict(X_val) == y_val).mean() train_acc_history.append(train_acc) val_acc_history.append(val_acc) # Decay learning rate learning_rate *= learning_rate_decay return { 'loss_history': loss_history, 'train_acc_history': train_acc_history, 'val_acc_history': val_acc_history, } def predict(self, X): l1 = X.dot(self.params['W1']) + self.params['b1'] l1[l1 < 0] = 0 l2 = l1.dot(self.params['W2']) + self.params['b2'] exp_scores = np.exp(l2) probs = exp_scores / np.sum(exp_scores, axis=1, keepdims=True) y_pred = np.argmax(probs, axis=1) return y_pred def predict_single(self, X): l1 = X.dot(self.params['W1']) + self.params['b1'] l1[l1 < 0] = 0 l2 = l1.dot(self.params['W2']) + self.params['b2'] exp_scores = np.exp(l2) y_pred = np.argmax(exp_scores) return y_pred
FNNcs231n
:

â , , , .
, N 784, N â (19200 ). , , :
ReLU (Rectified Linear Unit),
. ReLU , , sigmoid tahn; , ( ), learning rate . 784 100, N 100. :
softmax, :
, softmax â . , , . , 100 10, 10 , . Cross-entropy loss softmax :
:
- (Xavier). 2 / . ;
- loss L2 ;
- train -. . , learning rate decay;
- predict_single , predict â ;
:
input_size = 28 * 28 hidden_size = 100 num_classes = 10 net = tln(input_size, hidden_size, num_classes)
:
- ;
- -, ;
- learning rate decay, learning rate ;
- ;
- verbose / ;
stats = net.train(X_train_, y_train_, X_val, y_val, num_iters=19200, batch_size=24, learning_rate=0.1, learning_rate_decay=0.95, reg=0.001, verbose=True)
, loss , :
plt.subplot(2, 1, 1) plt.plot(stats['loss_history']) plt.title('Loss history') plt.xlabel('Iteration') plt.ylabel('Loss') plt.subplot(2, 1, 2) plt.plot(stats['train_acc_history'], label='train') plt.plot(stats['val_acc_history'], label='val') plt.title('Classification accuracy history') plt.xlabel('Epoch') plt.ylabel('Clasification accuracy') plt.legend() plt.show()

â . , , â , . . , , . , , , .
, . . learning rate, regularization, , -; , . .
from itertools import product best_net = None results = {} best_val = -1 learning_rates = [0.001, 0.1, 0.01, 0.5] regularization_strengths = [0.001, 0.1, 0.01] hidden_size = [5, 10, 20] epochs = [1000, 1500, 2000] batch_sizes = [24, 12] best_params = 0 best_params = 0 for v in product(learning_rates, regularization_strengths, hidden_size, epochs, batch_sizes): print('learning rate: {0}, regularization strength: {1}, hidden size: {2}, \ iterations: {3}, batch_size: {4}.'.format(v[0], v[1], v[2], v[3], v[4])) net = TwoLayerNet(input_size, v[2], num_classes) stats = net.train(X_train_, y_train, X_val_, y_val, num_iters=v[3], batch_size=v[4], learning_rate=v[0], learning_rate_decay=0.95, reg=v[1], verbose=False) y_train_pred = net.predict(X_train_) train_acc = np.mean(y_train == y_train_pred) val_acc = (net.predict(X_val_) == y_val).mean() print('Validation accuracy: ', val_acc) results[(v[0], v[1], v[2], v[3], v[4])] = val_acc if val_acc > best_val: best_val = val_acc best_net = net best_params = (v[0], v[1], v[2], v[3], v[4])
, , , - . , , learning rate . . - , : , 1 . 1 24 , - . 24.
MNIST, MNIST.
MNIST def read(path = "."): fname_img = os.path.join(path, 't10k-images.idx3-ubyte') fname_lbl = os.path.join(path, 't10k-labels.idx1-ubyte') with open(fname_lbl, 'rb') as flbl: magic, num = struct.unpack(">II", flbl.read(8)) lbl = np.fromfile(flbl, dtype=np.int8) with open(fname_img, 'rb') as fimg: magic, num, rows, cols = struct.unpack(">IIII", fimg.read(16)) img = np.fromfile(fimg, dtype=np.uint8).reshape(len(lbl), rows, cols) get_img = lambda idx: (lbl[idx], img[idx]) for i in range(len(lbl)): yield get_img(i) test = list(read(path="/MNIST_data")) mnist_labels = np.array([i[0] for i in test]).astype(int) mnist_picts = np.array([i[1] for i in test]).reshape(10000, 784) / 255 y_mnist = np.array(mnist_labels).astype(int)
:

, ~10 , .
, , MNIST . , , MNIST . CNN
Tensorflow MNIST . MNIST 99%+ ( ), : 78.5% 63.35% . , MNIST , MNIST.
, :
. , .
:
net.param
, â , â . numpy :
np.save('tmp/updated_weights.npy', net.params)
.
np.load('models/original_weights.npy')[()]
(
[()]
, ).
.
FNN
. :
- , . ;
- , ;
- (1 , 24);
- (learning rate ) ;
- predict-single 3 . , ;
FNN import numpy as np class FNN(object): """ A two-layer fully-connected neural network. The net has an input dimension of N, a hidden layer dimension of H, and performs classification over C classes. We train the network with a softmax loss function and L2 regularization on the weight matrices. The network uses a ReLU nonlinearity after the first fully connected layer. In other words, the network has the following architecture: input - fully connected layer - ReLU - fully connected layer - softmax The outputs of the second fully-connected layer are the scores for each class. """ def __init__(self, weights,input_size=28*28,hidden_size=100,output_size=10): """ Initialize the model. Weights are initialized following Xavier intialization and biases are initialized to zero. Weights and biases are stored in the variable self.params, which is a dictionary with the following keys: W1: First layer weights; has shape (D, H) b1: First layer biases; has shape (H,) W2: Second layer weights; has shape (H, C) b2: Second layer biases; has shape (C,) Inputs: - input_size: The dimension D of the input data. - hidden_size: The number of neurons H in the hidden layer. - output_size: The number of classes C. """ self.params = {} self.params['W1'] = weights['W1'] self.params['b1'] = weights['b1'] self.params['W2'] = weights['W2'] self.params['b2'] = weights['b2'] def loss(self, X, y, reg=0.0): """ Compute the loss and gradients for a two layer fully connected neural network. Inputs: - X: Input data of shape (N, D). X[i] is a training sample. - y: Vector of training labels. y[i] is the label for X[i], and each y[i] is an integer in the range 0 <= y[i] < C. - reg: Regularization strength. Returns: - loss: Loss (data loss and regularization loss) for this batch of training samples. - grads: Dictionary mapping parameter names to gradients of those parameters with respect to the loss function; has the same keys as self.params. """ # Unpack variables from the params dictionary W1, b1 = self.params['W1'], self.params['b1'] W2, b2 = self.params['W2'], self.params['b2'] N, D = X.shape # Compute the forward pass l1 = X.dot(W1) + b1 l1[l1 < 0] = 0 l2 = l1.dot(W2) + b2 exp_scores = np.exp(l2) probs = exp_scores / np.sum(exp_scores, axis=1, keepdims=True) # Backward pass: compute gradients grads = {} probs[range(X.shape[0]), y] -= 1 dW2 = np.dot(l1.T, probs) dW2 /= X.shape[0] dW2 += reg * W2 grads['W2'] = dW2 grads['b2'] = np.sum(probs, axis=0, keepdims=True) / X.shape[0] delta = probs.dot(W2.T) delta = delta * (l1 > 0) grads['W1'] = np.dot(XT, delta)/ X.shape[0] + reg * W1 grads['b1'] = np.sum(delta, axis=0, keepdims=True) / X.shape[0] return grads def train(self, X, y, learning_rate=0.1*(0.95**24)/32, reg=0.001, batch_size=24): """ Train this neural network using stochastic gradient descent. Inputs: - X: A numpy array of shape (N, D) giving training data. - y: A numpy array f shape (N,) giving training labels; y[i] = c means that [i] has label c, where 0 <= c < C. - X_val: A numpy array of shape (N_val, D); validation data. - y_val: A numpy array of shape (N_val,); validation labels. - learning_rate: Scalar giving learning rate for optimization. - learning_rate_decay: Scalar giving factor used to decay the learning rate each epoch. - reg: Scalar giving regularization strength. - num_iters: Number of steps to take when optimizing. - batch_size: Number of training examples to use per step. - verbose: boolean; if true print progress during optimization. """ num_train = X.shape[0] # Compute loss and gradients using the current minibatch grads = self.loss(X, y, reg=reg) self.params['W1'] -= learning_rate * grads['W1'] self.params['b1'] -= learning_rate * grads['b1'][0] self.params['W2'] -= learning_rate * grads['W2'] self.params['b2'] -= learning_rate * grads['b2'][0] def predict(self, X): """ Use the trained weights of this two-layer network to predict labels for points. For each data point we predict scores for each of the C classes, and assign each data point to the class with the highest score. Inputs: - X: A numpy array of shape (N, D) giving N D-dimensional data points to classify. Returns: - y_pred: A numpy array of shape (N,) giving predicted labels for each of elements of X. For all i, y_pred[i] = c means that X[i] is predicted to have class c, where 0 <= c < C. """ l1 = X.dot(self.params['W1']) + self.params['b1'] l1[l1 < 0] = 0 l2 = l1.dot(self.params['W2']) + self.params['b2'] exp_scores = np.exp(l2) probs = exp_scores / np.sum(exp_scores, axis=1, keepdims=True) y_pred = np.argmax(probs, axis=1) return y_pred def predict_single(self, X): """ Use the trained weights of this two-layer network to predict label for data point. We predict scores for each of the C classes, and assign the data point to the class with the highest score. Inputs: - X: A numpy array of shape (N, D) giving N D-dimensional data points to classify. Returns: - top_3: a list of 3 top most probable predictions with their probabilities as tuples. """ l1 = X.dot(self.params['W1']) + self.params['b1'] l1[l1 < 0] = 0 l2 = l1.dot(self.params['W2']) + self.params['b2'] exp_scores = np.exp(l2) probs = exp_scores / np.sum(exp_scores) y_pred = np.argmax(exp_scores) top_3 = list(zip(np.argsort(probs)[::-1][:3], np.round(probs[np.argsort(probs)[::-1][:3]] * 100, 2))) return top_3
, â , learning rate.
learning rate . 0.1, 24 0.1 x (0.95 ^ 24). , â 800 . , . 0.1 x (0.95 ^ 24) / 32. , .
CNN
, FNN , CNN, , CNN, .
CNN cs231n Tenforlow MNIST, .

import os import numpy as np import matplotlib.pyplot as plt plt.rcdefaults() from matplotlib.lines import Line2D from matplotlib.patches import Rectangle from matplotlib.collections import PatchCollection #https://github.com/gwding/draw_convnet NumConvMax = 8 NumFcMax = 20 White = 1. Light = 0.7 Medium = 0.5 Dark = 0.3 Black = 0. def add_layer(patches, colors, size=24, num=5, top_left=[0, 0], loc_diff=[3, -3], ): # add a rectangle top_left = np.array(top_left) loc_diff = np.array(loc_diff) loc_start = top_left - np.array([0, size]) for ind in range(num): patches.append(Rectangle(loc_start + ind * loc_diff, size, size)) if ind % 2: colors.append(Medium) else: colors.append(Light) def add_mapping(patches, colors, start_ratio, patch_size, ind_bgn, top_left_list, loc_diff_list, num_show_list, size_list): start_loc = top_left_list[ind_bgn] \ + (num_show_list[ind_bgn] - 1) * np.array(loc_diff_list[ind_bgn]) \ + np.array([start_ratio[0] * size_list[ind_bgn], -start_ratio[1] * size_list[ind_bgn]]) end_loc = top_left_list[ind_bgn + 1] \ + (num_show_list[ind_bgn + 1] - 1) \ * np.array(loc_diff_list[ind_bgn + 1]) \ + np.array([(start_ratio[0] + .5 * patch_size / size_list[ind_bgn]) * size_list[ind_bgn + 1], -(start_ratio[1] - .5 * patch_size / size_list[ind_bgn]) * size_list[ind_bgn + 1]]) patches.append(Rectangle(start_loc, patch_size, patch_size)) colors.append(Dark) patches.append(Line2D([start_loc[0], end_loc[0]], [start_loc[1], end_loc[1]])) colors.append(Black) patches.append(Line2D([start_loc[0] + patch_size, end_loc[0]], [start_loc[1], end_loc[1]])) colors.append(Black) patches.append(Line2D([start_loc[0], end_loc[0]], [start_loc[1] + patch_size, end_loc[1]])) colors.append(Black) patches.append(Line2D([start_loc[0] + patch_size, end_loc[0]], [start_loc[1] + patch_size, end_loc[1]])) colors.append(Black) def label(xy, text, xy_off=[0, 4]): plt.text(xy[0] + xy_off[0], xy[1] + xy_off[1], text, family='sans-serif', size=8) if __name__ == '__main__': fc_unit_size = 2 layer_width = 40 patches = [] colors = [] fig, ax = plt.subplots() ############################ # conv layers size_list = [28, 28, 14, 14, 7] num_list = [1, 16, 16, 32, 32] x_diff_list = [0, layer_width, layer_width, layer_width, layer_width] text_list = ['Inputs'] + ['Feature\nmaps'] * (len(size_list) - 1) loc_diff_list = [[3, -3]] * len(size_list) num_show_list = list(map(min, num_list, [NumConvMax] * len(num_list))) top_left_list = np.c_[np.cumsum(x_diff_list), np.zeros(len(x_diff_list))] for ind in range(len(size_list)): add_layer(patches, colors, size=size_list[ind], num=num_show_list[ind], top_left=top_left_list[ind], loc_diff=loc_diff_list[ind]) label(top_left_list[ind], text_list[ind] + '\n{}@{}x{}'.format( num_list[ind], size_list[ind], size_list[ind])) ############################ # in between layers start_ratio_list = [[0.4, 0.5], [0.4, 0.8], [0.4, 0.5], [0.4, 0.8]] patch_size_list = [4, 2, 4, 2] ind_bgn_list = range(len(patch_size_list)) text_list = ['Convolution', 'Max-pooling', 'Convolution', 'Max-pooling'] for ind in range(len(patch_size_list)): add_mapping(patches, colors, start_ratio_list[ind], patch_size_list[ind], ind, top_left_list, loc_diff_list, num_show_list, size_list) label(top_left_list[ind], text_list[ind] + '\n{}x{} kernel'.format( patch_size_list[ind], patch_size_list[ind]), xy_off=[26, -65]) ############################ # fully connected layers size_list = [fc_unit_size, fc_unit_size, fc_unit_size] num_list = [1568, 625, 10] num_show_list = list(map(min, num_list, [NumFcMax] * len(num_list))) x_diff_list = [sum(x_diff_list) + layer_width, layer_width, layer_width] top_left_list = np.c_[np.cumsum(x_diff_list), np.zeros(len(x_diff_list))] loc_diff_list = [[fc_unit_size, -fc_unit_size]] * len(top_left_list) text_list = ['Hidden\nunits'] * (len(size_list) - 1) + ['Outputs'] for ind in range(len(size_list)): add_layer(patches, colors, size=size_list[ind], num=num_show_list[ind], top_left=top_left_list[ind], loc_diff=loc_diff_list[ind]) label(top_left_list[ind], text_list[ind] + '\n{}'.format( num_list[ind])) text_list = ['Flatten\n', 'Fully\nconnected', 'Fully\nconnected'] for ind in range(len(size_list)): label(top_left_list[ind], text_list[ind], xy_off=[-10, -65]) ############################ colors += [0, 1] collection = PatchCollection(patches, cmap=plt.cm.gray) collection.set_array(np.array(colors)) ax.add_collection(collection) plt.tight_layout() plt.axis('equal') plt.axis('off') plt.show() fig.set_size_inches(8, 2.5) fig_dir = './' fig_ext = '.png' fig.savefig(os.path.join(fig_dir, 'convnet_fig' + fig_ext), bbox_inches='tight', pad_inches=0)
Tensorflow, , FNN numpy.
CNN import tensorflow as tf # Define architecture def model(X, w, w3, w4, w_o, p_keep_conv, p_keep_hidden): l1a = tf.nn.relu( tf.nn.conv2d( X, w, strides=[1, 1, 1, 1], padding='SAME' ) + b1 ) l1 = tf.nn.max_pool( l1a, ksize=[1, 2, 2, 1], strides=[1, 2, 2, 1], padding='SAME' ) l1 = tf.nn.dropout(l1, p_keep_conv) l3a = tf.nn.relu( tf.nn.conv2d( l1, w3, strides=[1, 1, 1, 1], padding='SAME' ) + b3 ) l3 = tf.nn.max_pool( l3a, ksize=[1, 2, 2, 1], strides=[1, 2, 2, 1], padding='SAME' ) # Reshaping for dense layer l3 = tf.reshape(l3, [-1, w4.get_shape().as_list()[0]]) l3 = tf.nn.dropout(l3, p_keep_conv) l4 = tf.nn.relu(tf.matmul(l3, w4) + b4) l4 = tf.nn.dropout(l4, p_keep_hidden) pyx = tf.matmul(l4, w_o) + b5 return pyx tf.reset_default_graph() # Define variables init_op = tf.global_variables_initializer() X = tf.placeholder("float", [None, 28, 28, 1]) Y = tf.placeholder("float", [None, 10]) w = tf.get_variable("w", shape=[4, 4, 1, 16], initializer=tf.contrib.layers.xavier_initializer()) b1 = tf.get_variable(name="b1", shape=[16], initializer=tf.zeros_initializer()) w3 = tf.get_variable("w3", shape=[4, 4, 16, 32], initializer=tf.contrib.layers.xavier_initializer()) b3 = tf.get_variable(name="b3", shape=[32], initializer=tf.zeros_initializer()) w4 = tf.get_variable("w4", shape=[32 * 7 * 7, 625], initializer=tf.contrib.layers.xavier_initializer()) b4 = tf.get_variable(name="b4", shape=[625], initializer=tf.zeros_initializer()) w_o = tf.get_variable("w_o", shape=[625, 10], initializer=tf.contrib.layers.xavier_initializer()) b5 = tf.get_variable(name="b5", shape=[10], initializer=tf.zeros_initializer()) # Dropout rate p_keep_conv = tf.placeholder("float") p_keep_hidden = tf.placeholder("float") py_x = model(X, w, w3, w4, w_o, p_keep_conv, p_keep_hidden) reg_losses = tf.get_collection(tf.GraphKeys.REGULARIZATION_LOSSES) reg_constant = 0.01 cost = tf.reduce_mean(tf.nn.softmax_cross_entropy_with_logits(logits=py_x, labels=Y) + reg_constant * sum(reg_losses)) train_op = tf.train.RMSPropOptimizer(0.0001, 0.9).minimize(cost) predict_op = tf.argmax(py_x, 1) #Training train_acc = [] val_acc = [] test_acc = [] train_loss = [] val_loss = [] test_loss = [] with tf.Session() as sess: tf.global_variables_initializer().run() # Training iterations for i in range(256): # Mini-batch training_batch = zip(range(0, len(trX), batch_size), range(batch_size, len(trX)+1, batch_size)) for start, end in training_batch: sess.run(train_op, feed_dict={X: trX[start:end], Y: trY[start:end], p_keep_conv: 0.8, p_keep_hidden: 0.5}) # Comparing labels with predicted values train_acc = np.mean(np.argmax(trY, axis=1) == sess.run(predict_op, feed_dict={X: trX, Y: trY, p_keep_conv: 1.0, p_keep_hidden: 1.0})) train_acc.append(train_acc) val_acc = np.mean(np.argmax(teY, axis=1) == sess.run(predict_op, feed_dict={X: teX, Y: teY, p_keep_conv: 1.0, p_keep_hidden: 1.0})) val_acc.append(val_acc) test_acc = np.mean(np.argmax(mnist.test.labels, axis=1) == sess.run(predict_op, feed_dict={X: mnist_test_images, Y: mnist.test.labels, p_keep_conv: 1.0, p_keep_hidden: 1.0})) test_acc.append(test_acc) print('Step {0}. Train accuracy: {3}. Validation accuracy: {1}. \ Test accuracy: {2}.'.format(i, val_acc, test_acc, train_acc)) _, loss_train = sess.run([predict_op, cost], feed_dict={X: trX, Y: trY, p_keep_conv: 1.0, p_keep_hidden: 1.0}) train_loss.append(loss_train) _, loss_val = sess.run([predict_op, cost], feed_dict={X: teX, Y: teY, p_keep_conv: 1.0, p_keep_hidden: 1.0}) val_loss.append(loss_val) _, loss_test = sess.run([predict_op, cost], feed_dict={X: mnist_test_images, Y: mnist.test.labels, p_keep_conv: 1.0, p_keep_hidden: 1.0}) test_loss.append(loss_test) print('Train loss: {0}. Validation loss: {1}. \ Test loss: {2}.'.format(loss_train, loss_val, loss_test)) # Saving model all_saver = tf.train.Saver() all_saver.save(sess, '/resources/data.chkp') #Predicting with tf.Session() as sess: # Restoring model saver = tf.train.Saver() saver.restore(sess, "./data.chkp") # Prediction pr = sess.run(predict_op, feed_dict={X: mnist_test_images, Y: mnist.test.labels, p_keep_conv: 1.0, p_keep_hidden: 1.0}) print(np.mean(np.argmax(mnist.test.labels, axis=1) == sess.run(predict_op, feed_dict={X: mnist_test_images, Y: mnist.test.labels, p_keep_conv: 1.0, p_keep_hidden: 1.0})))
CNNCNN FNN , . CNN â , - .
. ( N x 28 x 28 x 1), «» ( ), . . . . : . , RGB.
.

, , 1, . zero-padding, 0 , . :
- , , ;
- , ;
- , ;
- , , , zero-padding , ;
max pooling ( , max ). , . : , .
cs231n.
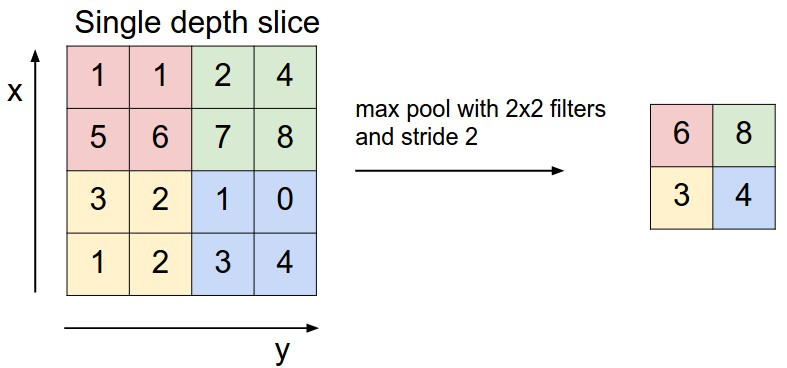
Max pooling ( 2 2) . . ; 2.
. . , , .
, .
N x 28 x 28 x 1. 4 4 16, zero-padding (
padding='SAME'
Tensorflow) , N x 28 x 28 x 16. max pooling 2 2 2, N x 14 x 14 x 16. 4 4 32 zero-padding, N x 14 x 14 x 32. max pooling ( ) N x 7 x 7 x 32. . 32 * 7 * 7 625 1568 625, N 625. 625 10 10 .
, . , «» .
:
- , ;
- : ;
- X, y dropout ;
- Cost softmax_cross_entropy_with_logits L2;
- â RMSPropOptimizer;
- (256), ;
- dropout 0.2 convolutional 0.5 . , «» (MNIST) ;
- , /. , , , ;
MNIST TF :
from tensorflow.examples.tutorials.mnist import input_data mnist = input_data.read_data_sets('MNIST_data', one_hot=True) mnist_test_images = mnist.test.images.reshape(-1, 28, 28, 1)
, FNN, - , :
trX = X_train.reshape(-1, 28, 28, 1)
CNN FNN, -. - , CNN , . , :

:
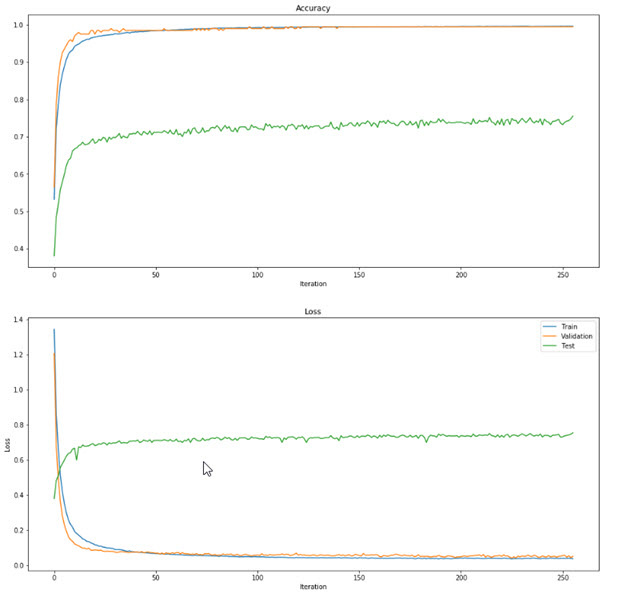
, , ~100 .
CNN , FNN: , 1 , -3 .
CNN import numpy as np import boto import boto3 from boto.s3.key import Key from boto.s3.connection import S3Connection import tensorflow as tf import os class CNN(object): """ Convolutional neural network with several levels. The architecture is the following: input - conv - relu - pool - dropout - conv - relu - pool - dropout - fully connected layer - dropout - fully connected layer The outputs of the second fully-connected layer are the scores for each class. """ def __init__(self): """ Initialize the model. Weights are passed into the class. """ self.params = {} def train(self, image, digit): """ Train this neural network. 1 step of gradient descent. Inputs: - X: A numpy array of shape (N, 784) giving training data. - y: A numpy array f shape (N,) giving training labels; y[i] = c means that X[i] has label c, where 0 <= c < C. """ tf.reset_default_graph() init_op = tf.global_variables_initializer() X = tf.placeholder("float", [None, 28, 28, 1]) Y = tf.placeholder("float", [None, 10]) w = tf.get_variable("w", shape=[4, 4, 1, 16], initializer=tf.contrib.layers.xavier_initializer()) b1 = tf.get_variable(name="b1", shape=[16], initializer=tf.zeros_initializer()) w3 = tf.get_variable("w3", shape=[4, 4, 16, 32], initializer=tf.contrib.layers.xavier_initializer()) b3 = tf.get_variable(name="b3", shape=[32], initializer=tf.zeros_initializer()) w4 = tf.get_variable("w4", shape=[32 * 7 * 7, 625], initializer=tf.contrib.layers.xavier_initializer()) b4 = tf.get_variable(name="b4", shape=[625], initializer=tf.zeros_initializer()) w_o = tf.get_variable("w_o", shape=[625, 10], initializer=tf.contrib.layers.xavier_initializer()) b5 = tf.get_variable(name="b5", shape=[10], initializer=tf.zeros_initializer()) p_keep_conv = tf.placeholder("float") p_keep_hidden = tf.placeholder("float") l1a = tf.nn.relu( tf.nn.conv2d( X, w, strides=[1, 1, 1, 1], padding='SAME' ) + b1 ) l1 = tf.nn.max_pool( l1a, ksize=[1, 2, 2, 1], strides=[1, 2, 2, 1], padding='SAME') l1 = tf.nn.dropout(l1, p_keep_conv) l3a = tf.nn.relu( tf.nn.conv2d( l1, w3, strides=[1, 1, 1, 1], padding='SAME' ) + b3 ) l3 = tf.nn.max_pool( l3a, ksize=[1, 2, 2, 1], strides=[1, 2, 2, 1], padding='SAME') #Reshape for matmul l3 = tf.reshape(l3, [-1, w4.get_shape().as_list()[0]]) l3 = tf.nn.dropout(l3, p_keep_conv) l4 = tf.nn.relu(tf.matmul(l3, w4) + b4) l4 = tf.nn.dropout(l4, p_keep_hidden) py_x = tf.matmul(l4, w_o) + b5 # Cost with L2 reg_losses = tf.get_collection(tf.GraphKeys.REGULARIZATION_LOSSES) reg_constant = 0.01 cost = tf.reduce_mean( tf.nn.softmax_cross_entropy_with_logits(logits=py_x, labels=Y) + reg_constant * sum(reg_losses) ) train_op = tf.train.RMSPropOptimizer(0.00001).minimize(cost) predict_op = tf.argmax(py_x, 1) with tf.Session() as sess: # Load weights saver = tf.train.Saver() saver.restore(sess, "./tmp/data-all_2_updated.chkp") trX = image.reshape(-1, 28, 28, 1) trY = np.eye(10)[digit] sess.run(train_op, feed_dict={X: trX, Y: trY, p_keep_conv: 1, p_keep_hidden: 1}) # Save updated weights all_saver = tf.train.Saver() all_saver.save(sess, './tmp/data-all_2_updated.chkp') def predict(self, image, weights='original'): """ Generate prediction for one or several digits. Weights are downloaded from Amazon. Returns: - top_3: a list of 3 top most probable predictions with their probabilities as tuples. """ s3 = boto3.client( 's3', aws_access_key_id=os.environ['AWS_ACCESS_KEY_ID'], aws_secret_access_key=os.environ['AWS_SECRET_ACCESS_KEY'] ) # Choose weights to load if weights == 'original': f = 'data-all_2.chkp' else: f = 'data-all_2_updated.chkp' fn = f + '.meta' s3.download_file('digit_draw_recognize',fn,os.path.join('tmp/',fn)) fn = f + '.index' s3.download_file('digit_draw_recognize',fn,os.path.join('tmp/',fn)) fn = f + '.data-00000-of-00001' s3.download_file('digit_draw_recognize',fn,os.path.join('tmp/',fn)) tf.reset_default_graph() init_op = tf.global_variables_initializer() sess = tf.Session() # Define model architecture X = tf.placeholder("float", [None, 28, 28, 1]) Y = tf.placeholder("float", [None, 10]) w = tf.get_variable("w", shape=[4, 4, 1, 16], initializer=tf.contrib.layers.xavier_initializer()) b1 = tf.get_variable(name="b1", shape=[16], initializer=tf.zeros_initializer()) w3 = tf.get_variable("w3", shape=[4, 4, 16, 32], initializer=tf.contrib.layers.xavier_initializer()) b3 = tf.get_variable(name="b3", shape=[32], initializer=tf.zeros_initializer()) w4 = tf.get_variable("w4", shape=[32 * 7 * 7, 625], initializer=tf.contrib.layers.xavier_initializer()) w_o = tf.get_variable("w_o", shape=[625, 10], initializer=tf.contrib.layers.xavier_initializer()) b4 = tf.get_variable(name="b4", shape=[625], initializer=tf.zeros_initializer()) p_keep_conv = tf.placeholder("float") p_keep_hidden = tf.placeholder("float") b5 = tf.get_variable(name="b5", shape=[10], initializer=tf.zeros_initializer()) l1a = tf.nn.relu( tf.nn.conv2d( X, w, strides=[1, 1, 1, 1], padding='SAME' ) + b1 ) l1 = tf.nn.max_pool( l1a, ksize=[1, 2, 2, 1], strides=[1, 2, 2, 1], padding='SAME') l1 = tf.nn.dropout(l1, p_keep_conv) l3a = tf.nn.relu( tf.nn.conv2d( l1, w3, strides=[1, 1, 1, 1], padding='SAME' ) + b3 ) l3 = tf.nn.max_pool( l3a, ksize=[1, 2, 2, 1], strides=[1, 2, 2, 1], padding='SAME') l3 = tf.reshape(l3, [-1, w4.get_shape().as_list()[0]]) l3 = tf.nn.dropout(l3, p_keep_conv) l4 = tf.nn.relu(tf.matmul(l3, w4) + b4) l4 = tf.nn.dropout(l4, p_keep_hidden) py_x = tf.matmul(l4, w_o) + b5 # Cost with L2 reg_losses = tf.get_collection(tf.GraphKeys.REGULARIZATION_LOSSES) reg_constant = 0.01 cost = tf.reduce_mean( tf.nn.softmax_cross_entropy_with_logits(logits=py_x, labels=Y) + reg_constant * sum(reg_losses) ) train_op = tf.train.RMSPropOptimizer(0.00001).minimize(cost) predict_op = tf.argmax(py_x, 1) probs = tf.nn.softmax(py_x) # Load model and predict saver = tf.train.Saver() saver.restore(sess, "./tmp/" + f) y_pred = sess.run(probs, feed_dict={X: image.reshape(-1, 28, 28, 1), p_keep_conv: 1.0, p_keep_hidden: 1.0}) probs = y_pred # Create list with top3 predictions and their probabilities top_3 = list(zip(np.argsort(probs)[0][::-1][:3], np.round(probs[0][np.argsort(probs)[0][::-1][:3]] * 100, 2))) sess.close() return top_3
learning rate. 0.00001, 100 . , . , .
( ). -, ,
bootstrap. : html css, js . , , .
. :
main.py ,
functions.py .
functions.py __author__ = 'Artgor' import base64 import os import uuid import random import numpy as np import boto import boto3 from boto.s3.key import Key from boto.s3.connection import S3Connection from codecs import open from PIL import Image from scipy.ndimage.interpolation import rotate, shift from skimage import transform from two_layer_net import FNN from conv_net import CNN class Model(object): def __init__(self): """ Load weights for FNN here. Original weights are loaded from local folder, updated - from Amazon. """ self.params_original = np.load('models/original_weights.npy')[()] self.params = self.load_weights_amazon('updated_weights.npy') def process_image(self, image): """ Processing image for prediction. Saving in temproral folder so that it could be opened by PIL. Cropping and scaling so that the longest side it 20. Then putting it in a center of 28x28 blank image. Returning array of normalized data. """ filename = 'digit' + '__' + str(uuid.uuid1()) + '.jpg' with open('tmp/' + filename, 'wb') as f: f.write(image) img = Image.open('tmp/' + filename) bbox = Image.eval(img, lambda px: 255-px).getbbox() if bbox == None: return None widthlen = bbox[2] - bbox[0] heightlen = bbox[3] - bbox[1] if heightlen > widthlen: widthlen = int(20.0 * widthlen/heightlen) heightlen = 20 else: heightlen = int(20.0 * widthlen/heightlen) widthlen = 20 hstart = int((28 - heightlen) / 2) wstart = int((28 - widthlen) / 2) img_temp = img.crop(bbox).resize((widthlen, heightlen), Image.NEAREST) new_img = Image.new('L', (28,28), 255) new_img.paste(img_temp, (wstart, hstart)) imgdata = list(new_img.getdata()) img_array = np.array([(255.0 - x) / 255.0 for x in imgdata]) return img_array def augment(self, image, label): """ Augmenting image for training. Saving in temporal folder so that it could be opened by PIL. Cropping and scaling so that the longest side it 20. The width and height of the scaled image are used to resize it again so that there would be 4 images with different combinations of weight and height. Then putting it in a center of 28x28 blank image. 12 possible angles are defined and each image is randomly rotated by 6 of these angles. Images converted to arrays and normalized. Returning these arrays and labels. """ filename = 'digit' + '__' + str(uuid.uuid1()) + '.jpg' with open('tmp/' + filename, 'wb') as f: f.write(image) image = Image.open('tmp/' + filename) ims_add = [] labs_add = [] angles = np.arange(-30, 30, 5) bbox = Image.eval(image, lambda px: 255-px).getbbox() widthlen = bbox[2] - bbox[0] heightlen = bbox[3] - bbox[1] if heightlen > widthlen: widthlen = int(20.0 * widthlen/heightlen) heightlen = 20 else: heightlen = int(20.0 * widthlen/heightlen) widthlen = 20 hstart = int((28 - heightlen) / 2) wstart = int((28 - widthlen) / 2) for i in [min(widthlen, heightlen), max(widthlen, heightlen)]: for j in [min(widthlen, heightlen), max(widthlen, heightlen)]: resized_img = image.crop(bbox).resize((i, j), Image.NEAREST) resized_image = Image.new('L', (28,28), 255) resized_image.paste(resized_img, (wstart, hstart)) angles_ = random.sample(set(angles), 6) for angle in angles_: transformed_image = transform.rotate(np.array(resized_image), angle, cval=255, preserve_range=True).astype(np.uint8) labs_add.append(int(label)) img_temp = Image.fromarray(np.uint8(transformed_image)) imgdata = list(img_temp.getdata()) normalized_img = [(255.0 - x) / 255.0 for x in imgdata] ims_add.append(normalized_img) image_array = np.array(ims_add) label_array = np.array(labs_add) return image_array, label_array def load_weights_amazon(self, filename): """ Load weights from Amazon. This is npy. file, which needs to be read with np.load. """ s3 = boto3.client( 's3', aws_access_key_id=os.environ['AWS_ACCESS_KEY_ID'], aws_secret_access_key=os.environ['AWS_SECRET_ACCESS_KEY'] ) s3.download_file('digit_draw_recognize', filename, os.path.join('tmp/', filename)) return np.load(os.path.join('tmp/', filename))[()] def save_weights_amazon(self, filename, file): """ Save weights to Amazon. """ REGION_HOST = 's3-external-1.amazonaws.com' conn = S3Connection(os.environ['AWS_ACCESS_KEY_ID'], os.environ['AWS_SECRET_ACCESS_KEY'], host=REGION_HOST) bucket = conn.get_bucket('digit_draw_recognize') k = Key(bucket) k.key = filename k.set_contents_from_filename('tmp/' + filename) return ('Weights saved') def save_image(self, drawn_digit, image): """ Save image on Amazon. Only existing files can be uploaded, so the image is saved in a temporary folder. """ filename = 'digit' + str(drawn_digit) + '__' + \ str(uuid.uuid1()) + '.jpg' with open('tmp/' + filename, 'wb') as f: f.write(image) REGION_HOST = 's3-external-1.amazonaws.com' conn = S3Connection(os.environ['AWS_ACCESS_KEY_ID'], os.environ['AWS_SECRET_ACCESS_KEY'], host=REGION_HOST) bucket = conn.get_bucket('digit_draw_recognize') k = Key(bucket) k.key = filename k.set_contents_from_filename('tmp/' + filename) return ('Image saved successfully with the \ name {0}'.format(filename)) def predict(self, image): """ Predicting image. If nothing is drawn, returns a message; otherwise 4 models are initialized and they make predictions. They return a list of tuples with 3 top predictions and their probabilities. These lists are sent to "select_answer" method to select the best answer. Also tuples are converted into strings for easier processing in JS. The answer and lists with predictions and probabilities are returned. """ img_array = self.process_image(image) if img_array is None: return "Can't predict, when nothing is drawn" net = FNN(self.params) net_original = FNN(self.params_original) cnn = CNN() cnn_original = CNN() top_3 = net.predict_single(img_array) top_3_original = net_original.predict_single(img_array) top_3_cnn = cnn.predict(img_array, weights='updated') top_3_cnn_original = cnn_original.predict(img_array, weights='original') answer, top_3, top_3_original, top_3_cnn, top_3_cnn_original = self.select_answer(top_3, top_3_original, top_3_cnn, top_3_cnn_original) answers_dict = {'answer': str(answer), 'fnn_t': top_3, 'fnn': top_3_original, 'cnn_t': top_3_cnn, 'cnn': top_3_cnn_original} return answers_dict def train(self, image, digit): """ Models are trained. Weights on Amazon are updated. """ r = self.save_image(digit, image) print(r)
«» , . FNN â ,
init
, .
, tmp ( , ). , Amazon. ,
load_weights_amazonAmazon : , ( , ,
os.environ['AWS_ACCESS_KEY_ID']
, , :
aws_access_key_id=os.environ['AWS_ACCESS_KEY_ID']
). bucket , .
Tensorflow , . , Tensorflow .
JS Python
predict
. , â , . , , . JS, , .
4 . FNN , .
CNN , â . .
4 . ?
select_answer
. FNN CNN: , ; 50%, , ; , , , ( ). , ( ) , JS. JS.
JS , â .
:
- ( ) Python;
- ( ) , ;
- train, 1 ;
- ;
. :
- canvas â , ;
- «Predict» â , , ;
- «Allow to use the drawn digit for model training» â , . / ;
- «Yes» â , ( );
- «No» â , ( );
- «Clear» â canvas ;
- / ;
JS / :
document.getElementById().style.display
«none» «block».
, , , .
- . , , - . bootstrap , . , . mozilla . , : ontouchstart, ontouchmove, ontouchend. , , ;
- - . MNIST , , . ⊠, Heroku , , ;
- : , . : CNN . ⊠, . , , , . .
- , : , . , . :
Models
. Flask. python , ;
ãããžã§ã¯ãã®éå§
23 « » . . , , ( ). , , , , « ». .
â . 2 : , ; mozilla . , , , .
â , / ( , , ), , «» .
: . , , 2,5 ( , ). ⊠:

.
~ , ( slack ODS) . , , .
- «-». , , . â - google quickdraw , . â SVM . , , â ( ), ;
- . : -;
- â ;
- / ;
- / . , «Yes» «No»;
- PC â , ;
ãããã«
. . . . . , , data science , .